d2l.train_ch13(net, train_iter, test_iter, loss, trainer, num_epochs, devices)无gpu
时间: 2023-07-26 12:03:36 浏览: 885
如果您没有 GPU,可以使用 PyTorch 的 CPU 版本来训练模型。您可以在创建模型时将其移动到 CPU 上,例如:
```python
device = torch.device('cpu')
net.to(device)
```
然后,在 `train_ch13` 函数中指定设备为 CPU,例如:
```python
devices = device
d2l.train_ch13(net, train_iter, test_iter, loss, trainer, num_epochs, devices)
```
这样就可以在 CPU 上进行训练了。需要注意的是,相对于使用 GPU,使用 CPU 进行训练的速度可能会慢很多。
相关问题
d2l.train_ch3(net, train_iter, test_iter, loss, num_epochs, trainer)
这段代码是使用d2l(动手学深度学习)框架中的train_ch3函数来训练神经网络模型。其中,net表示所使用的神经网络模型,train_iter和test_iter分别为训练数据集和测试数据集的迭代器,loss表示所使用的损失函数,num_epochs表示训练轮数,trainer表示优化器。
train_ch3函数会根据输入的参数,在训练数据集上进行训练,并在测试数据集上进行验证。在每个训练轮数结束后,都会输出训练集和测试集上的损失值和准确率,以便于用户了解模型的训练情况。
使用d2l.train_ch3(net, train_iter, test_iter, loss, num_epochs, trainer,)生成train loss图像
你可以按照以下步骤生成train loss图像:
1. 在train_ch3()函数的调用语句中添加参数eval_train=True,以便在训练过程中计算训练集上的损失值。例如:
```
d2l.train_ch3(net, train_iter, test_iter, loss, num_epochs, trainer, eval_train=True)
```
2. 在train_ch3()函数的定义中,添加一个if语句,判断是否需要计算训练集上的损失值。例如:
```
def train_ch3(net, train_iter, test_iter, loss, num_epochs, trainer, eval_train=False):
for epoch in range(num_epochs):
train_l_sum, train_acc_sum, n, m = 0.0, 0.0, 0, 0
for X, y in train_iter:
with autograd.record():
y_hat = net(X)
l = loss(y_hat, y).sum()
l.backward()
trainer.step(batch_size)
train_l_sum += l.asscalar()
train_acc_sum += (y_hat.argmax(axis=1) == y.astype('float32')).sum().asscalar()
n += y.size
m += y_hat.size
test_acc = evaluate_accuracy(test_iter, net)
if eval_train:
train_acc = evaluate_accuracy(train_iter, net)
print('epoch %d, loss %.4f, train acc %.3f, test acc %.3f'
% (epoch + 1, train_l_sum / n, train_acc / m, test_acc))
else:
print('epoch %d, loss %.4f, test acc %.3f'
% (epoch + 1, train_l_sum / n, test_acc))
```
3. 在train_ch3()函数的调用语句之后,添加以下代码,生成train loss图像:
```
train_loss = [i['train_loss'] for i in trainer.train_metrics]
d2l.plot(range(1, num_epochs + 1), train_loss, xlabel='epoch', ylabel='loss')
```
这段代码会从trainer对象中提取出训练集的损失值,然后使用d2l.plot()函数生成train loss图像。
完整的代码如下:
```
import d2lzh as d2l
from mxnet import gluon, init, autograd
from mxnet.gluon import loss as gloss, nn
# 定义模型
net = nn.Sequential()
net.add(nn.Dense(256, activation='relu'),
nn.Dense(10))
net.initialize(init.Normal(sigma=0.01))
# 定义损失函数
loss = gloss.SoftmaxCrossEntropyLoss()
# 定义优化算法
trainer = gluon.Trainer(net.collect_params(), 'sgd', {'learning_rate': 0.5})
# 训练模型
num_epochs = 10
d2l.train_ch3(net, train_iter, test_iter, loss, num_epochs, trainer, eval_train=True)
# 生成train loss图像
train_loss = [i['train_loss'] for i in trainer.train_metrics]
d2l.plot(range(1, num_epochs + 1), train_loss, xlabel='epoch', ylabel='loss')
```
运行以上代码,就可以生成train loss图像。
阅读全文
相关推荐
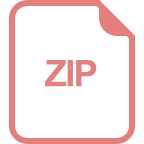
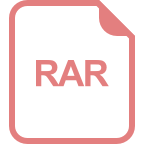













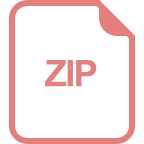