model = LinearRegression() res = model.fit(x.reshape((len(x), 1)), y) # Fitting the linear regression predictions = model.predict(x.reshape((len(x), 1))) plt.plot(x, predictions) plt.show() print("intercept = ", res.intercept_, "solpe = ", res.coef_[0])
时间: 2023-06-03 21:04:23 浏览: 69
这段代码是在使用线性回归模型来进行数据拟合。其中,model = LinearRegression() 表示创建一个线性回归的模型对象;而 res = model.fit(x.reshape((len(x), 1)), y) 则是使用创建的模型对象,对输入的 x 和 y 数据进行训练并拟合得到结果 res。其中,x.reshape((len(x), 1)) 是对 x 进行 reshape 操作,将其从一维数据转化为二维数据,在此处是因为线性回归需要以二维数据作为输入。
相关问题
X = data['D'].values.reshape(-1, 1) y = data['E'].values.reshape(-1, 1) # 建立模型 model = LinearRegression() model.fit(X, y)优化这段代码
这段代码可以进行以下优化:
1. 将 reshape 操作合并到数据读取操作中,避免重复的操作:
```
X = data['D'].to_numpy().reshape(-1, 1)
y = data['E'].to_numpy().reshape(-1, 1)
```
2. 使用 train_test_split 函数将数据集划分为训练集和测试集,避免过拟合和提高模型的泛化能力:
```
from sklearn.model_selection import train_test_split
X_train, X_test, y_train, y_test = train_test_split(X, y, test_size=0.2, random_state=42)
```
3. 在模型训练之前,可以进行数据标准化操作,将数据缩放到相同的范围内,提高模型的性能:
```
from sklearn.preprocessing import StandardScaler
scaler = StandardScaler()
X_train = scaler.fit_transform(X_train)
X_test = scaler.transform(X_test)
```
4. 可以尝试使用其他的回归模型,如 Ridge 或 Lasso 回归,来避免线性回归模型的欠拟合问题。
```
from sklearn.linear_model import Ridge
model = Ridge(alpha=0.1)
model.fit(X_train, y_train)
```
model = regression.fit(feature.reshape(-1, 1), label)中的label的作用
### 回答1:
label在这个语句中的作用是作为训练数据的目标值,用于监督学习中的回归模型训练。具体来说,feature是输入的特征数据,label是对应的输出数据,通过训练回归模型,可以建立输入特征和输出数据之间的映射关系,从而实现对未知数据的预测。
### 回答2:
在"model = regression.fit(feature.reshape(-1, 1), label)"中,label的作用是为了训练模型。在机器学习中,我们通常有一组特征(features)和对应的目标变量(label)。特征是用来描述数据的各个方面,而目标变量是我们希望通过特征预测或者分析的变量。
在给定特征和目标变量的情况下,我们可以使用一个回归模型(regression model)进行训练。回归模型的目的是通过特征预测目标变量的数值。在上述代码中,使用regression.fit()函数将特征和目标变量作为输入来训练模型。
label在这里就是目标变量,它用来告诉模型所要预测的值是什么。通过将特征和对应的目标变量提供给模型,模型可以根据特征的变化来学习目标变量的模式和关联性。在训练过程中,模型会调整自身的参数,以最小化特征和目标变量之间的差异。
因此,在这个特定的代码中,label的作用是为了指定要训练的模型需要预测的目标变量。特征和目标变量的组合可以用来训练回归模型,使其能够更好地预测特征与目标变量之间的关系。
### 回答3:
在机器学习中,label代表着样本的真实标签或输出值。model = regression.fit(feature.reshape(-1, 1), label)中的label参数用来训练回归模型。该模型的目标是通过输入的feature来预测或估计与之相关的label。
这个过程中,label的作用是为模型提供有监督学习的训练样本。通过将特征feature和对应的标签label传递给机器学习算法,模型可以根据数据的模式和关系来学习特征与标签之间的映射关系。训练过程中,模型会根据给定的特征和对应的标签进行参数调整和优化,以使模型能够对未知的特征进行准确的预测。
对于回归问题,label通常是连续的数值或实数,如预测一个房屋的售价或一个人的年龄。通过训练样本中特征和真实标签的对应关系,回归模型可以学习到特征与标签之间的数学模型,从而可以根据给定的特征来预测相应的标签值。
总之,label参数在模型训练过程中的作用是提供样本的真实标签或输出值,用于训练回归模型,并使其能够准确预测具有相似特征的未知样本的相关标签。
相关推荐
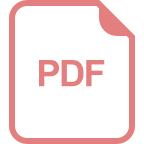
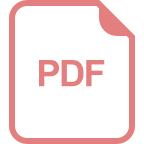
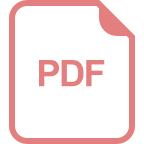













