均方根误差python代码
时间: 2023-10-13 08:28:38 浏览: 86
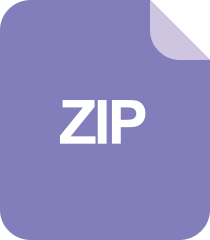
计算数据均方根误差的代码:这是一个简单的代码,可以准确计算真实或复杂数据的均方根误差。-matlab开发
均方根误差(Root Mean Square Error,RMSE)是衡量预测值与真实值之间差异的常用指标。在Python中,可以使用以下代码计算均方根误差:
```python
import numpy as np
def rmse(predictions, targets):
return np.sqrt(((predictions - targets) ** 2).mean())
# 示例
predictions = np.array([1, 2, 3])
targets = np.array([2, 3, 4])
print(rmse(predictions, targets)) # 输出0.816496580927726
```
在上面的示例中,我们首先导入NumPy库,然后定义了一个`rmse()`函数,该函数接受两个参数:预测值和真实值。接下来,我们使用NumPy的广播机制计算预测值与真实值之间的差异的平方,并取平均数。最后,我们再对平均数取平方根,得到均方根误差。
阅读全文
相关推荐
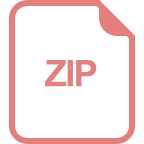















