cmd=queryReferenceAll&conditionSql=AM_ACTUAL_ACCNTINFO.PK_ACTUAL_ACCNT in (select distinct (t.pk_actual_accnt) from RA_CORP_ACCNTINFO t where t.company_id = '1012201100000004491' and t.site_code = 'C00100M')
时间: 2024-04-05 16:34:18 浏览: 81
这是一个查询语句,用于从数据库中获取符合特定条件的数据。具体来说,这个查询语句的作用是查询具有特定公司ID和站点代码的实际账户信息,然后使用这些信息从另一个表中检索参考数据。
在查询语句中,cmd参数指定要执行的命令为queryReferenceAll,conditionSql参数指定查询条件为AM_ACTUAL_ACCNTINFO.PK_ACTUAL_ACCNT在一个子查询中的结果中,该子查询检索具有特定公司ID和站点代码的RA_CORP_ACCNTINFO表中的实际账户信息。
相关问题
import pandas as pd import numpy as np import os from pprint import pprint from pandas import DataFrame from scipy import interpolate data_1_hour_predict_raw = pd.read_excel('./data/附件1 监测点A空气质量预报基础数据.xlsx' ) data_1_hour_actual_raw = pd.read_excel('./data/附件1 监测点A空气质量预报基础数据.xlsx' ) data_1_day_actual_raw = pd.rea df_1_predict = data_1_hour_actual_raw df_1_actual = data_1_day_actual_raw df_1_predict.set_axis( ['time', 'place', 'so2', 'no2', 'pm10', 'pm2.5', 'o3', 'co', 'temperature', 'humidity', 'pressure', 'wind', 'direction'], axis='columns', inplace=True) df_1_actual.set_axis(['time', 'place', 'so2', 'no2', 'pm10', 'pm2.5', 'o3', 'co'], axis='columns', inplace=True) modeltime_df_actual = df_1_actual['time'] modeltime_df_pre = df_1_predict['time'] df_1_actual = df_1_actual.drop(columns=['place', 'time']) df_1_predict = df_1_predict.drop(columns=['place', 'time']) df_1_predict = df_1_predict.replace('—', np.nan) df_1_predict = df_1_predict.astype('float') df_1_predict[df_1_predict < 0] = np.nan # 重新插入time列 df_1_actual.insert(0, 'time', modeltime_df_actual) df_1_predict.insert(0, 'time', modeltime_df_pre) # 线性插值的方法需要单独处理最后一行的数据 data_1_actual = df_1_actual[0:-3] data_1_predict = df_1_predict data_1_predict.iloc[-1:]['pm10'] = 22.0 data_1_actual_knn = df_1_actual[0:-3] data_1_predict_knn: DataFrame = df_1_predict for indexs in data_1_actual.columns: if indexs == 'time': continue data_1_actual['rownum'] = np.arange(data_1_actual.shape[0]) df_nona = data_1_actual.dropna(subset=[indexs]) f = interpolate.interp1d(df_nona['rownum'], df_nona[indexs]) data_1_actual[indexs] = f(data_1_actual['rownum']) data_1_actual = data_1_actual.drop(columns=['rownum']) for indexs in data_1_predict.columns: if indexs == 'time': continue data_1_predict['rownum'] = np.arange(data_1_predict.shape[0]) df_nona = data_1_predict.dropna(subset=[indexs]) f = interpolate.interp1d(df_nona['rownum'], df_nona[indexs]) data_1_predict[indexs] = f(data_1_predict['rownum']) data_1_predict = data_1_predict.drop(columns=['rownum']) writer = pd.E
这段代码主要是对一份空气质量预报基础数据进行处理和插值,具体的解释如下:
1. 导入需要的库和模块:
```
import pandas as pd
import numpy as np
import os
from pprint import pprint
from pandas import DataFrame
from scipy import interpolate
```
2. 读取 excel 文件中的数据:
```
data_1_hour_predict_raw = pd.read_excel('./data/附件1 监测点A空气质量预报基础数据.xlsx' )
data_1_hour_actual_raw = pd.read_excel('./data/附件1 监测点A空气质量预报基础数据.xlsx' )
data_1_day_actual_raw = pd.read_excel('./data/附件1 监测点A空气质量预报基础数据.xlsx' )
```
3. 对读取的数据进行处理:
```
df_1_predict = data_1_hour_actual_raw
df_1_actual = data_1_day_actual_raw
df_1_predict.set_axis( ['time', 'place', 'so2', 'no2', 'pm10', 'pm2.5', 'o3', 'co', 'temperature', 'humidity', 'pressure', 'wind', 'direction'], axis='columns', inplace=True)
df_1_actual.set_axis(['time', 'place', 'so2', 'no2', 'pm10', 'pm2.5', 'o3', 'co'], axis='columns', inplace=True)
```
4. 提取时间列并进行插值:
```
modeltime_df_actual = df_1_actual['time']
modeltime_df_pre = df_1_predict['time']
df_1_actual = df_1_actual.drop(columns=['place', 'time'])
df_1_predict = df_1_predict.drop(columns=['place', 'time'])
df_1_predict = df_1_predict.replace('—', np.nan)
df_1_predict = df_1_predict.astype('float')
df_1_predict[df_1_predict < 0] = np.nan
df_1_actual.insert(0, 'time', modeltime_df_actual)
df_1_predict.insert(0, 'time', modeltime_df_pre)
data_1_actual = df_1_actual[0:-3]
data_1_predict = df_1_predict
data_1_predict.iloc[-1:]['pm10'] = 22.0
data_1_actual_knn = df_1_actual[0:-3]
data_1_predict_knn: DataFrame = df_1_predict
for indexs in data_1_actual.columns:
if indexs == 'time':
continue
data_1_actual['rownum'] = np.arange(data_1_actual.shape[0])
df_nona = data_1_actual.dropna(subset=[indexs])
f = interpolate.interp1d(df_nona['rownum'], df_nona[indexs])
data_1_actual[indexs] = f(data_1_actual['rownum'])
data_1_actual = data_1_actual.drop(columns=['rownum'])
for indexs in data_1_predict.columns:
if indexs == 'time':
continue
data_1_predict['rownum'] = np.arange(data_1_predict.shape[0])
df_nona = data_1_predict.dropna(subset=[indexs])
f = interpolate.interp1d(df_nona['rownum'], df_nona[indexs])
data_1_predict[indexs] = f(data_1_predict['rownum'])
data_1_predict = data_1_predict.drop(columns=['rownum'])
```
5. 最后将处理好的数据写入 excel 文件:
```
writer = pd.ExcelWriter('./data/附件1 监测点A空气质量预报基础数据_preprocessed.xlsx')
data_1_predict.to_excel(writer, sheet_name='1小时预测数据', index=False)
data_1_predict_knn.to_excel(writer, sheet_name='1小时预测数据_knn', index=False)
data_1_actual.to_excel(writer, sheet_name='1天实际数据', index=False)
data_1_actual_knn.to_excel(writer, sheet_name='1天实际数据_knn', index=False)
writer.save()
```
总体来说,这段代码主要是对空气质量预报基础数据进行了一些预处理和插值,最终将处理好的数据写入了 excel 文件中。
java.sql.SQLException: sql injection violation, syntax error: TODO : pos 872, line 15, column 43, token UNION : SELECT asewo.code_s as code,asewo.order_type_s as orderType,asewo.equipment_name_s as equipmentName,asewo.executor_s as executor, asewo.plan_start_time_T as planStartTime,asewo.plan_finish_time_T as planFinishTime,asewo.tpm_key_s as tpmKey,asewo.shop_s as shop, asewo.line_S as line,asewo.actual_start_time_t as startTime,asewo.actual_finish_time_t as finishTime,asewo.executor_account_s as executorAccounts, asewo.source_type_s as sourceType,asewo.SOURCE_ORDER_S as sourceOrder,asewo.CREATION_TIME as creationTime FROM AT_AS_EM_Work_Order asewo WHERE to_char(asewo.plan_start_time_T,'yyyy_mm-dd hh24:mi') <= to_char(plan_finish_time_T,'yyyy_mm_dd hh24:mi') AND asewo.ORDER_STATUS_S='创建' AND asewo.executor_s=? ORDER BY asewo.creation_time DESC Union All SELECT asewo.code_s as code,asewo.order_type_s as orderType,asewo.equipment_name_s as equipmentName,asewo.executor_s as executor, asewo.plan_start_time_T as planStartTime,asewo.plan_finish_time_T as planFinishTime,asewo.tpm_key_s as tpmKey,asewo.shop_s as shop, asewo.line_S as line,asewo.actual_start_time_t as startTime,asewo.actual_finish_time_t as finishTime,asewo.executor_account_s as executorAccounts, asewo.source_type_s as sourceType,asewo.SOURCE_ORDER_S as sourceOrder,asewo.CREATION_TIME as creationTime FROM AT_AS_EM_Work_Order asewo WHERE asewo.ORDER_STATUS_S='创建' AND asewo.executor_s=? ORDER BY asewo.creation_time DESC如何改
这段代码是一个SQL查询语句,其中包含了一个UNION操作符,因此可能存在SQL注入漏洞。如果要避免注入漏洞,可以使用参数化查询来构建SQL语句。具体来说,可以将查询中的变量使用占位符(如?)代替,然后在执行查询时将变量的值传递给占位符。这样可以确保输入的变量不会被当做SQL语句的一部分来解释,从而避免注入攻击。
以下是一个使用参数化查询的示例代码:
```java
String sql = "SELECT asewo.code_s as code,asewo.order_type_s as orderType,asewo.equipment_name_s as equipmentName,asewo.executor_s as executor, "
+ "asewo.plan_start_time_T as planStartTime,asewo.plan_finish_time_T as planFinishTime,asewo.tpm_key_s as tpmKey,asewo.shop_s as shop, "
+ "asewo.line_S as line,asewo.actual_start_time_t as startTime,asewo.actual_finish_time_t as finishTime,asewo.executor_account_s as executorAccounts, "
+ "asewo.source_type_s as sourceType,asewo.SOURCE_ORDER_S as sourceOrder,asewo.CREATION_TIME as creationTime "
+ "FROM AT_AS_EM_Work_Order asewo "
+ "WHERE to_char(asewo.plan_start_time_T,'yyyy_mm-dd hh24:mi') <= to_char(plan_finish_time_T,'yyyy_mm_dd hh24:mi') "
+ "AND asewo.ORDER_STATUS_S='创建' "
+ "AND asewo.executor_s=? "
+ "ORDER BY asewo.creation_time DESC "
+ "UNION All "
+ "SELECT asewo.code_s as code,asewo.order_type_s as orderType,asewo.equipment_name_s as equipmentName,asewo.executor_s as executor, "
+ "asewo.plan_start_time_T as planStartTime,asewo.plan_finish_time_T as planFinishTime,asewo.tpm_key_s as tpmKey,asewo.shop_s as shop, "
+ "asewo.line_S as line,asewo.actual_start_time_t as startTime,asewo.actual_finish_time_t as finishTime,asewo.executor_account_s as executorAccounts, "
+ "asewo.source_type_s as sourceType,asewo.SOURCE_ORDER_S as sourceOrder,asewo.CREATION_TIME as creationTime "
+ "FROM AT_AS_EM_Work_Order asewo "
+ "WHERE asewo.ORDER_STATUS_S='创建' "
+ "AND asewo.executor_s=? "
+ "ORDER BY asewo.creation_time DESC";
PreparedStatement stmt = connection.prepareStatement(sql);
stmt.setString(1, executor);
stmt.setString(2, executor);
ResultSet rs = stmt.executeQuery();
```
在上面的代码中,使用`PreparedStatement`来创建查询语句,并将占位符替换为变量。然后可以使用`setString`等方法来设置变量的值,最后执行查询并获取结果。通过使用参数化查询,可以有效地避免SQL注入漏洞。
阅读全文
相关推荐
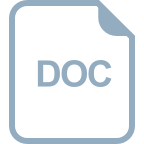
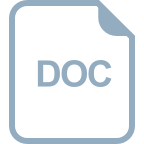
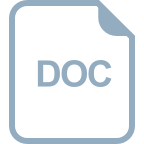






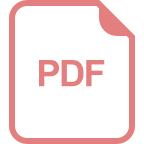
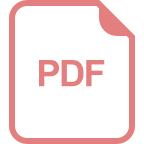






