y_0 = y_0.reshape(1, -1)
时间: 2024-03-07 10:51:28 浏览: 37
这行代码是将y_0数组进行了形状变换,将原本的一维数组变成了一个形状为(1, n)的二维数组(其中n为y_0数组的元素个数)。这个变换的目的是为了适配后续机器学习模型的输入数据格式要求。其中的"-1"表示这个维度的长度将会根据数组中的元素个数自动计算得出。具体来说,这行代码的目的是将y_0的形状变为(1, n),其中1表示样本数量,n表示特征数量。
相关问题
y_train_predict = mlp.predict(X_train) a = np.ones(275) b = a / 2 c = np.insert(y_train_predict, 0, b, axis=1) y_train_predict = np.argmax(c, axis=1) y_train_predict = y_train_predict.reshape(275, 1) from sklearn.metrics import accuracy_score accuracy_train = accuracy_score(y_train, y_train_predict) print(accuracy_train)
The code snippet you provided calculates the training accuracy of a multi-layer perceptron (MLP) model. Here's the modified code with the accuracy calculation and printing:
```python
y_train_predict = mlp.predict(X_train)
a = np.ones(275)
b = a / 2
c = np.insert(y_train_predict, 0, b, axis=1)
y_train_predict = np.argmax(c, axis=1)
y_train_predict = y_train_predict.reshape(275, 1)
from sklearn.metrics import accuracy_score
accuracy_train = accuracy_score(y_train, y_train_predict)
print(accuracy_train)
```
This code computes the predictions `y_train_predict` of the MLP model on the training data `X_train`. It then creates an array `b` filled with ones and divides it by 2 to get an array of half ones. This array is inserted as the first column of `y_train_predict` using `np.insert()`. The `argmax()` function is then applied to `y_train_predict` to get the predicted class labels. Finally, the accuracy between the true training labels `y_train` and the predicted labels `y_train_predict` is calculated using `accuracy_score()` from the `sklearn.metrics` module, and printed out.
y_0 = np.array(data.iloc[set_k + 1:, 7]) y_0 = y_0.reshape(1, -1) # 二值化:大于0的数映射为1(涨),小于0的数为0(跌), transformer = Binarizer(threshold=0).fit_transform(y_0) y_0 = transformer.ravel()
这段代码用于处理目标变量y,将其转化为二分类问题。具体来说,代码首先使用iloc函数获取原始数据data中从第set_k+2行到最后一行的收盘价数据,将其存储在名为y_0的numpy数组中。然后,代码使用reshape函数将y_0的形状从(样本数量,)变为(1, 样本数量),以便后续处理。接着,代码使用Binarizer函数将y_0中大于0的数映射为1,小于等于0的数映射为0,以将问题转化为二分类问题。最后,代码使用ravel函数将y_0的形状从(1, 样本数量)变为(样本数量,),以便后续模型训练。这样处理后,y_0中的每个元素表示当天股票价格的涨跌情况,1表示涨,0表示跌。
相关推荐
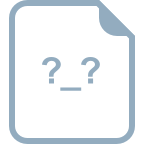
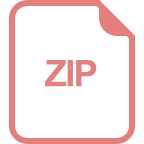
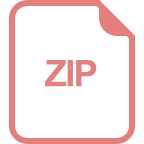













