MobileNet keras
时间: 2024-06-15 08:02:15 浏览: 17
MobileNet 是一种轻量级的深度学习模型,专为移动设备和嵌入式系统设计,以减少计算资源和内存占用,同时保持较高的性能。它是 Google 在 2017 年 ICLR 大会上提出的,由 Inception 模型发展而来,但采用了深度可分离卷积(Depthwise Separable Convolution)来大幅度减少参数数量。
在 Keras 中,你可以使用 `tf.keras.applications.MobileNet` 或 `keras.applications.mobilenet_v2.MobileNetV2` 来导入预训练的 MobileNet 模型。这个模型通常包括以下几个部分:
1. **输入层**:接受图像数据作为输入。
2. **卷积层**:包括深度可分离卷积层,它们分别对空间维度和通道维度进行操作,大大减少了参数数量。
3. **瓶颈层**:使用扩张路径(Expanded Path),包含一个深度可分离卷积后接一个1x1卷积来增加通道数。
4. **全局平均池化**(Global Average Pooling):代替全连接层,减少过拟合并使网络更易于部署。
5. **分类层**:如 `tf.keras.layers.Dense`,用于输出分类结果。
如果你想要在 Keras 中使用 MobileNet,可以直接加载预训练权重,然后可以选择冻结部分层进行微调,或者从头开始训练。以下是使用 Keras 导入 MobileNet 的基本步骤:
```python
from tensorflow.keras.applications import MobileNet
from tensorflow.keras.models import Model
from tensorflow.keras.layers import Dense, GlobalAveragePooling2D
# 加载预训练模型
base_model = MobileNet(weights='imagenet', include_top=False, input_shape=(img_height, img_width, 3))
# 添加全局平均池化和全连接层进行分类任务
x = base_model.output
x = GlobalAveragePooling2D()(x)
predictions = Dense(num_classes, activation='softmax')(x)
# 创建新的模型
model = Model(inputs=base_model.input, outputs=predictions)
# 选择是否训练或冻结预训练层
if fine_tuning:
# 冻结所有层
for layer in base_model.layers:
layer.trainable = False
# 再定义几个顶部的层进行微调
num_frozen_layers = len(base_model.layers) - num_top_layers_to_freeze
for layer in model.layers[:num_frozen_layers]:
layer.trainable = False
else:
# 训练整个模型
model.trainable = True
```
相关推荐
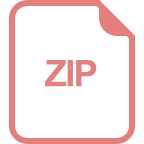
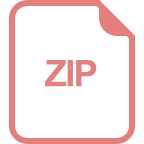
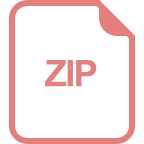














