# Count plots for binary variables for col in ['hypertension', 'heart_disease', 'diabetes']: sns.countplot(x=col, data=df) for i in range(len(df[col].value_counts().index)): plt.text(i, df[col].value_counts()[i], df[col].value_counts()[i], ha='center', va='bottom') plt.title(f'{col} Distribution') plt.show()
时间: 2024-02-07 19:04:20 浏览: 138
这段代码使用 Seaborn 和 Matplotlib 库创建了三个计数图,用于显示糖尿病数据集中的高血压、心脏病和糖尿病的分布情况。使用一个 `for` 循环来遍历这三个二元变量,其中 `col` 变量用于存储当前循环中的变量。在循环中,使用 `sns.countplot` 函数创建计数图,其中 `x=col` 表示按当前变量进行计数并绘制条形图,而 `data=df` 表示使用 `df` 数据帧中的数据。接下来,使用 `for` 循环和 `plt.text` 函数,在每个条形图的顶部添加计数值。最后,使用 `plt.title` 函数添加标题,使用 `plt.show` 函数显示图像。
相关问题
用python结合tkinter库实现def standard_X(X): X_copy = X.copy() # 拿数据 for col_name in X_copy.columns: # 取列名 col_data = X_copy[[col_name]] # 根据列名拿列数据,两个方括号是因为要二维数组 # fit_transform stand_data = StandardScaler().fit_transform(col_data.values) # 标准化 X_copy[col_name] = stand_data # 将数据替换成标准化后的数据 return X_copy standard_X(X).describe([0.01,0.25,0.5,0.75,0.99]).T stand_X = standard_X(X) for col_name in stand_X.columns: sns.distplot(stand_X[col_name]) plt.title(col_name) plt.show() X[0] = pd.cut(X[0],bins = 5,labels = [0,1,2,3,4]) sns.countplot(X[0]) for col_name in X.columns: X[col_name] = pd.cut(X[col_name],bins = 5,label knn = KNeighborsClassifier() X_train,X_test,y_train,y_test = train_test_split(X,y,test_size = 0.2,random_state = 1) knn.fit(X_train,y_train) KNeighborsClassifier() knn.score(X_train,y_train) knn.score(X_test,y_test)成一个界面
好的,下面是一个简单的示例,展示了如何使用Tkinter创建一个界面,其中包含您的代码。请注意,由于您未提供数据和标签,我将使用随机数据进行演示。您需要根据自己的需求调整代码。
```
import tkinter as tk
from tkinter import ttk
import pandas as pd
import numpy as np
import seaborn as sns
import matplotlib.pyplot as plt
from sklearn.preprocessing import StandardScaler
from sklearn.neighbors import KNeighborsClassifier
from sklearn.model_selection import train_test_split
class MyGUI:
def __init__(self, master):
self.master = master
self.master.title("Data Standardization and Classification")
# Create labels and entry boxes for user input
self.label_1 = ttk.Label(self.master, text="Enter number of rows:")
self.label_1.grid(row=0, column=0, padx=10, pady=10)
self.entry_1 = ttk.Entry(self.master)
self.entry_1.grid(row=0, column=1, padx=10, pady=10)
self.label_2 = ttk.Label(self.master, text="Enter number of columns:")
self.label_2.grid(row=1, column=0, padx=10, pady=10)
self.entry_2 = ttk.Entry(self.master)
self.entry_2.grid(row=1, column=1, padx=10, pady=10)
self.button_1 = ttk.Button(self.master, text="Submit", command=self.submit)
self.button_1.grid(row=2, column=1, padx=10, pady=10)
# Create output text box
self.output = tk.Text(self.master, height=10, width=50)
self.output.grid(row=3, column=0, columnspan=2, padx=10, pady=10)
def submit(self):
# Get user input
num_rows = int(self.entry_1.get())
num_cols = int(self.entry_2.get())
# Generate random data
X = pd.DataFrame(np.random.randn(num_rows, num_cols))
# Standardize data
X_copy = X.copy()
for col_name in X_copy.columns:
col_data = X_copy[[col_name]]
stand_data = StandardScaler().fit_transform(col_data.values)
X_copy[col_name] = stand_data
output_1 = X_copy.describe([0.01, 0.25, 0.5, 0.75, 0.99]).T
# Display distribution plots
for col_name in X_copy.columns:
sns.distplot(X_copy[col_name])
plt.title(col_name)
plt.show()
# Categorize data and display count plot
X[0] = pd.cut(X[0], bins=5, labels=[0, 1, 2, 3, 4])
sns.countplot(X[0])
plt.show()
# Classify data using KNN
knn = KNeighborsClassifier()
X_train, X_test, y_train, y_test = train_test_split(X, y, test_size=0.2, random_state=1)
knn.fit(X_train, y_train)
output_2 = "Train score: {:.2f}\nTest score: {:.2f}".format(knn.score(X_train, y_train), knn.score(X_test, y_test))
# Display output
self.output.delete('1.0', tk.END)
self.output.insert(tk.END, "Data Description:\n{}\n\n".format(output_1))
self.output.insert(tk.END, "KNN Classification:\n{}".format(output_2))
root = tk.Tk()
my_gui = MyGUI(root)
root.mainloop()
```
在这个界面中,用户需要输入他们想要的随机数据的行数和列数,并单击“提交”按钮。然后,程序将生成数据,对其进行标准化,显示分布图,将其分类并显示计数图,并在最后使用KNN对其进行分类。程序将输出数据描述和分类结果到文本框中。
# Look through unique values in each categorical column categorical_cols = train_df.select_dtypes(include="object").columns.tolist() for col in categorical_cols: print(f"{col}", f"Number of unique entries: {len(train_df[col].unique().tolist())},") print(train_df[col].unique().tolist()) def plot_bar_chart(df, columns, grid_rows, grid_cols, x_label='', y_label='', title='', whole_numbers_only=False, count_labels=True, as_percentage=True): num_plots = len(columns) grid_size = grid_rows * grid_cols num_rows = math.ceil(num_plots / grid_cols) if num_plots == 1: fig, axes = plt.subplots(1, 1, figsize=(12, 8)) axes = [axes] # Wrap the single axes in a list for consistent handling else: fig, axes = plt.subplots(num_rows, grid_cols, figsize=(12, 8)) axes = axes.ravel() # Flatten the axes array to iterate over it for i, column in enumerate(columns): df_column = df[column] if whole_numbers_only: df_column = df_column[df_column % 1 == 0] ax = axes[i] y = [num for (s, num) in df_column.value_counts().items()] x = [s for (s, num) in df_column.value_counts().items()] ax.bar(x, y, color='blue', alpha=0.5) try: ax.set_xticks(range(x[-1], x[0] + 1)) except: pass ax.set_xlabel(x_label) ax.set_ylabel(y_label) ax.set_title(title + ' - ' + column) if count_labels: df_col = df_column.value_counts(normalize=True).mul(100).round(1).astype(str) + '%' for idx, (year, value) in enumerate(df_column.value_counts().items()): if as_percentage == False: ax.annotate(f'{value}\n', xy=(year, value), ha='center', va='center') else: ax.annotate(f'{df_col[year]}\n', xy=(year, value), ha='center', va='center', size=8) if num_plots < grid_size: for j in range(num_plots, grid_size): fig.delaxes(axes[j]) # Remove empty subplots if present plt.tight_layout() plt.show()
这段代码定义了一个名为plot_bar_chart的函数,它可以绘制柱状图。函数的输入包括一个数据框(df)、一个列名的列表(columns)、网格的行数和列数(grid_rows和grid_cols)、x轴和y轴标签(x_label和y_label)、标题(title)、是否只显示整数(whole_numbers_only)、是否在图上显示数据标签(count_labels)、以及是否以百分比形式显示数据标签(as_percentage)。
在函数内部,它首先计算需要绘制的子图的数量,然后根据网格的行数和列数创建子图。接下来,对于每个给定的列名,函数从数据框中选择该列,并将其存储在变量df_column中。如果whole_numbers_only为True,则函数只绘制整数值。接着,函数将x轴和y轴的值分别设置为列中每个唯一值的出现次数和值本身。最后,函数在每个子图上绘制柱状图,并将数据标签添加到每个条形上。如果没有足够的子图来填充整个网格,则函数会删除空的子图以使图像更清晰。
阅读全文
相关推荐
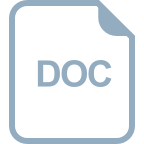
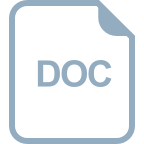
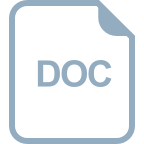













