智能优化算法里的莱维飞行策略的代码及讲解
时间: 2024-02-25 22:51:14 浏览: 125
莱维飞行策略(Levy Flight)是一种基于随机游走的优化算法,它模拟了莱维分布中的随机步长。其主要思想是通过一定的概率分布来控制搜索过程中的步长和方向,从而更好地探索搜索空间。下面是莱维飞行策略的代码实现及讲解:
```python
import numpy as np
# 莱维分布的概率密度函数
def levy_pdf(x):
return 1.0 / (x * np.sqrt(2 * np.pi)) * np.exp(-1.0 / (2 * x))
# 生成莱维飞行的步长
def levy_flight(beta=1.5):
sigma = np.power(np.math.gamma(1 + beta) * np.sin(np.pi * beta / 2) / np.math.gamma((1 + beta) / 2) * beta * np.power(2, (beta - 1) / 2), 1 / beta)
u = np.random.normal(0, sigma)
v = np.random.normal(0, 1)
step = u / np.power(np.abs(v), 1 / beta)
return step
# 莱维飞行策略的实现
def levy_flight_search(objective_function, bounds, n_iterations, n_pop):
best_solution = None
best_fitness = np.inf
pop_size = (n_pop, len(bounds))
pop = np.random.uniform(bounds[:, 0], bounds[:, 1], size=pop_size)
for i in range(n_iterations):
for j in range(n_pop):
# 生成莱维飞行的步长
step = levy_flight()
# 更新位置
candidate = pop[j] + step * (pop[j] - best_solution)
candidate = np.clip(candidate, bounds[:, 0], bounds[:, 1])
# 计算适应度
fitness = objective_function(candidate)
# 更新最优解
if fitness < best_fitness:
best_solution = candidate
best_fitness = fitness
print('Iteration {}: Best F({}) = {}'.format(i, best_solution, best_fitness))
return best_solution, best_fitness
```
上述代码中,`levy_pdf` 函数用于计算莱维分布的概率密度函数,`levy_flight` 函数用于生成莱维飞行的步长,`levy_flight_search` 函数实现了莱维飞行策略的搜索过程。
具体来说,莱维飞行的步长是根据莱维分布生成的,其中 `beta` 是莱维分布的形状参数,一般取值在 1.5 到 3 之间。在 `levy_flight` 函数中,先计算出莱维分布的标准差 `sigma`,然后生成两个标准正态分布的随机数 `u` 和 `v`,最后根据莱维分布的公式计算出步长 `step`。
在 `levy_flight_search` 函数中,首先生成随机的初始种群 `pop`,然后进行多次迭代,每次迭代对每个个体进行更新。具体地,使用莱维飞行生成一个新的候选解 `candidate`,然后将其限制在搜索空间内。计算候选解的适应度 `fitness`,并更新最优解 `best_solution` 和最优适应度 `best_fitness`。
最后,返回最优解和最优适应度即可。
需要注意的是,莱维飞行策略虽然具有一定的优化效果,但其收敛性和稳定性并不稳定,因此在实际应用中需要谨慎使用。
相关推荐
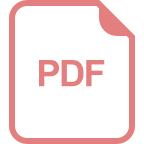














