keras.model在gpu运行
时间: 2023-09-23 21:07:21 浏览: 157
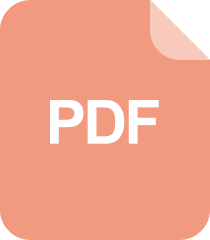
keras 多gpu并行运行案例
要让Keras模型在GPU上运行,需要确保以下几点:
1. 安装了GPU版本的TensorFlow或其他支持GPU的深度学习框架。
2. 在Keras中指定使用GPU作为计算设备。可以通过以下代码实现:
```python
import tensorflow as tf
from keras.backend.tensorflow_backend import set_session
# 在GPU上分配一部分显存
config = tf.ConfigProto()
config.gpu_options.per_process_gpu_memory_fraction = 0.5
set_session(tf.Session(config=config))
# 定义模型
model = Sequential()
...
# 编译模型
model.compile(...)
```
在这个例子中,我们使用TensorFlow的`ConfigProto`类来配置GPU选项。我们将`per_process_gpu_memory_fraction`设置为0.5,表示我们将在GPU上分配一半的显存。
然后,我们将TensorFlow会话的配置传递给Keras的`set_session`函数。这将确保Keras使用正确的TensorFlow会话。
最后,我们定义和编译模型就像平常一样。Keras会自动使用GPU来加速计算。
阅读全文
相关推荐







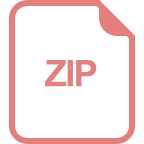








