temp = temp.transpose() # 打乱顺序 np.random.shuffle(temp) # print(temp) # 取出第一个元素作为 image 第二个元素作为 label image_list = temp[:, 0] label1_train = temp[:train_num, 1] # print(label1_train) # 单出,去掉单字符 label_train = [int(y) for y in label1_train] # print(label_train) label1_test = temp[train_num:, 1] label_test = [int(y) for y in label1_test]
时间: 2024-04-04 18:29:39 浏览: 17
这段代码是对加载的数据进行一些处理,包括打乱顺序、划分训练集和测试集,并将标签数据转换为 int 类型。首先,使用 temp.transpose() 函数将 temp 数组转置,然后使用 np.random.shuffle(temp) 函数将 temp 数组打乱顺序,以增加数据的随机性。接着,使用 temp[:, 0] 取出 temp 数组中的第一列作为图片路径,使用 temp[:train_num, 1] 取出前 train_num 行第二列作为训练集的标签,使用 temp[train_num:, 1] 取出后面的为测试集的标签。然后,使用列表推导式将标签数据转换为 int 类型,并分别将训练集和测试集的标签存储到 label_train 和 label_test 中。需要注意的是,该函数并没有对图片进行读取和预处理的操作,只是简单地将图片路径和标签存储到了列表中,并将标签转换为了 int 类型。
相关问题
import os import cv2 import numpy as np def load_data(file_dir): all_num = 4000 train_num = int(all_num * 0.75) cats = [] label_cats = [] dogs = [] label_dogs = [] for file in os.listdir(file_dir): file="\\"+file name = file.split(sep='.') if 'cat' in name[0]: cats.append(file_dir + file) label_cats.append(0) else: if 'dog' in name[0]: dogs.append(file_dir + file) label_dogs.append(1) image_list = np.hstack((cats,dogs)) label_list = np.hstack((label_cats, label_dogs)) temp = np.array([image_list, label_list]) # 矩阵转置 temp = temp.transpose() # 打乱顺序 np.random.shuffle(temp) # print(temp) # 取出第一个元素作为 image 第二个元素作为 label image_list = temp[:, 0] label1_train = temp[:train_num, 1] # print(label1_train) # 单出,去掉单字符 label_train = [int(y) for y in label1_train] # print(label_train) label1_test = temp[train_num:, 1] label_test = [int(y) for y in label1_test] data_test=[] data_train = [] for i in range (all_num): if i <train_num: image= image_list[i] image = cv2.imread(image) image = cv2.cvtColor(image, cv2.COLOR_BGR2GRAY) #将图片转换成RGB格式 image = cv2.resize(image, (28, 28)) image = image.astype('float32') image = np.array(image)/255#归一化[0,1] image=image.reshape(-1,28,28) data_train.append(image) # label_train.append(label_list[i]) else: image = image_list[i] image = cv2.imread(image) image = cv2.cvtColor(image, cv2.COLOR_BGR2GRAY) image = cv2.resize(image, (28, 28)) image = image.astype('float32') image = np.array(image) / 255 image = image.reshape(-1, 28, 28) data_test.append(image) # label_test.append(label_list[i]) data_train=np.array(data_train) label_train = np.array(label_train) data_test = np.array(data_test) label_test = np.array(label_test) return data_train,label_train,data_test, label_test
这段代码是定义了一个函数load_data,用来加载数据集,并将数据集划分为训练集和测试集。函数接收一个参数file_dir,表示数据集所在的路径。首先,定义了all_num和train_num两个变量,用来表示数据集的总数和训练集的数量,其中训练集的数量是总数的75%。然后,定义了四个空列表,分别用来存储猫的图片路径、猫的标签、狗的图片路径和狗的标签。接着,使用os.listdir函数遍历数据集路径下的所有文件,对每个文件进行判断,如果文件名中包含'cat'字符串,则将该文件的路径添加到cats列表中,并将标签0添加到label_cats列表中;如果文件名中包含'dog'字符串,则将该文件的路径添加到dogs列表中,并将标签1添加到label_dogs列表中。然后,使用numpy.hstack函数将猫和狗的图片路径和标签拼接成两个一维数组image_list和label_list。接着,使用numpy.array函数将image_list和label_list拼接成一个二维数组temp,并将其转置,使得图片路径和标签分别位于temp的第一列和第二列。然后,使用numpy.random.shuffle函数对temp进行打乱顺序操作。接着,将temp的第一列(即图片路径)赋值给image_list,将temp的前train_num行的第二列(即标签)赋值给label1_train,将temp的后面部分的第二列(即标签)赋值给label1_test。然后,将label1_train和label1_test从字符串类型转换为整型,并分别赋值给label_train和label_test。最后,调用前面提到的数据预处理代码,将image_list中的每张图片进行预处理,并将处理后的图片数据分别添加到data_train和data_test列表中,并将列表转换为numpy数组类型。最后,函数返回data_train、label_train、data_test和label_test四个变量。
mask = (torch.triu(torch.ones(sz, sz)) == 1).transpose(0, 1)什么意思
这行代码是用来生成一个掩码矩阵的,用于在自注意力机制中屏蔽模型访问未来位置的信息。
首先,torch.ones(sz, sz) 会生成一个大小为 (sz, sz) 的全为1的矩阵。然后,torch.triu() 函数将矩阵的下三角部分(包括对角线)全部变为0,只保留上三角部分,这是因为在自注意力机制中,模型只能访问当前位置及之前的位置,因此未来位置的信息需要被屏蔽。接着,通过 == 1 将上三角部分的值变为True,下三角部分的值变为False。最后,使用 .transpose(0, 1) 将矩阵进行转置,这是因为在PyTorch中,矩阵的维度顺序是 (行, 列),而在自注意力机制中,模型需要按列进行计算,因此需要将矩阵进行转置,使得行和列对应的是位置和时间步。
生成的掩码矩阵mask的大小为(sz, sz),其中mask[i][j]的值为True表示第i个位置不能访问第j个位置之后的信息,而mask[i][j]的值为False表示第i个位置可以访问第j个位置之后的信息。
相关推荐
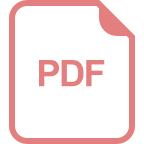
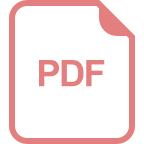












