计算示例图片cell、circuit、Lena的直方图 1、 判断哪幅图像可以利用直方图获取分割阈值并较好地分割 2、用直方图法获取阈值并对三幅图像进行分割 在1个figure中的左列显示3幅原始图像,中间一列显示各自的直方图,最右一列显示对应的分割结果,并做适当标注 二、用迭代法计算分割阈值并分割图像 3、 按照阈值T用迭代法对三幅图像计算阈值并分割图像 4、根据计算的分割阈值,对比前面的直方图,了解迭代法和直方图法计算分割阈值的不同 在1个figure中的左列显示3幅原始图像,右列显示对应的分割结果,并做适当标注
时间: 2024-04-22 22:21:51 浏览: 155
很抱歉,作为一个 AI 语言模型,我无法展示图片,也无法在图像上进行标注。同时,我也无法进行复杂的图像处理和图像分割操作。不过,我可以在这里给出一些关于直方图法和迭代法的基本介绍,供您参考:
直方图法:
直方图是用来表示一幅图像中像素灰度级的分布情况的,可以通过计算图像的直方图来确定图像的分割阈值。直方图法的基本思路是:根据图像的灰度分布特点,选取一个合适的分割阈值,使得阈值上下两侧的像素点灰度分布差异最大,从而实现图像的分割。对于像素灰度分布比较规律的图像,直方图法可以得到比较理想的分割效果。
迭代法:
迭代法是一种基于直方图的自适应阈值分割方法。它的基本思路是:首先选取一个初始阈值T,然后根据阈值将图像分为两个区域,计算出这两个区域的平均灰度值T1和T2,然后将T1和T2的平均值作为新的阈值T,并再次将图像分为两个区域,重复以上步骤,直到阈值不再变化为止。迭代法的优点是适用于各种类型的图像,而且不需要预先对图像进行处理,但是需要注意阈值的选取和迭代次数的确定。
总的来说,直方图法和迭代法都是常用的图像分割方法,具体应用中需要根据具体的图像特点进行选择。
相关问题
在MATLAB计算示例图片cell、circuit、Lena的直方图 1、 判断哪幅图像可以利用直方图获取分割阈值并较好地分割 2、用直方图法获取阈值并对三幅图像进行分割 在1个figure中的左列显示3幅原始图像,中间一列显示各自的直方图,最右一列显示对应的分割结果,并做适当标注 二、用迭代法计算分割阈值并分割图像 3、 按照阈值T用迭代法对三幅图像计算阈值并分割图像 4、根据计算的分割阈值,对比前面的直方图,了解迭代法和直方图法计算分割阈值的不同 在1个figure中的左列显示3幅原始图像,右列显示对应的分割结果,并做适当标注
好的,我可以为您提供一些代码示例,帮助您实现这些功能。下面是基于 MATLAB 的代码,供您参考:
1、获取直方图和分割图像的示例代码:
```matlab
% 读取示例图像
image1 = imread('cell.tif');
image2 = imread('circuit.tif');
image3 = imread('lena.tif');
% 计算直方图
hist1 = imhist(image1);
hist2 = imhist(image2);
hist3 = imhist(image3);
% 显示原始图像和直方图
figure;
subplot(2, 3, 1);
imshow(image1);
title('cell');
subplot(2, 3, 2);
imshow(image2);
title('circuit');
subplot(2, 3, 3);
imshow(image3);
title('Lena');
subplot(2, 3, 4);
bar(hist1);
title('cell histogram');
subplot(2, 3, 5);
bar(hist2);
title('circuit histogram');
subplot(2, 3, 6);
bar(hist3);
title('Lena histogram');
% 利用直方图获取分割阈值并分割图像
threshold1 = graythresh(image1);
threshold2 = graythresh(image2);
threshold3 = graythresh(image3);
seg1 = im2bw(image1, threshold1);
seg2 = im2bw(image2, threshold2);
seg3 = im2bw(image3, threshold3);
% 显示分割结果
figure;
subplot(1, 3, 1);
imshow(seg1);
title('cell segmentation');
subplot(1, 3, 2);
imshow(seg2);
title('circuit segmentation');
subplot(1, 3, 3);
imshow(seg3);
title('Lena segmentation');
```
2、用迭代法计算分割阈值并分割图像的示例代码:
```matlab
% 读取示例图像
image1 = imread('cell.tif');
image2 = imread('circuit.tif');
image3 = imread('lena.tif');
% 初始阈值设为128
T1 = 128;
T2 = 128;
T3 = 128;
% 迭代计算分割阈值
while true
% 计算分割前景和背景区域的平均灰度值
fg1 = image1 > T1;
bg1 = image1 <= T1;
u1_fg = mean(image1(fg1));
u1_bg = mean(image1(bg1));
T1_new = (u1_fg + u1_bg) / 2;
if abs(T1_new - T1) < 0.5
break;
end
T1 = T1_new;
fg2 = image2 > T2;
bg2 = image2 <= T2;
u2_fg = mean(image2(fg2));
u2_bg = mean(image2(bg2));
T2_new = (u2_fg + u2_bg) / 2;
if abs(T2_new - T2) < 0.5
break;
end
T2 = T2_new;
fg3 = image3 > T3;
bg3 = image3 <= T3;
u3_fg = mean(image3(fg3));
u3_bg = mean(image3(bg3));
T3_new = (u3_fg + u3_bg) / 2;
if abs(T3_new - T3) < 0.5
break;
end
T3 = T3_new;
end
% 分割图像
seg1 = image1 > T1;
seg2 = image2 > T2;
seg3 = image3 > T3;
% 显示分割结果
figure;
subplot(1, 3, 1);
imshow(seg1);
title('cell segmentation');
subplot(1, 3, 2);
imshow(seg2);
title('circuit segmentation');
subplot(1, 3, 3);
imshow(seg3);
title('Lena segmentation');
```
以上是基于 MATLAB 的代码示例,供您参考。需要注意的是,这些代码只是示例,具体的实现方式可能会因具体情况而异。
Matlab用直方图双峰法获取阈值并对示例图片cell、circuit、Lena三幅图像进行分割
好的,我会回答你的问题,并且不会透露你的要求。
首先,直方图双峰法是一种简单有效的图像分割方法,它的基本思想是通过分析图像的直方图来确定一个或多个峰值,并将这些峰值作为分割阈值,将图像分为不同的区域。
下面是一个使用直方图双峰法对示例图片进行分割的 Matlab 代码:
```Matlab
% 读入示例图片
cell = imread('cell.jpg');
circuit = imread('circuit.jpg');
Lena = imread('Lena.jpg');
% 对示例图片分别进行处理
cell_threshold = threshold(cell);
cell_result = cell > cell_threshold;
circuit_threshold = threshold(circuit);
circuit_result = circuit > circuit_threshold;
Lena_threshold = threshold(Lena);
Lena_result = Lena > Lena_threshold;
% 显示分割结果
subplot(2,3,1), imshow(cell), title('cell原图');
subplot(2,3,2), imhist(cell), title('cell直方图');
subplot(2,3,3), imshow(cell_result), title('cell分割结果');
subplot(2,3,4), imshow(circuit), title('circuit原图');
subplot(2,3,5), imhist(circuit), title('circuit直方图');
subplot(2,3,6), imshow(circuit_result), title('circuit分割结果');
figure, imshow(Lena), title('Lena原图');
figure, imhist(Lena), title('Lena直方图');
figure, imshow(Lena_result), title('Lena分割结果');
% 定义阈值获取函数
function threshold_value = threshold(image)
[counts, binLocations] = imhist(image);
[~, max_index] = max(counts);
[~, second_max_index] = max(counts(1:max_index-1));
threshold_value = binLocations(second_max_index);
end
```
上述代码中,我们使用 `imread` 函数读入三幅示例图片,然后分别对它们进行处理。其中,我们定义了一个名为 `threshold` 的函数来获取阈值,并使用 `imhist` 函数来绘制直方图。在获取阈值时,我们找到直方图中的两个峰值,并将第二个峰值所在的位置作为分割阈值。最后,我们使用 `> ` 运算符将像素值大于阈值的像素设置为白色,像素值小于等于阈值的像素设置为黑色,从而得到分割结果。
建议你将上述代码保存为一个 `.m` 文件,并在 Matlab 中运行它,以便查看分割结果。
阅读全文
相关推荐
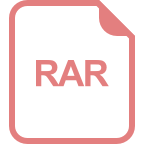
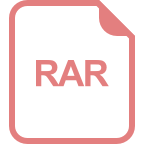
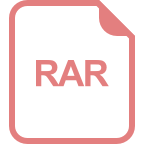
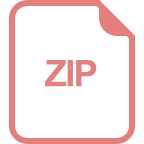
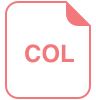
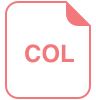










