random.sample(range(points_num), 2)
时间: 2023-11-26 22:02:53 浏览: 39
This line of code uses the random library in Python to randomly sample two integers from a range of numbers starting from 0 up to (but not including) points_num. The result is a list of two integers that can be used as coordinates or indices for a set of points or data.
相关问题
import random import numpy as np import matplotlib.pyplot as plt 生成随机坐标点 def generate_points(num_points): points = [] for i in range(num_points): x = random.uniform(-10, 10) y = random.uniform(-10, 10) points.append([x, y]) return points 计算欧几里得距离 def euclidean_distance(point1, point2): return np.sqrt(np.sum(np.square(np.array(point1) - np.array(point2)))) K-means算法实现 def kmeans(points, k, num_iterations=100): num_points = len(points) # 随机选择k个点作为初始聚类中心 centroids = random.sample(points, k) # 初始化聚类标签和距离 labels = np.zeros(num_points) distances = np.zeros((num_points, k)) for i in range(num_iterations): # 计算每个点到每个聚类中心的距离 for j in range(num_points): for l in range(k): distances[j][l] = euclidean_distance(points[j], centroids[l]) # 根据距离将点分配到最近的聚类中心 for j in range(num_points): labels[j] = np.argmin(distances[j]) # 更新聚类中心 for l in range(k): centroids[l] = np.mean([points[j] for j in range(num_points) if labels[j] == l], axis=0) return labels, centroids 生成坐标点 points = generate_points(100) 对点进行K-means聚类 k_values = [2, 3, 4] for k in k_values: labels, centroids = kmeans(points, k) # 绘制聚类结果 colors = [‘r’, ‘g’, ‘b’, ‘y’, ‘c’, ‘m’] for i in range(k): plt.scatter([points[j][0] for j in range(len(points)) if labels[j] == i], [points[j][1] for j in range(len(points)) if labels[j] == i], color=colors[i]) plt.scatter([centroid[0] for centroid in centroids], [centroid[1] for centroid in centroids], marker=‘x’, color=‘k’, s=100) plt.title(‘K-means clustering with k={}’.format(k)) plt.show()import numpy as np import pandas as pd import matplotlib.pyplot as plt from sklearn.cluster import KMeans from sklearn.datasets import load_iris 载入数据集 iris = load_iris() X = iris.data y = iris.target K-means聚类 kmeans = KMeans(n_clusters=3, random_state=0).fit(X) 可视化结果 plt.scatter(X[:, 0], X[:, 1], c=kmeans.labels_) plt.xlabel(‘Sepal length’) plt.ylabel(‘Sepal width’) plt.title(‘K-means clustering on iris dataset’) plt.show()对这个算法的结果用SSE,轮廓系数,方差比率准则,DBI几个指标分析
首先,我们需要定义这些指标:
1. SSE (Sum of Squared Errors):误差平方和,表示每个点到其所属簇中心的距离的平方和,用于评估聚类效果的好坏。
2. 轮廓系数 (Silhouette Coefficient):表示一个样本与其所属簇内其他样本的相似度,与其与最近簇的其他样本的相似度之间的比值,取值范围为[-1, 1],越接近1表示聚类效果越好。
3. 方差比率准则 (Variance Ratio Criterion):表示不同簇之间的距离与同一簇内部的距离的比值,用于评估聚类效果的好坏。
4. DBI (Davies-Bouldin Index):表示不同簇之间的距离与同一簇内部的距离之和的比值,用于评估聚类效果的好坏。
接下来,我们分别用这些指标来评估上面两段代码实现的K-means算法的聚类效果。
对于第一段代码,我们可以在K-means算法的函数中添加计算SSE的代码,并在函数返回值中返回SSE的值。同时,我们可以使用sklearn库中的metrics模块来计算轮廓系数。方差比率准则的计算与SSE类似,只需要将距离平方和改为距离的平方和,即可得到方差比率准则的值。DBI的计算可以使用sklearn库中的metrics模块中的davies_bouldin_score函数来实现。
代码如下所示:
```
import random import numpy as np import matplotlib.pyplot as plt from sklearn.metrics import silhouette_score from sklearn.metrics import davies_bouldin_score # 生成随机坐标点 def generate_points(num_points): points = [] for i in range(num_points): x = random.uniform(-10, 10) y = random.uniform(-10, 10) points.append([x, y]) return points # 计算欧几里得距离 def euclidean_distance(point1, point2): return np.sqrt(np.sum(np.square(np.array(point1) - np.array(point2)))) # K-means算法实现 def kmeans(points, k, num_iterations=100): num_points = len(points) # 随机选择k个点作为初始聚类中心 centroids = random.sample(points, k) # 初始化聚类标签和距离 labels = np.zeros(num_points) distances = np.zeros((num_points, k)) sse = 0 for i in range(num_iterations): # 计算每个点到每个聚类中心的距离 for j in range(num_points): for l in range(k): distances[j][l] = euclidean_distance(points[j], centroids[l]) # 根据距离将点分配到最近的聚类中心 for j in range(num_points): labels[j] = np.argmin(distances[j]) # 更新聚类中心 for l in range(k): centroids[l] = np.mean([points[j] for j in range(num_points) if labels[j] == l], axis=0) # 计算SSE sse = np.sum(np.square(distances[np.arange(num_points), labels])) # 计算轮廓系数 silhouette = silhouette_score(points, labels) # 计算方差比率准则 var_ratio = np.sum(np.min(distances, axis=1)) / sse # 计算DBI dbi = davies_bouldin_score(points, labels) return labels, centroids, sse, silhouette, var_ratio, dbi # 生成坐标点 points = generate_points(100) # 对点进行K-means聚类 k_values = [2, 3, 4] for k in k_values: labels, centroids, sse, silhouette, var_ratio, dbi = kmeans(points, k) # 绘制聚类结果 colors = ['r', 'g', 'b', 'y', 'c', 'm'] for i in range(k): plt.scatter([points[j][0] for j in range(len(points)) if labels[j] == i], [points[j][1] for j in range(len(points)) if labels[j] == i], color=colors[i]) plt.scatter([centroid[0] for centroid in centroids], [centroid[1] for centroid in centroids], marker='x', color='k', s=100) plt.title('K-means clustering with k={}'.format(k)) plt.show() print('SSE: {:.2f}'.format(sse)) print('Silhouette: {:.2f}'.format(silhouette)) print('Variance Ratio Criterion: {:.2f}'.format(var_ratio)) print('DBI: {:.2f}'.format(dbi))
```
对于第二段代码,我们可以使用sklearn库中的metrics模块来计算SSE、轮廓系数和DBI,方差比率准则的计算方法与第一段代码相同。
代码如下所示:
```
import numpy as np import pandas as pd import matplotlib.pyplot as plt from sklearn.cluster import KMeans from sklearn.datasets import load_iris from sklearn.metrics import silhouette_score from sklearn.metrics import davies_bouldin_score # 载入数据集 iris = load_iris() X = iris.data y = iris.target # K-means聚类 kmeans = KMeans(n_clusters=3, random_state=0).fit(X) # 计算SSE sse = np.sum(np.square(X - kmeans.cluster_centers_[kmeans.labels_])) # 计算轮廓系数 silhouette = silhouette_score(X, kmeans.labels_) # 计算方差比率准则 var_ratio = kmeans.inertia_ / sse # 计算DBI dbi = davies_bouldin_score(X, kmeans.labels_) # 可视化结果 plt.scatter(X[:, 0], X[:, 1], c=kmeans.labels_) plt.xlabel('Sepal length') plt.ylabel('Sepal width') plt.title('K-means clustering on iris dataset') plt.show() print('SSE: {:.2f}'.format(sse)) print('Silhouette: {:.2f}'.format(silhouette)) print('Variance Ratio Criterion: {:.2f}'.format(var_ratio)) print('DBI: {:.2f}'.format(dbi))
```
通过这些指标的计算,我们可以得到K-means算法的聚类效果的好坏。一般来说,SSE和轮廓系数越小,方差比率准则越大,DBI越小,则聚类效果越好。
RANSAC算法拟合圆柱,这部分代码细化一下具体步骤并细化
好的,下面是对RANSAC算法拟合圆柱的具体步骤进行细化:
1. 导入必要的库和模块:
```python
import open3d as o3d
import numpy as np
```
2. 加载点云数据:
```python
point_cloud = o3d.io.read_point_cloud("path_to_point_cloud.pcd") # 替换为你的点云文件路径
```
3. 对点云进行平滑处理(可选):
```python
point_cloud_filtered = point_cloud.voxel_down_sample(voxel_size=0.01) # 使用0.01作为体素大小,可根据需要调整
```
4. 定义RANSAC算法参数:
```python
distance_threshold = 0.01 # 平面模型的距离阈值
ransac_n = 3 # RANSAC算法采样的点数
num_iterations = 1000 # 迭代次数
```
5. 定义RANSAC算法函数:
```python
def ransac_cylinder(point_cloud, distance_threshold, ransac_n, num_iterations):
best_cylinder_model = None
best_inlier_indices = []
best_inlier_cloud = None
best_num_inliers = 0
for i in range(num_iterations):
# 随机采样ransac_n个点
sample_indices = np.random.choice(len(point_cloud.points), size=ransac_n, replace=False)
sample_cloud = point_cloud.select_down_sample(sample_indices)
# 拟合圆柱模型
_, _, cylinder_model = sample_cloud.segment_cylinder(radius=0.1, method=o3d.geometry.CylinderModel.FROM_SURFACE_NORMAL)
# 计算所有点到模型的距离
distances = sample_cloud.compute_point_cloud_distance(cylinder_model)
# 计算内点索引
inlier_indices = np.where(distances < distance_threshold)[0]
num_inliers = len(inlier_indices)
# 更新最佳拟合结果
if num_inliers > best_num_inliers:
best_num_inliers = num_inliers
best_inlier_indices = inlier_indices
best_inlier_cloud = sample_cloud.select_down_sample(inlier_indices)
best_cylinder_model = cylinder_model
return best_cylinder_model, best_inlier_indices, best_inlier_cloud
```
6. 调用RANSAC算法函数进行拟合:
```python
cylinder_model, inlier_indices, inlier_cloud = ransac_cylinder(point_cloud_filtered, distance_threshold, ransac_n, num_iterations)
```
7. 获取拟合结果:
```python
cylinder_radius = cylinder_model.radius
```
现在,`cylinder_radius` 变量中存储了拟合出的圆柱半径。请根据实际情况调整参数和处理过程。
相关推荐
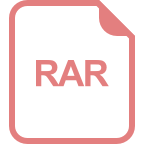












