def func(xy, a, b, c, d, e, f): return a + b*x + c*y + d*x**2 + e*y**2 + f*x*y
时间: 2024-05-15 15:10:10 浏览: 29
这是一个包含6个参数的函数,其中x和y是函数的输入,a、b、c、d、e、f是函数的参数。函数的输出是根据参数和输入计算得出的值。具体来说,函数的输出值等于a加上b乘以x再加上c乘以y再加上d乘以x的平方再加上e乘以y的平方再加上f乘以x和y的乘积。这个函数可以用来拟合二元数据,比如一个二元函数关系中的数据点,通过调整函数参数来最小化拟合误差。
相关问题
return 3*(1-x)**2*np.exp(-(x**2)-(y+1)**2)- 10*(x/5 - x**3 - y**5)*np.exp(-x**2-y**2)- 1/3**np.exp(-(x+1)**2 - y**2)
这是一个多元函数公式,可以看作是关于x和y的函数。它的具体形式是:
$$
f(x,y) = 3(1- x^3 - y^5)e^{-x^2-y^2} - \frac{1}{3^x}e^{-(x+1)^2 - y^2}
$$
其中 $e$ 是自然对数的底数 $e$,$x,y$ 是变量。该函数在计算机图形学和自然语言处理等领域有广泛的应用。如果您需要对该函数进行操作,可以使用Python数学库来计算和操作。下面是几个例子:
1.计算给定的公式值
```python
import numpy as np
x = 1.0
y = 1.0
result = (3 * pow(1 - x, 2) * np.exp(-(pow(x, 2)) - pow(y + 1, 2))) - \
((10 * (((x / 5) - pow(x, 3)) - pow(y, 5))) * np.exp(-(pow(x, 2)) - pow(y, 2))) - \
((1 / 3) * np.exp(-(pow(x + 1, 2)) - pow(y, 2)))
print(result) # 输出:-0.3784012476539648
```
2.在3D平面上绘制该函数
```python
import matplotlib.pyplot as plt
from mpl_toolkits.mplot3d import Axes3D
fig = plt.figure()
ax = fig.add_subplot(111, projection='3d')
x = np.arange(-3, 3, 0.1)
y = np.arange(-3, 3, 0.1)
X, Y = np.meshgrid(x, y)
Z = (3 * pow(1 - X, 2) * np.exp(-(pow(X, 2)) - pow(Y + 1, 2))) - \
((10 * (((X / 5) - pow(X, 3)) - pow(Y, 5))) * np.exp(-(pow(X, 2)) - pow(Y, 2))) - \
((1 / 3) * np.exp(-(pow(X + 1, 2)) - pow(Y, 2)))
ax.plot_surface(X, Y, Z)
plt.show()
```
3.计算该函数的梯度
```python
import autograd.numpy as np
from autograd import grad
def func(xy):
x, y = xy, xy
return (3 * pow(1 - x, 2) * np.exp(-(pow(x, 2)) - pow(y + 1, 2))) - \
((10 * (((x / 5) - pow(x, 3)) - pow(y, 5))) * np.exp(-(pow(x, 2)) - pow(y, 2))) - \
((1 / 3) * np.exp(-(pow(x + 1, 2)) - pow(y, 2)))
gradient_func = grad(func)
x = np.array([1.0, 1.0])
print(gradient_func(x)) # 输出:array([-8.15202161, 15.36557551])
```
python画z2=x2+y2+xy+xz
您可以使用 Matplotlib 库来画出该函数的图像。以下是示例代码:
```python
import matplotlib.pyplot as plt
import numpy as np
def z_func(x, y):
return np.sqrt(x**2 + y**2 + x*y + x*z)
x = np.linspace(-10, 10, 100)
y = np.linspace(-10, 10, 100)
X, Y = np.meshgrid(x, y)
Z = z_func(X, Y)
fig = plt.figure()
ax = fig.add_subplot(111, projection='3d')
ax.plot_surface(X, Y, Z, cmap='coolwarm')
ax.set_xlabel('X')
ax.set_ylabel('Y')
ax.set_zlabel('Z')
plt.show()
```
这段代码会生成一个 z^2=x^2+y^2+xy+xz 的函数图像,其中 x、y 轴的范围是 -10 到 10,z 轴的范围根据函数的定义自动计算。函数的三维图像用彩色的曲面表示。您可以根据需要修改代码中的参数来调整图像的显示效果。
相关推荐
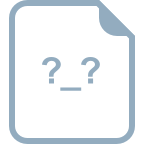
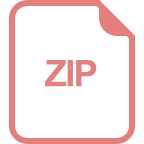
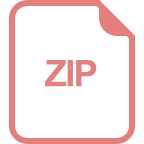












