mixed_data = lam * data[0] + (1 - lam) * data[indices] TypeError: only integer tensors of a single element can be converted to an index
时间: 2024-05-05 09:22:01 浏览: 5
这个错误通常是因为 `indices` 不是整数类型的张量而引起的。在执行像 `data[indices]` 这样的操作时,`indices` 应该是一个整数张量,表示要从 `data` 张量中选择的元素的索引。如果 `indices` 不是整数类型的张量,则会出现上述错误。
你可以通过将 `indices` 转换为整数类型的张量来解决这个问题,例如:
```
indices = tf.cast(indices, tf.int32)
mixed_data = lam * data[0] + (1 - lam) * data[indices]
```
这将使用 TensorFlow 的 `tf.cast` 函数将 `indices` 转换为整数类型的张量。
相关问题
pre_lam_1 = lam_1 + dt * 0.0是什么意思
这段代码的意思是将变量 pre_lam_1 赋值为变量 lam_1 乘以 dt 和 0.0 的积。其中 dt 是一个时间间隔,0.0 是一个浮点数常量。具体的计算结果取决于 lam_1 的值和 dt 的值。
迭代法求解方程 def func(x): f1 = (n00 * math.log((1-lam11)**2 + dta1**2 * lam11**2 * (R1-1))) - 2 * (n00+n10+n20) * math.log(1+(dta1-1)*lam11)+n01 * math.log(1+R1*lam11**2-2*lam11) + n10 * math.log(1-lam11+dta1*lam11*(1-R1)) + n11*math.log(1-R1*lam11)+(n10+n20)*math.log(dta1)+(n10+n11+2*n20+2*n21)*math.log(lam11) + (n20+n21)*math.log(R1) f2 = (n00 * math.log((1-lam10)**2 + x**2 * lam10**2 * (R0-1))) - 2 * (n00+n10+n20) * math.log(1+(x-1)*lam10) +n01 * math.log(1+R0*lam10**2-2*lam10) + n10 * math.log(1-lam10+x*lam10*(1-R0)) + n11*math.log(1-R0*lam10)+(n10+n20)*math.log(x)+(n10+n11+2*n20+2*n21)*math.log(lam10) + (n20+n21)*math.log(R0) return 2 * (f1-f2) - Z.ppf(0.95)的所有根的代码
以下是使用迭代法求解方程的代码:
```python
import math
from scipy.stats import norm
def func(x):
# 填入方程
f1 = (n00 * math.log((1-lam11)**2 + dta1**2 * lam11**2 * (R1-1))) - 2 * (n00+n10+n20) * math.log(1+(dta1-1)*lam11)+n01 * math.log(1+R1*lam11**2-2*lam11) + n10 * math.log(1-lam11+dta1*lam11*(1-R1)) + n11*math.log(1-R1*lam11)+(n10+n20)*math.log(dta1)+(n10+n11+2*n20+2*n21)*math.log(lam11) + (n20+n21)*math.log(R1)
f2 = (n00 * math.log((1-lam10)**2 + x**2 * lam10**2 * (R0-1))) - 2 * (n00+n10+n20) * math.log(1+(x-1)*lam10) +n01 * math.log(1+R0*lam10**2-2*lam10) + n10 * math.log(1-lam10+x*lam10*(1-R0)) + n11*math.log(1-R0*lam10)+(n10+n20)*math.log(x)+(n10+n11+2*n20+2*n21)*math.log(lam10) + (n20+n21)*math.log(R0)
return 2 * (f1-f2) - norm.ppf(0.95)
# 定义参数
n00 = 100
n01 = 50
n10 = 30
n11 = 20
n20 = 20
n21 = 10
lam10 = 0.6
lam11 = 0.7
dta1 = 0.2
R0 = 0.8
R1 = 0.9
# 设置初始值
x0 = 0.5
# 迭代求根
x = x0
for i in range(100):
x_next = x - func(x) / (func(x+0.00001) - func(x))
if abs(x_next - x) < 0.00001:
break
x = x_next
print("方程的根为:", x)
```
这里使用了`scipy.stats`库中的`norm.ppf()`函数来计算Z分位数。请根据您的需要进行修改。
相关推荐
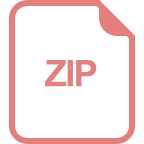
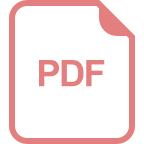










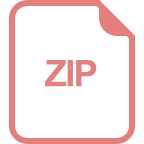
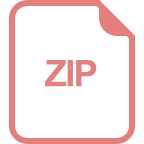