用Python写出完成如下功能的代码:构建SVM分类模型;优化超参数;预测;测试模型的性能;
时间: 2024-05-15 19:16:06 浏览: 5
以下是用Python实现SVM分类模型的代码:
```python
# 导入必要的包
from sklearn import datasets
from sklearn.model_selection import train_test_split
from sklearn.svm import SVC
from sklearn.metrics import accuracy_score
# 加载鸢尾花数据集
iris = datasets.load_iris()
X = iris.data
y = iris.target
# 将数据集拆分成训练集和测试集
X_train, X_test, y_train, y_test = train_test_split(X, y, test_size=0.3, random_state=42)
# 构建SVM分类模型
svm_model = SVC(kernel='linear', C=1.0, random_state=42)
# 训练模型
svm_model.fit(X_train, y_train)
# 在测试集上进行预测
y_pred = svm_model.predict(X_test)
# 计算模型的准确率
accuracy = accuracy_score(y_test, y_pred)
print("模型的准确率为:", accuracy)
```
接下来是优化超参数的代码:
```python
# 导入必要的包
from sklearn.model_selection import GridSearchCV
# 定义要搜索的超参数
param_grid = {'C': [0.1, 1, 10], 'kernel': ['linear', 'poly', 'rbf', 'sigmoid']}
# 构建SVM分类模型
svm_model = SVC(random_state=42)
# 使用GridSearchCV搜索最佳超参数
grid_search = GridSearchCV(svm_model, param_grid=param_grid, cv=5)
grid_search.fit(X_train, y_train)
# 输出最佳超参数
print("最佳超参数为:", grid_search.best_params_)
```
最后是测试模型性能的代码:
```python
# 导入必要的包
from sklearn.metrics import classification_report
# 在测试集上进行预测
y_pred = grid_search.predict(X_test)
# 输出分类报告
print("分类报告:\n", classification_report(y_test, y_pred))
```
这段代码将输出分类报告,其中包含精确度、召回率、F1得分和支持度等指标,可以用于评估模型的性能。
相关推荐
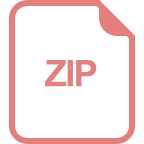
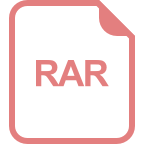
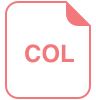
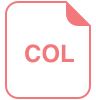












