def preprocess_data(data): # 将音符和和弦分开 notes, chords = [], [] for seq in data: notes.append(seq[:, :NUM_CLASSES]) chords.append(seq[:, NUM_CLASSES:]) notes = np.array(notes) chords = np.array(chords) # 对音符序列进行one-hot编码 notes = to_categorical(notes, num_classes=NUM_CLASSES) # 对和弦序列进行编码 chords = np.argmax(chords, axis=-1) # 对音符和和弦序列进行填充 notes = pad_sequences(notes, maxlen=SEQ_LEN, padding='pre', truncating='pre') chords = pad_sequences(chords, maxlen=SEQ_LEN, padding='pre', truncating='pre') return notes, chords
时间: 2024-04-28 18:24:09 浏览: 116
这是一个用于预处理音符和和弦数据的函数,其中有几个步骤:
1. 将原始数据中的音符和和弦分开存储。
2. 对音符序列进行 one-hot 编码,使其可以被神经网络直接处理。
3. 对和弦序列进行编码,将其转换为整数表示。
4. 对音符和和弦序列进行填充,使它们的长度一致,便于神经网络进行处理。
函数的输入是原始数据,输出是经过预处理后的音符和和弦序列。其中,NUM_CLASSES 表示音符或和弦的数量,SEQ_LEN 表示序列的长度。
相关问题
def preprocess_data(note, default_value=1): note_to_int = dict((notes, chords) for notes, chords in enumerate(note)) note_to_number = {'C4': 60, 'D4': 62, 'E4': 64, 'F4': 65, 'G4': 67, 'A4': 69, 'B4': 71} # 将音符和和弦分开 notes, chords = [], [] notes.append([note_to_int.get(char, default_value) for char in note]) chords.append([note_to_int.get(char, default_value) for char in note]) # 对音符序列进行one-hot编码 notes = to_categorical(notes, num_classes=NUM_CLASSES) for seq in note: seq = 'C4' if seq.isnumeric(): seq = int(seq) else: # 处理无效的序列字符串 pass seq = note_to_number[seq] seq = int(seq) notes.append(seq[:, :NUM_CLASSES]) chords.append(seq[:, NUM_CLASSES:]) # 对和弦序列进行编码 chords = np.argmax(chords, axis=-1) notes = np.array(notes) chords = np.array(chords) # 对音符和和弦序列进行填充 notes = pad_sequences(notes, maxlen=SEQ_LEN, padding='pre', truncating='pre') chords = pad_sequences(chords, maxlen=SEQ_LEN, padding='pre', truncating='pre') return notes, chords
这段代码是一个音乐数据预处理函数,它将音符和和弦分开,对音符进行one-hot编码,对和弦进行编码,并对音符和和弦进行填充。其中,参数note是一个音符序列,default_value是一个默认值,note_to_int和note_to_number是两个字典,用于将音符和数字进行映射。该函数的返回值为notes和chords,它们分别是经过处理后的音符和和弦序列。
将下列代码补全:# Data Loading and Preprocessing def load_and_preprocess_data(): # 加载雷达和ECG数据 # 数据归一化和预处理 pass
要完成这个函数,你需要首先导入必要的库,然后加载雷达和ECG数据。如果数据存储在文件中,可以使用pandas读取CSV文件;如果是从网络获取,可以用像`requests`这样的库。接着,数据预处理通常是将数值型特征缩放到0到1之间,或者根据需求标准化。对于分类特征,你可能需要转换成独热编码。这里是一个示例代码:
```python
import pandas as pd
from sklearn.preprocessing import MinMaxScaler
def load_and_preprocess_data():
# 加载雷达和ECG数据
if data_path.endswith('.csv'):
radar_data = pd.read_csv('radar_data.csv')
ecg_data = pd.read_csv('ecg_data.csv')
else:
# 如果数据是从网络获取,替换为适当的URL或API请求
radar_data = requests.get('radar_data_url').content
ecg_data = requests.get('ecg_data_url').content
# 数据归一化
scaler = MinMaxScaler()
radar_data_normalized = scaler.fit_transform(radar_data)
ecg_data_normalized = scaler.transform(ecg_data)
# 结合两个数据集
combined_data = pd.concat([pd.DataFrame(radar_data_normalized), pd.DataFrame(ecg_data_normalized)], axis=1)
# 返回预处理后的数据
return combined_data
```
在这个例子中,我们假设数据已经按照每列对应的方式分开存储,如果没有,则可能需要进一步拆分或合并数据。
阅读全文
相关推荐
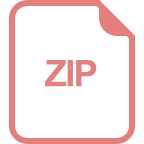














