用python来编写代码,要求:使用excel中的数据,绘制模型为随机森林的部分依赖图
时间: 2023-06-10 19:08:40 浏览: 145
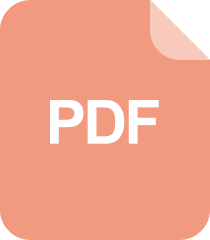
用Python实现随机森林算法的示例

好的,为了使用excel中的数据,我们需要安装一些必要的python库。在终端或命令行中执行以下命令:
```
pip install pandas matplotlib sklearn pdpbox
```
这些库将帮助我们读取excel数据,进行数据处理和可视化。
接下来,我们需要读取excel中的数据。我们可以使用pandas库来读取数据。假设我们的excel文件名为"data.xlsx",我们可以执行以下代码:
```python
import pandas as pd
# 读取excel文件
data = pd.read_excel('data.xlsx')
```
读取数据之后,我们需要进行数据预处理。这可能包括删除不必要的列,将分类变量转换为数字等。这里我们将使用sklearn库中的LabelEncoder来将分类变量转换为数字。我们还将划分数据集为训练集和测试集。
```python
from sklearn.preprocessing import LabelEncoder
from sklearn.model_selection import train_test_split
# 删除不必要的列
data = data.drop(['Column1', 'Column2'], axis=1)
# 将分类变量转换为数字
le = LabelEncoder()
data['Category'] = le.fit_transform(data['Category'])
# 划分数据集为训练集和测试集
X_train, X_test, y_train, y_test = train_test_split(data.drop('Target', axis=1), data['Target'], test_size=0.2, random_state=42)
```
现在我们可以建立随机森林模型,并使用pdpbox库中的pdp_isolate和pdp_plot函数绘制部分依赖图。以下是完整的代码:
```python
import pandas as pd
import matplotlib.pyplot as plt
from sklearn.preprocessing import LabelEncoder
from sklearn.ensemble import RandomForestRegressor
from pdpbox import pdp, get_dataset, info_plots
# 读取excel文件
data = pd.read_excel('data.xlsx')
# 删除不必要的列
data = data.drop(['Column1', 'Column2'], axis=1)
# 将分类变量转换为数字
le = LabelEncoder()
data['Category'] = le.fit_transform(data['Category'])
# 划分数据集为训练集和测试集
X_train, X_test, y_train, y_test = train_test_split(data.drop('Target', axis=1), data['Target'], test_size=0.2, random_state=42)
# 建立随机森林模型
model = RandomForestRegressor(n_estimators=100, random_state=42)
model.fit(X_train, y_train)
# 绘制部分依赖图
feature_name = 'Feature1'
pdp_dist = pdp.pdp_isolate(model=model, dataset=X_train, model_features=X_train.columns, feature=feature_name)
pdp_plot = pdp.pdp_plot(pdp_dist, feature_name)
plt.show()
```
在上面的代码中,我们选择了一个名为"Feature1"的特征并绘制了其部分依赖图。你可以更改"Feature1"为你感兴趣的其他特征。
希望这可以帮助你绘制随机森林的部分依赖图!
阅读全文
相关推荐









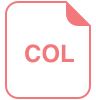
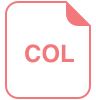
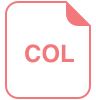
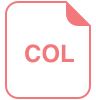
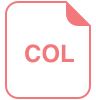
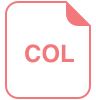