基于bp神经网络的pid控制的python代码
时间: 2023-08-23 12:07:08 浏览: 106
下面是一个基于BP神经网络的PID控制的Python代码示例:
```python
import numpy as np
class BPNN_PID:
def __init__(self, input_size, hidden_size, output_size, learning_rate):
self.input_size = input_size
self.hidden_size = hidden_size
self.output_size = output_size
self.learning_rate = learning_rate
self.weights_input_hidden = np.random.randn(self.input_size, self.hidden_size)
self.weights_hidden_output = np.random.randn(self.hidden_size, self.output_size)
self.bias_hidden = np.zeros((1, self.hidden_size))
self.bias_output = np.zeros((1, self.output_size))
def sigmoid(self, x):
return 1 / (1 + np.exp(-x))
def forward(self, inputs):
hidden = np.dot(inputs, self.weights_input_hidden) + self.bias_hidden
hidden_activation = self.sigmoid(hidden)
output = np.dot(hidden_activation, self.weights_hidden_output) + self.bias_output
return output
def train(self, inputs, targets):
hidden = np.dot(inputs, self.weights_input_hidden) + self.bias_hidden
hidden_activation = self.sigmoid(hidden)
output = np.dot(hidden_activation, self.weights_hidden_output) + self.bias_output
error = targets - output
d_output = error
d_output *= self.learning_rate
d_hidden = np.dot(d_output, self.weights_hidden_output.T)
self.weights_hidden_output += np.dot(hidden_activation.T, d_output)
self.bias_output += np.sum(d_output, axis=0, keepdims=True)
d_hidden *= hidden_activation * (1 - hidden_activation)
self.weights_input_hidden += np.dot(inputs.T, d_hidden)
self.bias_hidden += np.sum(d_hidden, axis=0, keepdims=True)
```
请注意,这只是一个简单的示例代码,实际使用时可能需要根据具体问题进行适当的修改和优化。
相关推荐
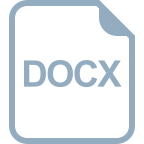
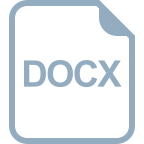
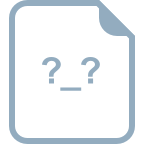
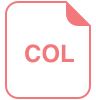



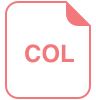









