train_data = ts[:len(ts)-24] test_data = ts[len(ts)-24:]啥意思
时间: 2023-10-27 21:49:30 浏览: 140
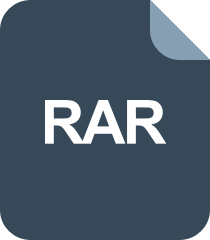
train data
这两行代码将时间序列数据ts分成了训练数据train_data和测试数据test_data。
训练数据train_data包含了ts的前len(ts)-24个时间步,即除去最后24个时间步的所有时间步数据,用于训练模型。
测试数据test_data包含了ts的最后24个时间步,用于评估模型的预测效果。
阅读全文
相关推荐







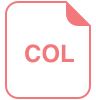
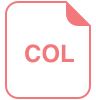
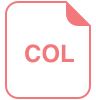
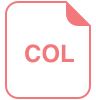
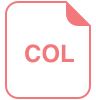
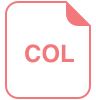
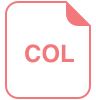
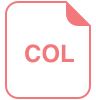
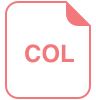