dtype=np.long numpy没有np.long,怎么办?
时间: 2023-10-11 22:09:33 浏览: 66
对于 NumPy 来说,没有 `np.long` 这种数据类型。如果你需要使用 64 位整数类型,可以使用 `np.int64`。所以你可以将代码中的 `dtype=np.long` 替换为 `dtype=np.int64`,例如:
```python
audio = np.frombuffer(b''.join(frames), dtype=np.int64)
```
这样应该就可以解决数据类型不匹配的问题了。
相关问题
import torch import os import torch.nn as nn import torch.optim as optim import numpy as np import random class Net(nn.Module): def init(self): super(Net, self).init() self.conv1 = nn.Conv2d(1, 16, kernel_size=3,stride=1) self.pool = nn.MaxPool2d(kernel_size=2,stride=2) self.conv2 = nn.Conv2d(16, 32, kernel_size=3,stride=1) self.fc1 = nn.Linear(32 * 9 * 9, 120) self.fc2 = nn.Linear(120, 84) self.fc3 = nn.Linear(84, 2) def forward(self, x): x = self.pool(nn.functional.relu(self.conv1(x))) x = self.pool(nn.functional.relu(self.conv2(x))) x = x.view(-1, 32 * 9 * 9) x = nn.functional.relu(self.fc1(x)) x = nn.functional.relu(self.fc2(x)) x = self.fc3(x) return x net = Net() criterion = nn.CrossEntropyLoss() optimizer = optim.SGD(net.parameters(), lr=0.001, momentum=0.9) folder_path = 'random_matrices2' # 创建空的tensor x = torch.empty((40, 1, 42, 42)) # 遍历文件夹内的文件,将每个矩阵转化为tensor并存储 for j in range(40): for j in range(40): file_name = 'matrix_{}.npy'.format(j) file_path = os.path.join(folder_path, file_name) matrix = np.load(file_path) x[j] = torch.from_numpy(matrix).unsqueeze(0) #y = torch.cat((torch.zeros(20), torch.ones(20))) #y = torch.cat((torch.zeros(20, dtype=torch.long), torch.ones(20, dtype=torch.long))) y = torch.cat((torch.zeros(20, dtype=torch.long), torch.ones(20, dtype=torch.long)), dim=0) for epoch in range(10): running_loss = 0.0 for i in range(40): inputs = x[i] labels = y[i].unsqueeze(0) labels = labels.long() optimizer.zero_grad() outputs = net(inputs) #loss = criterion(outputs, labels) loss = criterion(outputs.unsqueeze(0), labels.float()) loss.backward() optimizer.step() running_loss += loss.item() print('[%d] loss: %.3f' % (epoch + 1, running_loss / 40)) print('Finished Training') 报错:RuntimeError: Expected target size [1, 2], got [1],怎么修改?
这个错误是因为标签labels的形状不正确。在你的代码中,你使用了`labels = y[i].unsqueeze(0)`来定义标签,但这会将标签的形状从`(20,)`转换为`(1,20)`。因此,你需要使用`labels = y[i]`来定义标签,这样标签的形状将是`(1,)`,然后在计算损失时,使用`loss = criterion(outputs.unsqueeze(0), labels)`即可。修改后的代码如下:
```
import torch
import os
import torch.nn as nn
import torch.optim as optim
import numpy as np
import random
class Net(nn.Module):
def __init__(self):
super(Net, self).__init__()
self.conv1 = nn.Conv2d(1, 16, kernel_size=3,stride=1)
self.pool = nn.MaxPool2d(kernel_size=2,stride=2)
self.conv2 = nn.Conv2d(16, 32, kernel_size=3,stride=1)
self.fc1 = nn.Linear(32 * 9 * 9, 120)
self.fc2 = nn.Linear(120, 84)
self.fc3 = nn.Linear(84, 2)
def forward(self, x):
x = self.pool(nn.functional.relu(self.conv1(x)))
x = self.pool(nn.functional.relu(self.conv2(x)))
x = x.view(-1, 32 * 9 * 9)
x = nn.functional.relu(self.fc1(x))
x = nn.functional.relu(self.fc2(x))
x = self.fc3(x)
return x
net = Net()
criterion = nn.CrossEntropyLoss()
optimizer = optim.SGD(net.parameters(), lr=0.001, momentum=0.9)
folder_path = 'random_matrices2'
# 创建空的tensor
x = torch.empty((40, 1, 42, 42))
# 遍历文件夹内的文件,将每个矩阵转化为tensor并存储
for j in range(40):
file_name = 'matrix_{}.npy'.format(j)
file_path = os.path.join(folder_path, file_name)
matrix = np.load(file_path)
x[j] = torch.from_numpy(matrix).unsqueeze(0)
y = torch.cat((torch.zeros(20, dtype=torch.long), torch.ones(20, dtype=torch.long)), dim=0)
for epoch in range(10):
running_loss = 0.0
for i in range(40):
inputs = x[i]
labels = y[i]
optimizer.zero_grad()
outputs = net(inputs)
loss = criterion(outputs.unsqueeze(0), labels)
loss.backward()
optimizer.step()
running_loss += loss.item()
print('[%d] loss: %.3f' % (epoch + 1, running_loss / 40))
print('Finished Training')
```
希望能够帮助到你。
def __getitem__(self, idx): i = np.random.randint(0, len(self.data) - (self.ctx_len + 1)) # cheat: pick a random spot in dataset chunk = self.data[i:i+self.ctx_len+1] dix = [self.stoi[s] for s in chunk] x = torch.tensor(dix[:-1], dtype=torch.long) y = torch.tensor(dix[1:], dtype=torch.long) return x, y
这段代码是`Dataset`类的`__getitem__`方法。该方法用于实现索引操作,通过索引获取数据集中的一个样本。
首先,代码使用`np.random.randint(0, len(self.data) - (self.ctx_len + 1))`随机生成一个索引`i`,该索引用于选择数据集中的一个随机位置作为样本的起始位置。这里使用了`np.random.randint`函数从0到`(self.ctx_len + 1)`之间生成一个随机整数,用于确定样本的起始位置。
然后,代码从数据集中选取从起始位置`i`到`(i+self.ctx_len+1)`之间的一段数据作为样本的片段,存储在变量`chunk`中。
接下来,代码使用`self.stoi[s]`将`chunk`中的每个单词映射为对应的索引,并将结果存储在列表`dix`中。
然后,代码将列表`dix[:-1]`转换为一个PyTorch张量,并将其命名为`x`。这里使用了切片操作`[:-1]`来获取除最后一个元素之外的所有元素。
代码接着将列表`dix[1:]`转换为另一个PyTorch张量,并将其命名为`y`。这里使用了切片操作`[1:]`来获取除第一个元素之外的所有元素。
最后,代码返回张量`x`和张量`y`作为样本的输入和目标。
注意,这段代码还使用了`torch`和`np`模块,但是没有在代码中导入这些模块,所以你可能需要在代码开头添加以下导入语句:
```python
import torch
import numpy as np
```
相关推荐
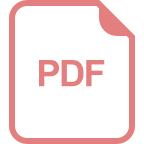
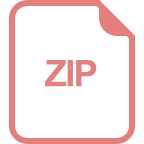











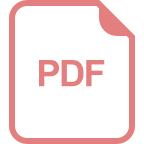