data1=df_train.loc[(df_train['LABEL']==0)] data2=df_train.loc[(df_train['LABEL']==1)] x=data1["REVIEW_ID"] y=data1["RATING"] x1=data2["REVIEW_ID"] y2=data2["RATING"] plt.xlabel("REVIEW_ID") plt.ylabel("RATING") plt.show()
时间: 2023-06-17 22:07:57 浏览: 40
这是一段 Python 代码,通过 Pandas 和 Matplotlib 库来对数据进行可视化。假设 df_train 是一个 Pandas DataFrame,其中包含了一些电影评论的数据,包括 REVIEW_ID 和 RATING 两个字段。这段代码的作用是将 LABEL 为 0 和 1 的两类评论的 REVIEW_ID 和 RATING 分别取出,然后用 Matplotlib 画出它们的散点图,横轴为 REVIEW_ID,纵轴为 RATING。通过观察散点图,我们可以初步了解两类评论的分布情况和可能存在的差异。
相关问题
data1=df_train.loc[(df_train['PRODUCT_CATEGORY']==0)] data2=df_train.loc[(df_train['PRODUCT_CATEGORY']==1)] x=data1["LABEL"] y=data1["RATING"] x1=data2["LABEL"] y2=data2["RATING"] plt.xlabel("LABEL") plt.ylabel("RATING") plt.show()
这段代码的作用是将训练数据集中"PRODUCT_CATEGORY"列为0和1的两类数据分别存储到data1和data2中,然后将data1和data2中的"LABEL"和"RATING"分别存储到x、y和x1、y2中。最后,通过matplotlib库绘制散点图,横轴为"LABEL",纵轴为"RATING",展示两类数据的分布情况。
def set_data(df_0, df_1, df_9, cfg_dict): cfg_train_dict = cfg_dict['train'] df_train_1 = df_1.sample(len(df_1) - int(cfg_train_dict['simulate_pos_count']), random_state=int(cfg_train_dict['random_state'])) print('df_train_1 : ',len(df_train_1)) if cfg_train_dict['use_neg_sample'] == 'True': df_train_0 = df_0.copy() if len(df_0) >= len(df_1): df_train_0 = df_0.sample(len(df_1)) #else: # df_train_0 = df_0.append(df_9.sample(len(df_train_1) - len(df_0), # random_state=int(cfg_train_dict['random_state'])), # sort=False) else: df_train_0 = df_9.sample(round(len(df_train_1)), random_state=int(cfg_train_dict['random_state'])) df_train_0['label'] = 0 print('train set: pos_num--%i nag_num--%i' % (len(df_train_1), len(df_train_0))) df_train = df_train_1.append(df_train_0, sort=False) df_1_final_test = df_1.loc[list(set(df_1.index.tolist()).difference(set(df_train_1.index.tolist())))] #df_9_final_test = df_9.copy() 使负样本验证集等于正样本的验证集 df_9_final_test = df_9.sample(round(len(df_1_final_test)), random_state=int(cfg_train_dict['random_state'])) df_9_final_test['label'] = 0 df_ft = df_1_final_test.append(df_9_final_test, sort=False)
这段代码是一个名为 set_data 的函数,它用于设置数据集。
首先,函数从 cfg_dict 字典中获取了一个名为 cfg_train_dict 的子字典,其中包含了训练配置的相关参数。
接着,函数从 df_1 数据框中随机抽样了一部分样本作为训练集 df_train_1,抽样数量为 len(df_1) 减去 cfg_train_dict['simulate_pos_count'],random_state 参数用于设置随机种子。函数打印了 df_train_1 的长度。
然后,根据 cfg_train_dict['use_neg_sample'] 的值,函数决定如何选择负例样本。如果值为 'True',则将 df_0 数据框复制给 df_train_0,并根据 df_1 的长度进行抽样。如果 df_0 的长度大于等于 df_1 的长度,则抽样数量为 len(df_1);否则,函数还会从 df_9 数据框中抽样剩余的样本,抽样数量为 len(df_train_1) 减去 len(df_0),random_state 参数同样用于设置随机种子。
如果 cfg_train_dict['use_neg_sample'] 的值不为 'True',则直接从 df_9 数据框中抽样数量为 round(len(df_train_1)) 的样本作为负例样本,并将其赋值给 df_train_0。
接下来,函数给 df_train_0 添加了一个名为 'label' 的列,并将所有行的值都设置为 0。函数打印了 df_train_1 和 df_train_0 的长度。
然后,函数将 df_train_1 和 df_train_0 两个数据框按行合并成一个新的数据框 df_train。
接着,函数根据 df_train_1 的索引和 df_1 的索引的差异,获取了 df_1 中不在训练集中的样本,并将其赋值给 df_1_final_test。
接下来,函数从 df_9 数据框中随机抽样数量为 round(len(df_1_final_test)) 的样本作为负例测试集,并给其添加一个名为 'label' 的列,所有行的值都设置为 0。
最后,函数将 df_1_final_test 和 df_9_final_test 两个数据框按行合并成一个新的数据框 df_ft。
这段代码的作用是根据配置参数设置训练集和测试集。训练集由正例样本和负例样本组成,而测试集则包含了未在训练集中出现的正例样本和负例样本。
相关推荐
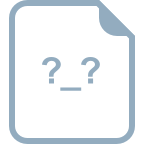
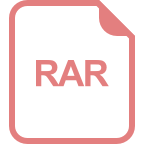












