i for i in samples if i is not None
时间: 2024-05-16 22:12:00 浏览: 27
As an AI language model, I understand that the code snippet you provided is written in Python. This is a list comprehension that filters out any 'None' values from the 'samples' list and returns a new list with only the non-None elements. Here is an example:
```
samples = [1, 2, None, 4, None, 5]
new_samples = [i for i in samples if i is not None]
print(new_samples)
```
Output:
```
[1, 2, 4, 5]
```
In this example, the original 'samples' list contains two 'None' values. The list comprehension filters them out and creates a new list 'new_samples' with only the non-None elements. The output of the code is '[1, 2, 4, 5]'.
相关问题
Compute the loss and gradients for a two layer fully connected neural network. Inputs: - X: Input data of shape (N, D). Each X[i] is a training sample. - y: Vector of training labels. y[i] is the label for X[i], and each y[i] is an integer in the range 0 <= y[i] < C. This parameter is optional; if it is not passed then we only return scores, and if it is passed then we instead return the loss and gradients. - reg: Regularization strength. Returns: If y is None, return a matrix scores of shape (N, C) where scores[i, c] is the score for class c on input X[i]. If y is not None, instead return a tuple of: - loss: Loss (data loss and regularization loss) for this batch of training samples. - grads: Dictionary mapping parameter names to gradients of those parameters with respect to the loss function; has the same keys as self.params.
To compute the loss and gradients for a two layer fully connected neural network, we need to perform forward and backward propagation.
Forward propagation:
1. Compute the scores for each class by multiplying the input data X with the weight matrix W1 and adding the bias term b1. Then apply ReLU activation function to the result.
2. Compute the scores for each class by multiplying the output of the first layer with the weight matrix W2 and adding the bias term b2.
The loss function for a multi-class classification problem is usually the cross-entropy loss.
Backward propagation:
1. Compute the gradient of the loss with respect to the scores of the second layer.
2. Compute the gradient of the loss with respect to the parameters of the second layer (W2 and b2).
3. Compute the gradient of the loss with respect to the output of the first layer.
4. Compute the gradient of the loss with respect to the scores of the first layer (taking into account the ReLU activation function).
5. Compute the gradient of the loss with respect to the parameters of the first layer (W1 and b1).
Finally, we add the regularization term to the loss and compute the gradients with respect to the regularization term as well.
Here's the code:
```python
def two_layer_fc(X, params, reg=0.0):
W1, b1, W2, b2 = params['W1'], params['b1'], params['W2'], params['b2']
N, D = X.shape
scores = None
# Forward pass
hidden_layer = np.maximum(0, np.dot(X, W1) + b1) # ReLU activation
scores = np.dot(hidden_layer, W2) + b2
# If y is not given, return scores
if y is None:
return scores
# Compute the loss and gradients
loss = None
grads = {}
# Compute the loss (data loss and regularization loss)
num_classes = W2.shape[1]
exp_scores = np.exp(scores)
probs = exp_scores / np.sum(exp_scores, axis=1, keepdims=True)
correct_logprobs = -np.log(probs[range(N),y])
data_loss = np.sum(correct_logprobs) / N
reg_loss = 0.5 * reg * (np.sum(W1*W1) + np.sum(W2*W2))
loss = data_loss + reg_loss
# Compute the gradients
dscores = probs
dscores[range(N),y] -= 1
dscores /= N
dW2 = np.dot(hidden_layer.T, dscores)
db2 = np.sum(dscores, axis=0, keepdims=True)
dhidden = np.dot(dscores, W2.T)
dhidden[hidden_layer <= 0] = 0
dW1 = np.dot(X.T, dhidden)
db1 = np.sum(dhidden, axis=0, keepdims=True)
# Add regularization gradient contribution
dW2 += reg * W2
dW1 += reg * W1
# Store gradients in dictionary
grads['W1'] = dW1
grads['b1'] = db1
grads['W2'] = dW2
grads['b2'] = db2
return loss, grads
```
怎么删除,不知道具体哪些图片is None\
可以在 `__init__` 方法中先将所有图片的路径和标签存储到 `self.samples` 中,然后在 `__getitem__` 方法中根据索引读取对应的图片和标签。如果图片加载失败,可以将该样本的索引保存到一个列表中,最后再从 `self.samples` 中删除这些索引对应的样本。具体的实现代码如下:
```python
class FlowerDataset(Dataset):
def __init__(self, filenames, labels, transform):
self.filenames = filenames
self.labels = labels
self.transform = transform
self.samples = [(filename, label) for filename, label in zip(filenames, labels)]
def __len__(self):
return len(self.samples)
def __getitem__(self, idx):
filename, label = self.samples[idx]
image = None
if os.path.exists(filename):
image = Image.open(filename).convert('RGB')
if image is None:
print(f"图片 {filename} 加载失败")
return None
image = self.transform(image)
return image, label
def clean_samples(self):
failed_indices = []
for idx, (filename, label) in enumerate(self.samples):
if not os.path.exists(filename):
print(f"图片 {filename} 不存在")
failed_indices.append(idx)
else:
try:
Image.open(filename)
except:
print(f"图片 {filename} 加载失败")
failed_indices.append(idx)
self.samples = [sample for idx, sample in enumerate(self.samples) if idx not in failed_indices]
```
在这个实现中,我们新增了一个 `clean_samples` 方法,用于从数据集中删除加载失败的样本。在 `clean_samples` 方法中,我们遍历了所有样本,如果某个样本的图片不存在或者加载失败,就将该样本的索引保存到 `failed_indices` 中。最后,我们通过列表推导式从 `self.samples` 中删除这些索引对应的样本。执行 `clean_samples` 方法后,所有加载失败的样本都会被从数据集中删除。
相关推荐
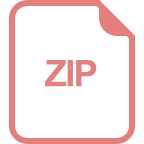
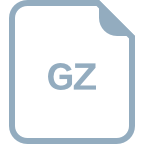
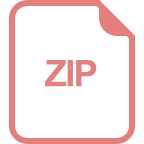











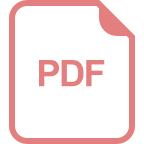