翻译:经本文研究发现多层感知机的平均预测精度最高,且FPR、FNR、TPR、TNR都最为优秀,所以本文中最合适的算法为多层感知机。
时间: 2024-05-19 10:14:41 浏览: 17
It was found through the study in this article that multi-layer perceptron has the highest average prediction accuracy and the most excellent FPR, FNR, TPR, and TNR. Therefore, the most suitable algorithm in this article is multi-layer perceptron.
相关问题
FPR、FNR、TPR、TNR数据分析里中文叫什么
FPR(False Positive Rate):假阳性率,也叫误报率;
FNR(False Negative Rate):假阴性率,也叫漏报率;
TPR(True Positive Rate):真阳性率,也叫命中率、召回率、灵敏度;
TNR(True Negative Rate):真阴性率,也叫特异性。
roc_auc = auc(fpr, tpr) TypeError: 'numpy.float64' object is not callable
这错误提示表明在代码中尝试调用了一个numpy.float64类型的对象,而这个对象不是可调用的函数。通常这种错误是由于变量名和函数名重复导致的。在这个问题中,可能是在之前的代码中已经定义了一个名为“auc”的变量,导致在调用“auc(fpr, tpr)”时出现了错误。解决方法是更改变量名或者使用完整的函数名“metrics.auc”来调用函数。例如:roc_auc = metrics.auc(fpr, tpr)。
相关推荐
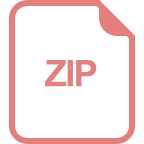









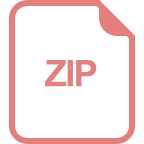
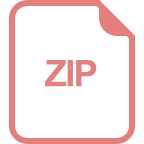
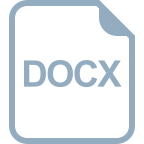
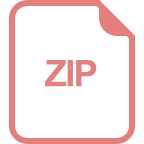