import torch.optim
时间: 2024-01-31 18:03:18 浏览: 111
`torch.optim`是PyTorch中用于优化算法的模块。它提供了各种优化器,如随机梯度下降(SGD)、Adam、Adagrad等,用于更新神经网络的参数以最小化损失函数。通过使用`torch.optim`模块,我们可以方便地在训练过程中调整学习率、设置动量、设置权重衰减等。
以下是一个使用`torch.optim`模块的例子:
```python
import torch
import torch.nn as nn
import torch.optim as optim
# 定义模型
model = nn.Linear(10, 2)
# 定义优化器
optimizer = optim.SGD(model.parameters(), lr=0.01, momentum=0.9, weight_decay=1e-5)
# 在训练过程中使用优化器更新模型参数
for epoch in range(100):
# 前向传播和计算损失
loss = ...
# 梯度清零
optimizer.zero_grad()
# 反向传播
loss.backward()
# 更新模型参数
optimizer.step()
```
在上面的例子中,我们使用了`optim.SGD`优化器,它使用随机梯度下降算法来更新模型参数。`lr`参数表示学习率,`momentum`参数表示动量,`weight_decay`参数表示权重衰减。
相关问题
import torch.optim as optim
from typing import List,Tuple
import torch
import torch.nn as nn
import torch.nn.functional as F
class Net(nn.Module):
def __init__(self, input_size:int, hidden_size:List[int], output_size:int, dropout:float):
super(Net, self).__init__()
self.input_size = input_size
self.hidden_size = hidden_size
self.output_size = output_size
self.dropout = dropout
# Construct the hidden layers
self.hidden_layers = nn.ModuleList()
for i in range(len(hidden_size)):
if i == 0:
self.hidden_layers.append(nn.Linear(input_size, hidden_size[i]))
else:
self.hidden_layers.append(nn.Linear(hidden_size[i-1], hidden_size[i]))
# Construct the output layer
self.output_layer = nn.Linear(hidden_size[-1], output_size)
# Set up the dropout layer
self.dropout_layer = nn.Dropout(p=dropout)
def forward(self, x:torch.Tensor) -> torch.Tensor:
# Pass the input through the hidden layers
for layer in self.hidden_layers:
x = F.relu(layer(x))
x = self.dropout_layer(x)
# Pass the output from the last hidden layer through the output layer
x = self.output_layer(x)
return x
def train_model(model:Net, train_data:Tuple[torch.Tensor, torch.Tensor],
test_data:Tuple[torch.Tensor, torch.Tensor],
batch_size:int, num_epochs:int, learning_rate:float):
# Extract the inputs and labels from the training data
train_inputs, train_labels = train_data
# Define the loss function and optimizer
criterion = nn.CrossEntropyLoss()
optimizer = optim.Adam(model.parameters(), lr=learning_rate)
# Loop over the training data for the specified number of epochs
for epoch in range(num_epochs):
# Shuffle the training data
perm = torch.randperm(train_inputs.size(0))
train_inputs = train_inputs[perm]
train_labels = train_labels[perm]
# Loop over the training data in batches
for i in range(0, train_inputs.size(0), batch_size):
# Extract the current batch of data
inputs = train_inputs[i:i+batch_size]
labels = train_labels[i:i+batch_size]
# Zero the gradients
optimizer.zero_grad()
# Forward pass
outputs = model(inputs)
loss = criterion(outputs, labels)
# Backward pass and update parameters
loss.backward()
optimizer.step()
# Evaluate the model on the test data
test_inputs, test_labels = test_data
test_outputs = model(test_inputs)
test_loss = criterion(test_outputs, test_labels)
test_accuracy = accuracy(test_outputs, test_labels)
# Print the epoch number, training loss, and test accuracy
print(f"Epoch {epoch+1}/{num_epochs}: Train loss={loss:.4f}, Test loss={test_loss:.4f}, Test accuracy={test_accuracy:.4f}")
def accuracy(outputs:torch.Tensor, labels:torch.Tensor) -> float:
predictions = torch.argmax(outputs, dim=1)
correct_predictions = torch.sum(predictions == labels)
accuracy = correct_predictions.float() / labels.size(0)
return accuracy.item()
import torch.nn as nn import torch.nn.functional as F import torch.optim as optim
As an AI language model, I don't have a context to decide what task you want to achieve by importing these modules. So, I am providing you some information on these modules.
These modules are PyTorch built-in modules for building and training deep learning models.
torch.nn module provides various classes and functions for building neural networks. For example, it provides classes for defining layers such as Linear, Conv2d, LSTM, etc. It also provides classes for defining activation functions, loss functions, and more.
torch.nn.functional module provides various functions that can be used inside the neural network layers. For example, it provides functions for activation functions like ReLU, sigmoid, etc., pooling functions like max pooling, convolution functions, and more.
torch.optim module provides various optimization algorithms for training deep learning models. For example, it provides classes for optimization algorithms like Stochastic Gradient Descent (SGD), Adam, Adagrad, etc.
In summary, these modules are essential for building and training deep learning models using PyTorch.
阅读全文
相关推荐
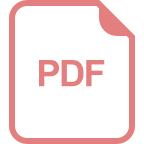
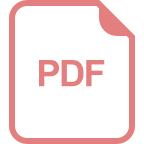
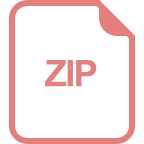














