dy/dx+(x+(1+3x²)/(1+x+x³))*y=x³+2x+x²*(1+3x²)/(1+x+x³) y(0)=1
时间: 2023-07-02 22:16:15 浏览: 94
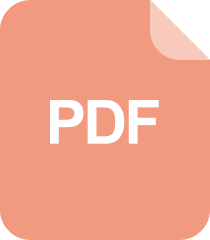
数学分析3-3(数学类)--期末考试--2015级1
可以使用深度学习框架进行神经网络的构建和训练,这里以PyTorch为例。
首先,定义神经网络模型:
```python
import torch
import torch.nn as nn
import torch.optim as optim
class Net(nn.Module):
def __init__(self):
super(Net, self).__init__()
self.linear1 = nn.Linear(2, 10) # 输入层到隐层
self.linear2 = nn.Linear(10, 1) # 隐层到输出层
def forward(self, x):
x = torch.sigmoid(self.linear1(x))
x = self.linear2(x)
return x
```
接下来,定义训练函数:
```python
def train(net, criterion, optimizer, x_train, y_train):
net.train()
running_loss = 0.0
for i in range(len(x_train)):
x = x_train[i]
y = y_train[i]
optimizer.zero_grad()
outputs = net(x)
loss = criterion(outputs, y)
loss.backward()
optimizer.step()
running_loss += loss.item()
return running_loss / len(x_train)
```
然后,定义测试函数:
```python
def test(net, criterion, x_test, y_test):
net.eval()
running_loss = 0.0
with torch.no_grad():
for i in range(len(x_test)):
x = x_test[i]
y = y_test[i]
outputs = net(x)
loss = criterion(outputs, y)
running_loss += loss.item()
return running_loss / len(x_test)
```
接下来,进行数据处理,将微分方程转化为数据集:
```python
import numpy as np
# 定义微分方程
def f(x, y):
return x ** 3 + 2 * x + x ** 2 * (1 + 3 * x ** 2) / (1 + x + x ** 3) - (x + (1 + 3 * x ** 2) / (1 + x + x ** 3)) * y
# 定义数据集
x_train = np.linspace(0, 1, 100)
y_train = np.zeros((100, 1))
y_train[0] = 1
for i in range(1, len(x_train)):
h = x_train[i] - x_train[i - 1]
k1 = f(x_train[i - 1], y_train[i - 1])
k2 = f(x_train[i - 1] + h / 2, y_train[i - 1] + h / 2 * k1)
k3 = f(x_train[i - 1] + h / 2, y_train[i - 1] + h / 2 * k2)
k4 = f(x_train[i], y_train[i - 1] + h * k3)
y_train[i] = y_train[i - 1] + h / 6 * (k1 + 2 * k2 + 2 * k3 + k4)
```
最后,进行神经网络的训练和测试:
```python
# 初始化神经网络模型、损失函数和优化器
net = Net()
criterion = nn.MSELoss()
optimizer = optim.Adam(net.parameters(), lr=0.01)
# 进行训练和测试
for epoch in range(1000):
train_loss = train(net, criterion, optimizer, x_train, y_train)
test_loss = test(net, criterion, x_train, y_train)
if epoch % 100 == 0:
print('Epoch: {}, Train Loss: {:.6f}, Test Loss: {:.6f}'.format(epoch+1, train_loss, test_loss))
```
最终,可以得到训练好的神经网络模型,将新的输入数据输入到模型中,即可得到相应的输出。
阅读全文
相关推荐
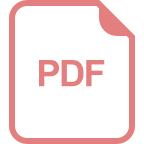
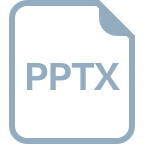





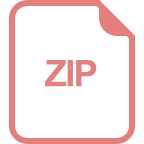
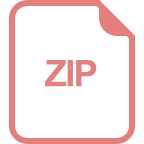
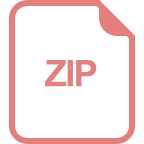
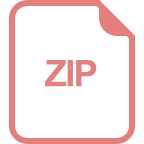
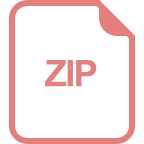
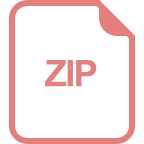
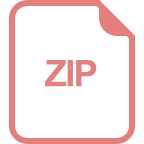
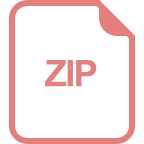