y = file.split(os.sep)[-1].split( . )[0]为什么要这段代码
时间: 2024-06-06 18:10:20 浏览: 11
这段代码的作用是获取文件名的字符串,其中 os.sep 代表不同操作系统的文件路径分隔符,[-1] 表示从后往前取最后一个元素,再用 split 方法将字符串按照 "." 进行分割,取分割后的第一个元素作为文件名(不包括扩展名),赋值给变量 y。这样做的目的是方便后续的文件处理操作、文件命名规则等。
相关问题
y = file.split(os.sep)[-1].split('.')[0]是什么意思
这是一个Python代码,它的作用是将文件名拆分成几部分,并将文件名的第一部分赋值给变量y。具体来说,它将文件路径字符串分隔符os.sep作为分隔符,将文件名分成几部分,然后使用点号作为分隔符,将文件名的第一个部分提取出来赋值给变量y。
使用 pathlib 替换操作系统路径(os) file_suffix = sweep['lidar_points']['lidar_path'].split( os.sep)[-1]
可以使用pathlib的name属性来获取路径中的文件名,可以替换掉使用os模块的split和索引操作。
示例代码如下:
```
from pathlib import Path
file_suffix = Path(sweep['lidar_points']['lidar_path']).name
```
这里,我们将路径字符串转换为Path对象,并使用name属性获取文件名。这种方法更加简洁和可读性更高。
相关推荐
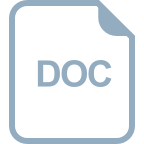
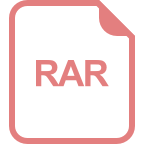
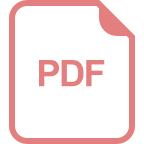












