爬取https://nba.hupu.com/stats/players网址中的所有球队的比赛数据,并使用分布雷达可视化图表将其呈现。
时间: 2023-12-02 15:03:58 浏览: 66
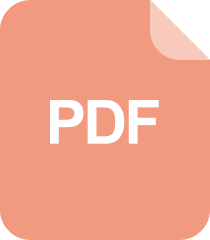
python爬虫系列Selenium定向爬取虎扑篮球图片详解
好的,我可以为您提供一些指导。
首先,您需要使用 Python 爬虫库(如 requests、beautifulsoup4 或 scrapy)获取 https://nba.hupu.com/stats/players 网站上的数据。您可以使用 requests 库向该网站发送 GET 请求,然后使用 beautifulsoup4 库解析 HTML 页面。
然后,您需要将数据存储在 Pandas DataFrame 中。使用 Pandas 库,您可以轻松地将数据从 HTML 表格中提取出来,然后将其转换为 DataFrame。
接下来,您需要使用 Matplotlib 和 Seaborn 库来创建分布雷达可视化图表。这种类型的图表通常用于显示多个变量之间的关系。您可以使用 Matplotlib 的 polar 函数来绘制极坐标轴,然后使用 Seaborn 的 radar_chart 函数来绘制雷达图。
最后,您需要将生成的可视化图表保存为图像文件或将其显示在屏幕上。使用 Matplotlib 的 savefig 函数可以将图表保存为图像文件,而 show 函数可以将其显示在屏幕上。
需要注意的是,如果您打算将此代码用于商业目的,请确保遵守相关法律法规,包括但不限于隐私政策和数据使用规定。
阅读全文
相关推荐
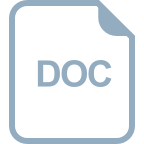
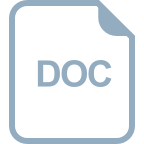
















