image = image * np.array([0.229, 0.224, 0.225]) + np.array([0.485, 0.456, 0.406])
时间: 2024-05-22 20:14:49 浏览: 14
This code is performing an element-wise multiplication of the input image with two arrays, [0.229, 0.224, 0.225] and [0.485, 0.456, 0.406].
The first array is used to normalize the image by subtracting the mean of the ImageNet dataset, which is approximately [0.485, 0.456, 0.406]. The second array is used to standardize the image by dividing it by the standard deviation of the ImageNet dataset, which is approximately [0.229, 0.224, 0.225].
This normalization and standardization process is commonly used in deep learning models to ensure that the input data has zero mean and unit variance, which helps the model to converge faster and achieve better performance.
相关问题
image = image * np.array((0.229, 0.224, 0.225)) + np.array((0.485, 0.456, 0.406))
这段代码在进行图像预处理,将原始图像的每个像素值乘以一个系数,然后再减去另一个系数。这个操作是为了将图像的像素值归一化到一个较小的范围内,以便于神经网络的训练和推理。具体来说,这里使用了常用的 ImageNet 数据集的均值和标准差,即 (0.485, 0.456, 0.406) 和 (0.229, 0.224, 0.225)。这个操作可以提高模型的训练效果和泛化能力。
import numpy as np import pandas as pd import matplotlib.pyplot as plt import PIL import torch from torchvision import transforms import torchvision #调用已经训练好的FCN语义分割网络 model = torchvision.models.segmentation.fcn_resnet101(pretrained=True) model.eval() #读取照片 image=PIL.Image.open('1234.jpg') #照片进行预处理 image_transf=transforms.Compose([ transforms.ToTensor(), transforms.Normalize(mean=[0.485,0.456,0.406], std=[0.229,0.224,0.225]) ] ) image_tensor=image_transf(image).unsqueeze(0) output=model(image_tensor)['out'] output.shape #读取图片,进行分割,总共21个通道,因为在21个数据集上训练 #转化为2维图像 outputarg=torch.argmax(output.squeeze(),dim=0).numpy() outputarg def decode_seqmaps(image,label_colors,nc=21): r=np.zeros_like(image).astype(np.uint8) g=np.zeros_like(image).astype(np.uint8) b=np.zeros_like(image).astype(np.uint8) for cla in range(0,nc): idx = image == cla r[idx] = label_colors[cla,0] g[idx] = label_colors[cla,1] b[idx] = label_colors[cla,2] rgbimage= np.stack([r,g,b],axis=2) return rgbimage import os os.environ["KMP_DUPLICATE_LIB_OK"]="TRUE" label_colors =np.array([(0,0,0), #0=background (128,0,0),(0,128,0),(128,128,0),(0,0,128), #1=airplane,2=bicycle,3=bird,4=boat (128,0,128),(0,128,128),(128,128,128),(64,0,0), #6=bus,7=car,8=cat,9=chair (192,0,0),(64,128,0),(192,128,0),(64,0,128), #10=cow,11=dining table,12=dog,13=horse (192,0,128),(64,128,128),(192,128,128),(0,64,0), #14=motorbike,15=person,16=potted plant,17=sheep (128,64,0),(0,192,0),(128,192,0),(0,64,128) #18=sofa,19=train,20=tv/monitor ]) outputrgb=decode_seqmaps(outputarg,label_colors) plt.figure(figsize=(20,8)) plt.subplot(1,2,1) plt.imshow(image) plt.axis('off') plt.subplot(1,2,2) plt.imshow(outputrgb) plt.axis('off') plt.subplots_adjust(wspace=0.05) plt.show()使用了哪些深度学习的模型和方法
这段代码是导入了一些常用的Python库和工具,包括:
- numpy:用于数组和矩阵运算的库;
- pandas:用于数据分析和处理的库;
- matplotlib:用于数据可视化的库;
- PIL:Python图像库,用于图像处理和操作;
- torch:PyTorch深度学习框架的库;
- transforms:PyTorch中用于数据预处理的工具;
- torchvision:PyTorch中用于构建计算机视觉模型的框架。
这些库和工具都是在开发计算机视觉模型时会经常用到的,可以方便地处理图像数据、构建模型并进行训练和验证。
相关推荐
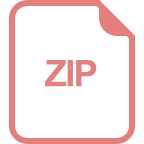
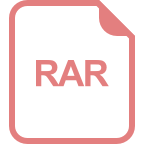












