class SelfAttention(Layer): def __init__(self, output_dim, **kwargs): self.output_dim = output_dim super(SelfAttention, self).__init__(**kwargs) def build(self, input_shape): self.W = self.add_weight(name='W', shape=(input_shape[-1], self.output_dim), initializer='uniform', trainable=True) self.b = self.add_weight(name='b', shape=(self.output_dim,), initializer='zeros', trainable=True) self.u = self.add_weight(name='u', shape=(self.output_dim, 1), initializer='uniform', trainable=True) super(SelfAttention, self).build(input_shape) def call(self, x): uit = K.tanh(K.bias_add(K.dot(x, self.W), self.b)) ait = K.softmax(K.squeeze(K.dot(uit, self.u), axis=-1)) weighted_input = x * K.expand_dims(ait) return K.sum(weighted_input, axis=1) def compute_output_shape(self, input_shape): return (input_shape[0], self.output_dim) def LSTNetAttention(trainX1,trainX2,trainY,config): # 输入数据 input1 = Input(shape=(trainX1.shape[1], trainX1.shape[2])) # 定义输入层 # 定义attention权重 # Add LSTM layer lstm1 = LSTM(64, return_sequences=True)(input1) # Add Self-Attention layer Self_Attention1 = SelfAttention(64)(lstm1) # 应用注意力机制到第二个输入 # Input2: long-term time series with period #input2 = Input(shape=(trainX2.shape[1], trainX2.shape[2],)) input2 = Input(shape=(trainX2.shape[1], trainX2.shape[2])) # Add LSTM layer lstm2 = LSTM(64, return_sequences=True)(input2) # Add Self-Attention layer Self_Attention2 = SelfAttention(64)(lstm2) merged_output = concatenate([Self_Attention1,Self_Attention2]) lstm_out = LSTM(64, return_sequences=False)(merged_output) # 加入LSTM层 lstm_out = Dense(32, activation='relu')(lstm_out) res = Dense(trainY.shape[1])(lstm_out)
时间: 2023-11-11 18:06:42 浏览: 236
这段代码定义了一个带有两个输入的 LSTNet 模型,并在其中添加了自注意力机制。其中,第一个输入是一个时间序列数据,经过 LSTM 后进行自注意力机制处理;第二个输入是一个长期时间序列数据,也经过 LSTM 后进行自注意力机制处理;最后将两个自注意力得到的输出合并,再经过一层 LSTM 和一层全连接层,最终输出模型预测结果。
具体来说,`SelfAttention` 类定义了一个自注意力机制,其核心计算在 `call` 方法中实现。输入 `x` 经过一层全连接层 `W` 和偏置项 `b` 后,再通过 tanh 激活函数。之后,将结果与一个可学习的权重向量 `u` 进行点积操作,得到注意力分布 `ait`。最后,将输入 `x` 与注意力分布相乘得到加权后的输入,并对加权后的输入进行求和,得到自注意力机制的输出。
在 `LSTNetAttention` 函数中,首先分别定义了两个输入层 `input1` 和 `input2`,并对它们分别应用 LSTM 和自注意力机制。然后将两个自注意力机制得到的输出合并,再经过一层 LSTM 和一层全连接层,最终输出模型预测结果。
相关问题
# 定义权重层 class WeightLayer(Layer): def __init__(self, output_dim, **kwargs): self.output_dim = output_dim super(WeightLayer, self).__init__(**kwargs) def build(self, input_shape): self.kernel = self.add_weight(name='kernel', shape=(input_shape[1], self.output_dim), initializer='uniform', trainable=True) super(WeightLayer, self).build(input_shape) def call(self, x): return x * self.kernel def compute_output_shape(self, input_shape): return (input_shape[0], self.output_dim)?
这段代码定义了一个权重层 WeightLayer,它继承了 Keras 的 Layer 类。这个层的作用是将输入 x 与自己的 kernel(权重矩阵)相乘,并返回结果。
在构造函数中,我们传入了 output_dim 参数,代表了输出的维度。在 build 方法中,我们根据输入的形状定义了一个名为 kernel 的权重矩阵,它的形状是 (input_shape[1], self.output_dim),其中 input_shape[1] 表示输入的第二个维度,因为第一个维度是样本数,我们不需要在权重层中处理。
在 call 方法中,我们将输入 x 乘以 kernel,得到输出。最后,我们重载了 compute_output_shape 方法,返回了输出的形状。
from keras import backend as K from keras.engine.topology import Layer class MyLayer(Layer): def __init__(self, output_dim, **kwargs): self.output_dim = output_dim super(MyLayer, self).__init__(**kwargs) def build(self, input_shape): # 为该层创建一个可训练的权重 self.kernel = self.add_weight(name='kernel', shape=(input_shape[1], self.output_dim), initializer='uniform', trainable=True) super(MyLayer, self).build(input_shape) # 一定要在最后调用它 def call(self, x): return K.dot(x, self.kernel) def compute_output_shape(self, input_shape): return (input_shape[0], self.output_dim)
这是一个使用Keras实现自定义层的代码,具体来说,定义了一个名为MyLayer的层,该层的功能是将输入x与可训练的权重kernel做矩阵乘法,并输出结果。其中,input_shape[1]表示输入x的第二个维度的大小,即输入的特征维度,self.output_dim表示输出的特征维度,initializer='uniform'表示权重的初始化方式为均匀分布。最后,compute_output_shape方法用于计算该层的输出形状。
阅读全文
相关推荐
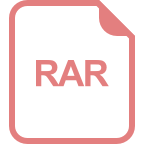
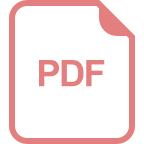
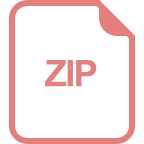













