def preprocess_data(self): if not self.data.empty: # 忽略warning warnings.filterwarnings("ignore", category=UserWarning) # 复制数据集 processed_data = self.data.copy() # 处理字符串列 string_columns = processed_data.select_dtypes(include=['object']).columns for column in string_columns: processed_data[column] = processed_data[column].str.split(';').apply(lambda x: [float(val) for val in x]) # 处理数值列 numeric_columns = processed_data.select_dtypes(include=['float', 'int']).columns imputer = SimpleImputer(strategy='mean') processed_data[numeric_columns] = imputer.fit_transform(processed_data[numeric_columns]) # 处理异常值 isolation_forest = IsolationForest(contamination=0.05) outliers = isolation_forest.fit_predict(processed_data[numeric_columns]) processed_data = processed_data[outliers != -1] # 标准化处理 scaler = StandardScaler() processed_data[numeric_columns] = scaler.fit_transform(processed_data[numeric_columns]) # 创建一个新窗口来显示处理后的数据集 top = tk.Toplevel(self.master) top.title("处理后的数据集") # 创建用于显示处理后的数据集的表格 table = tk.Text(top) table.pack() # 将处理后的数据集转换为字符串并显示在表格中 table.insert(tk.END, str(processed_data)) table.config(state=tk.DISABLED) else: self.path_label.config(text="请先导入数据集")
时间: 2024-02-14 12:23:53 浏览: 127
这段代码是用于数据预处理的,主要包括以下步骤:
1. 处理字符串列:将字符串列按分号拆分为多个数值,并将每个数值转换为 float 类型。
2. 处理数值列:使用均值填充缺失值。
3. 处理异常值:使用孤立森林算法检测和过滤异常值。
4. 标准化处理:使用 StandardScaler 类对数值列进行标准化处理。
5. 在新窗口中显示处理后的数据集:创建一个新窗口,并在其中使用 Text 控件显示处理后的数据集。
需要注意的是,这段代码使用了一些第三方库,如 scikit-learn 和 tkinter。
相关问题
修改以下代码使其能够输出模型预测结果: def open_image(self): file_dialog = QFileDialog() file_paths, _ = file_dialog.getOpenFileNames(self, "选择图片", "", "Image Files (*.png *.jpg *.jpeg)") if file_paths: self.display_images(file_paths) def preprocess_images(self, image_paths): data_transform = transforms.Compose([ transforms.CenterCrop(150), transforms.ToTensor(), transforms.Normalize([0.485, 0.456, 0.406], [0.229, 0.224, 0.225]) ]) self.current_image_paths = [] images = [] for image_path in image_paths: image = Image.open(image_path) image = data_transform(image) image = torch.unsqueeze(image, dim=0) images.append(image) self.current_image_paths.append(image_path) return images def predict_images(self): if not self.current_image_paths: return for i, image_path in enumerate(self.current_image_paths): image = self.preprocess_image(image_path) output = self.model(image) predicted_class = self.class_dict[output.argmax().item()] self.result_labels[i].setText(f"Predicted Class: {predicted_class}") self.progress_bar.setValue((i+1)*20) def display_images(self, image_paths): for i, image_path in enumerate(image_paths): image = QImage(image_path) image = image.scaled(300, 300, Qt.KeepAspectRatio) if i == 0: self.image_label_1.setPixmap(QPixmap.fromImage(image)) elif i == 1: self.image_label_2.setPixmap(QPixmap.fromImage(image)) elif i == 2: self.image_label_3.setPixmap(QPixmap.fromImage(image)) elif i == 3: self.image_label_4.setPixmap(QPixmap.fromImage(image)) elif i == 4: self.image_label_5.setPixmap(QPixmap.fromImage(image))
请注意,代码中的 preprocess_images 函数和 predict_images 函数中的 self.preprocess_image(image_path) 需要修改为 self.preprocess_images([image_path])。修改后的代码如下:
```
def open_image(self):
file_dialog = QFileDialog()
file_paths, _ = file_dialog.getOpenFileNames(self, "选择图片", "", "Image Files (*.png *.jpg *.jpeg)")
if file_paths:
self.display_images(file_paths)
def preprocess_images(self, image_paths):
data_transform = transforms.Compose([
transforms.CenterCrop(150),
transforms.ToTensor(),
transforms.Normalize([0.485, 0.456, 0.406], [0.229, 0.224, 0.225])
])
self.current_image_paths = []
images = []
for image_path in image_paths:
image = Image.open(image_path)
image = data_transform(image)
image = torch.unsqueeze(image, dim=0)
images.append(image)
self.current_image_paths.append(image_path)
return images
def predict_images(self):
if not self.current_image_paths:
return
for i, image_path in enumerate(self.current_image_paths):
image = self.preprocess_images([image_path])
output = self.model(image[0])
predicted_class = self.class_dict[output.argmax().item()]
self.result_labels[i].setText(f"Predicted Class: {predicted_class}")
self.progress_bar.setValue((i+1)*20)
def display_images(self, image_paths):
for i, image_path in enumerate(image_paths):
image = QImage(image_path)
image = image.scaled(300, 300, Qt.KeepAspectRatio)
if i == 0:
self.image_label_1.setPixmap(QPixmap.fromImage(image))
elif i == 1:
self.image_label_2.setPixmap(QPixmap.fromImage(image))
elif i == 2:
self.image_label_3.setPixmap(QPixmap.fromImage(image))
elif i == 3:
self.image_label_4.setPixmap(QPixmap.fromImage(image))
elif i == 4:
self.image_label_5.setPixmap(QPixmap.fromImage(image))
```
def preprocess_text(d://pythonpath//zy.txt):有什么错误
这段代码中的路径应该使用反斜杠(\)而不是正斜杠(/),并且字符串应该被引号包含起来。正确的写法应该是:
```python
def preprocess_text("d:\\pythonpath\\zy.txt"):
# 处理文本的代码
pass
```
或者使用原始字符串:
```python
def preprocess_text(r"d:\pythonpath\zy.txt"):
# 处理文本的代码
pass
```
阅读全文
相关推荐
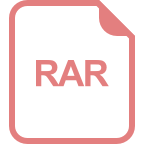
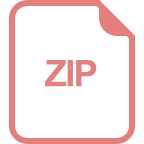













