This code uses a modified Dijkstra algorithm where we keep track of the number of visited waypoints in addition to the distances. When we visit a new point that is one of the required waypoints, we increment the number of visited waypoints. We also add an additional condition to check if we have visited all 8 required waypoints before we break out of the loop. To reconstruct the path, we need to traverse the distances table in reverse, starting from the end point and the optimal number of visited waypoints, and finding the previous point in the optimal path with the same number of visited waypoints. Finally, we print the optimal path and the total distance.什么意思
时间: 2024-01-04 11:02:59 浏览: 36
这段代码使用了一种改进版的Dijkstra算法,不仅记录了距离,还记录了访问的航点数。当我们访问到一个新的航点是,如果它是必须要访问的航点之一,我们就会增加已访问航点数的计数器。同时,我们还添加了一个额外的条件来判断是否已经访问了所有8个必须要访问的航点,如果是,则跳出循环。为了重构路径,我们需要从终点和最优访问航点数开始,反向遍历距离表,并找到在同一访问航点数下的最优路径的前一个点。最后,我们输出最优路径和总距离。
相关问题
Use Dijkstra's algorithm to find the shortest paths from vertex a to other vertices. In which order that the results must be obtained?
The order of the results obtained from using Dijkstra's algorithm to find the shortest paths from vertex a to other vertices is not specified by the algorithm itself. However, typically the results are obtained in ascending order based on the distance from vertex a to each of the other vertices.
Help me write a literature review of the research on path planning algorithms for satellite maps
Satellite maps have become an essential tool for various applications, including navigation, agriculture, urban planning, and disaster response. One of the critical challenges in using satellite maps is path planning, which involves finding the optimal path between two locations while considering various constraints such as terrain, obstacles, and weather conditions. Over the years, several path planning algorithms have been developed for satellite maps, and this literature review aims to provide an overview of the research in this field.
One of the earliest and most popular path planning algorithms for satellite maps is Dijkstra's algorithm. This algorithm uses a graph-based approach to find the shortest path between two points while avoiding obstacles. However, Dijkstra's algorithm has limitations when dealing with large-scale maps or dynamic environments, and several variants have been proposed to overcome these limitations. For example, A* algorithm is an extension of Dijkstra's algorithm that uses heuristics to reduce the search space and improve efficiency.
Another popular path planning algorithm for satellite maps is the Rapidly-exploring Random Tree (RRT) algorithm. RRT is a probabilistic algorithm that generates a tree of random samples and connects them to form a path. RRT has been shown to be effective in dealing with complex environments and non-holonomic constraints. However, RRT has limitations when dealing with dynamic obstacles or multi-objective optimization problems.
In recent years, machine learning techniques have been applied to path planning for satellite maps. For example, Deep Reinforcement Learning (DRL) algorithms have been used to learn optimal paths in complex and dynamic environments. DRL algorithms use a combination of deep neural networks and reinforcement learning to learn policies that maximize a reward function. These algorithms have shown promising results in various applications, including autonomous navigation and robotics.
Another recent development in path planning for satellite maps is the integration of satellite data with other data sources such as social media and sensor data. This integration allows for more accurate and real-time path planning, which is essential in disaster response and emergency situations.
In conclusion, path planning for satellite maps is a challenging and evolving field, and several algorithms and techniques have been proposed over the years. While graph-based algorithms and RRT remain popular choices, machine learning techniques such as DRL have shown promising results. The integration of satellite data with other data sources is also a promising direction for future research.
相关推荐
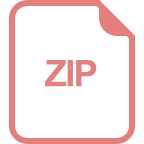
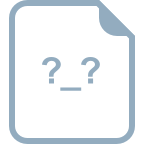
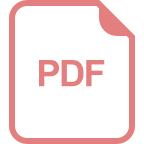











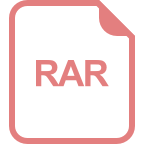