if __name__ == "__main__": dataset_dir = Path(os.getenv("DETECTRON2_DATASETS", "datasets")) print('Caution: we only generate the training set!') coco_path = dataset_dir / "coco" mask_dir = coco_path / "stuffthingmaps" out_mask_dir = coco_path / "stuffthingmaps_detectron2" for name in ["train2017"]: os.makedirs((out_mask_dir / name), exist_ok=True) train_list = glob(osp.join(mask_dir, "train2017", "*.png")) for file in tqdm.tqdm(train_list): convert_to_trainID(file, out_mask_dir, is_train=True)
时间: 2024-03-07 22:52:54 浏览: 22
这段代码的作用是将 COCO 数据集中的实例分割标注图转换成 Detectron2 模型训练所需的标注格式,并保存到指定的文件夹中。
具体来说,代码首先获取 COCO 数据集所在的路径 `dataset_dir`,并在其中找到实例分割标注图所在的文件夹 `mask_dir`。然后代码创建一个新的文件夹 `out_mask_dir`,用于存放转换后的标注图。接着,代码遍历 COCO 数据集中的训练集 `train2017`,并逐一读取其中的实例分割标注图,调用 `convert_to_trainID` 函数将其转换成 Detectron2 模型训练所需的标注格式,并保存到 `out_mask_dir` 文件夹中。最后,代码通过 `tqdm` 库实现了进度条的显示。
需要注意的是,这段代码只会处理 COCO 数据集中的训练集,而不是验证集或测试集。并且,该转换只适用于使用 Detectron2 进行模型训练时需要的标注格式,与其他深度学习框架或任务可能不兼容。
相关问题
self.dir = os.listdir(self.dataset_path)
这行代码是用于获取指定路径下的所有文件和文件夹的列表。其中,`self` 是一个对象,`dir` 是该对象的一个属性。`os.listdir()` 是一个函数,它接受一个路径作为参数,并返回该路径下所有文件和文件夹的列表。在这个例子中,`self.dataset_path` 是一个路径字符串,它指定了要获取列表的目录。通过调用 `os.listdir(self.dataset_path)`,将返回一个包含目录下所有文件和文件夹的列表,并将其赋值给 `self.dir` 属性。
dataset_dir = os.path.join(args.data_dir, args.dataset)
这行代码的作用是将命令行参数 args.data_dir 和 args.dataset 合并为一个完整的数据集目录路径,存储在变量 dataset_dir 中。假设 args.data_dir 是 "/home/user/data",args.dataset 是 "mydataset",那么最终的 dataset_dir 值就是 "/home/user/data/mydataset"。这个目录是用来存放数据集文件的。
相关推荐
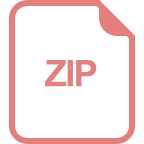













