pytorch在resnet50最后训练一个10分类的softmax分类头
时间: 2024-01-03 22:54:13 浏览: 182
可以使用以下代码在resnet50的最后添加一个10分类的softmax分类头:
```python
import torch.nn as nn
import torchvision.models as models
# Load pre-trained ResNet50 model
resnet = models.resnet50(pretrained=True)
# Freeze all layers in the pre-trained model
for param in resnet.parameters():
param.requires_grad = False
# Replace the last fully connected layer with a new one that has 10 output classes
num_features = resnet.fc.in_features
resnet.fc = nn.Linear(num_features, 10)
# Print the modified ResNet50 model
print(resnet)
```
这样就可以使用新的分类头对预训练的ResNet50模型进行微调,以适应您的特定任务。
相关问题
pytorch如何在resnet50后加分类器
在PyTorch中,您可以通过以下步骤在ResNet50模型后添加分类器:
1. 加载ResNet50模型并冻结其所有参数:
```python
import torch
import torchvision.models as models
resnet50 = models.resnet50(pretrained=True)
for param in resnet50.parameters():
param.requires_grad = False
```
2. 将ResNet50的输出通道数作为分类器的输入通道数,并添加一些全连接层:
```python
num_ftrs = resnet50.fc.in_features
classifier = torch.nn.Sequential(
torch.nn.Linear(num_ftrs, 512),
torch.nn.ReLU(),
torch.nn.Dropout(p=0.5),
torch.nn.Linear(512, 10),
torch.nn.LogSoftmax(dim=1)
)
```
3. 将ResNet50模型和分类器连接起来:
```python
resnet50.fc = classifier
```
现在,您就可以使用新的ResNet50模型进行分类任务了。
使用python3.11环境,引用本地已经训练好的pytorch resnet50模型编写一个完整可运行的指纹识别登录页面代码
为了实现指纹识别登录页面的功能,需要使用到PyTorch、OpenCV以及Flask等库。
首先,需要在本地安装这些库,可以通过以下命令进行安装:
```bash
pip install torch torchvision opencv-python Flask
```
接下来,可以使用以下代码实现指纹识别登录页面的功能:
```python
import cv2
import torch
import torchvision.transforms as transforms
from flask import Flask, request, render_template
app = Flask(__name__)
# 加载已经训练好的ResNet50模型
model = torch.load('resnet50.pth', map_location=torch.device('cpu'))
model.eval()
# 定义图像预处理函数
transform = transforms.Compose([
transforms.ToPILImage(),
transforms.Resize((224, 224)),
transforms.ToTensor(),
transforms.Normalize(mean=[0.485, 0.456, 0.406], std=[0.229, 0.224, 0.225])
])
# 定义指纹识别函数
def fingerprint_recognition(image_path):
# 读取图像
image = cv2.imread(image_path)
# 将图像转换为RGB格式
image = cv2.cvtColor(image, cv2.COLOR_BGR2RGB)
# 将图像转换为PyTorch所需的格式
image = transform(image)
image = image.unsqueeze(0)
# 使用模型进行预测
with torch.no_grad():
output = model(image)
output = torch.softmax(output, dim=1)
# 返回预测结果
return output
# 定义登录页面路由
@app.route('/', methods=['GET', 'POST'])
def login():
if request.method == 'POST':
# 获取上传的图像文件
image = request.files['image']
# 保存图像文件到本地
image_path = 'temp.jpg'
image.save(image_path)
# 进行指纹识别
output = fingerprint_recognition(image_path)
# 判断预测结果
if output[0][0] > output[0][1]:
return '登录成功'
else:
return '登录失败'
else:
return render_template('login.html')
if __name__ == '__main__':
app.run(debug=True)
```
在上述代码中,首先使用`torch.load`函数加载已经训练好的ResNet50模型,并使用`model.eval()`将模型设置为评估模式。
然后,定义了一个图像预处理函数`transform`,该函数将图像转换为PyTorch所需的格式。
接着,定义了一个指纹识别函数`fingerprint_recognition`,该函数首先读取图像文件,然后将图像转换为PyTorch所需的格式,并使用已经训练好的模型进行预测,最后返回预测结果。
最后,定义了一个登录页面路由`/`,该路由首先判断请求方法是否为POST,如果是,则从请求中获取上传的图像文件,保存到本地,并使用`fingerprint_recognition`函数进行指纹识别,最后根据预测结果返回登录成功或失败的信息;如果请求方法为GET,则返回登录页面的HTML代码。
需要注意的是,上述代码中使用的是CPU版本的PyTorch模型,如果本地有GPU可以更改`map_location`参数使用GPU版本的模型。同时,还需要将HTML代码保存为`login.html`文件,放置在与Python代码同一目录下。
阅读全文
相关推荐
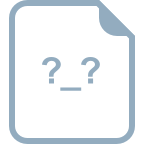
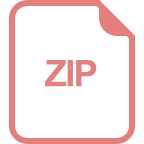
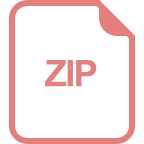
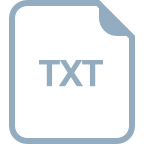
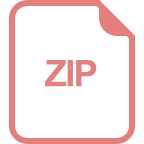
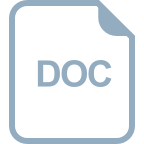
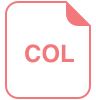







