reg=stats.OLS.from_formula('price~sqft_living+bedrooms+age',housing).fit()什么意思
时间: 2024-03-04 07:53:14 浏览: 22
这段代码使用了Python中的statsmodels库的OLS模型,通过最小二乘法来拟合一个线性回归模型。其中,'price~sqft_living+bedrooms+age'是线性回归模型的公式,表示因变量为price,自变量分别为sqft_living、bedrooms和age。housing是数据集。拟合后的结果存储在reg变量中。
相关问题
reg=stats.OLS.from_formula('price~sqft_living+bedrooms+age',train).fit()什么意思
这段代码使用了Python中的statsmodels库的OLS模型,通过最小二乘法来拟合一个线性回归模型。其中,'price~sqft_living+bedrooms+age'是线性回归模型的公式,表示因变量为price,自变量分别为sqft_living、bedrooms和age。train是数据集,通常是用于机器学习模型的训练集。拟合后的结果存储在reg变量中。
reg=stats.OLS.from_formula('y~x1,x2,x3').fit()是什么意思
`reg=stats.OLS.from_formula('y~x1,x2,x3').fit()`是一个用于根据公式字符串进行线性回归分析的代码,其中:
- `stats.OLS.from_formula()`是一个函数,用于根据公式字符串进行线性回归分析。
- `'y~x1,x2,x3'`是一个公式字符串,表示回归模型的形式。其中,`y`表示目标变量,`x1`、`x2`和`x3`表示自变量,中间使用逗号`,`进行分隔。
- `reg`是一个变量,表示线性回归模型的结果。通过调用`fit()`方法对模型进行拟合后,可以获得回归系数、截距、拟合优度、标准误差等指标。
在Python中,通常使用`statsmodels`库进行回归分析。使用`statsmodels`库时,可以使用`stats.OLS.from_formula()`函数根据公式字符串进行线性回归分析,从而方便地指定回归模型中的变量和函数,以及进行变量的转换和交互。
相关推荐
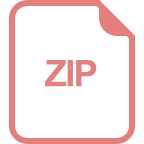












