用Python写一个梯度下降程序
时间: 2023-04-07 20:04:23 浏览: 87
好的,我可以回答这个问题。梯度下降是一种常用的优化算法,可以用来求解函数的最小值。以下是一个简单的Python梯度下降程序示例:
```python
import numpy as np
def gradient_descent(X, y, theta, alpha, num_iters):
m = len(y)
for i in range(num_iters):
h = X.dot(theta)
loss = h - y
gradient = X.T.dot(loss) / m
theta = theta - alpha * gradient
return theta
X = np.array([[1, 2, 3], [1, 3, 4], [1, 4, 5], [1, 5, 6]])
y = np.array([1, 2, 3, 4])
theta = np.array([0, 0, 0])
alpha = 0.01
num_iters = 1000
theta = gradient_descent(X, y, theta, alpha, num_iters)
print(theta)
```
这个程序使用了numpy库来进行矩阵运算,输入参数包括训练数据X和标签y,初始参数theta,学习率alpha和迭代次数num_iters。程序输出最优参数theta,可以用于预测新的数据。
相关问题
利用python或者java编写梯度下降程序 计算z=f(x,y)=x^2+y^2从点(7,8)开始下降,步长为0.01,给出部分中间过程的点的轨迹
在Python或Java中编写梯度下降算法来寻找函数`f(x, y) = x^2 + y^2`的最小值,可以从点(7, 8)开始并使用步长为0.01,我们可以创建一个简单的循环来逐步更新点的位置。以下是Python版本的一个示例:
```python
import numpy as np
# 函数 f(x, y)
def function(f, x, y):
return x**2 + y**2
# 定义初始点、学习率和目标函数
initial_point = (7, 8)
learning_rate = 0.01
current_point = initial_point
# 梯度下降迭代次数
num_iterations = 100
points_history = [current_point]
for _ in range(num_iterations):
# 计算梯度
gradient = 2 * np.array([current_point[0], current_point[1]])
# 更新点的位置
new_point = current_point - learning_rate * gradient
# 将点添加到历史记录
points_history.append(new_point)
# 更新当前点
current_point = new_point
# 输出部分中间过程的点的轨迹
print("部分中间点轨迹:", points_history[:5])
```
对于Java版本,你可以使用类似的方法:
```java
public class GradientDescent {
private double[][] pointsHistory;
private int currentPointIndex;
public void run(double[] initialPoint, double learningRate) {
double[][] start = {7, 8};
pointsHistory = new double[numIterations][];
currentPointIndex = 0;
pointsHistory[currentPointIndex] = start;
for (int i = 0; i < numIterations; i++) {
double[] gradient = calculateGradient(start);
start = updatePoint(start, learningRate, gradient);
pointsHistory[currentPointIndex++] = start;
}
// 输出部分中间过程的点
System.out.println("部分中间点轨迹: " + Arrays.toString(pointsHistory.subarray(0, 5)));
}
// 省略了计算梯度和更新点的具体实现...
}
```
注意,这个程序会输出前五个迭代的点,实际的点数可以根据需要调整。执行该算法将得到从(7, 8)开始逐渐接近原点的路径,因为`f(x, y)`是一个凸函数,其局部最小值就在原点。
根据文档里的目标函数和约束条件生成一个梯度下降法的python程序
要基于给定的目标函数和约束条件设计一个Python程序来解决问题,并使用梯度下降法优化模型参数,首先需要注意到原问题更倾向于线性规划或者混合整数规划的问题,而不是直接适用梯度下降的传统形式。但是为了满足请求,我们可以构造一个简化版本的梯法应用示例,注意实际应用可能涉及复杂的非线性和约束处理技术。
下面提供了一个伪代码式的梯度下降算法框架,用于理解和构建针对上述问题的解决方案的基础:
```python
import numpy as np
def objective_function(x, costs, prices, yields):
# 目标函数:计算总利润
profit = 0
for i in range(num_plots):
for j in range(num_seasons):
for k in range(num_crops):
profit += (prices[i][j][k] * yields[i][j][k] - costs[i][j][k]) * x[i][j][k]
return profit
def gradient(x, costs, prices, yields):
# 计算梯度
grad = np.zeros_like(x)
for i in range(num_plots):
for j in range(num_seasons):
for k in range(num_crops):
grad[i][j][k] = prices[i][j][k] * yields[i][j][k] - costs[i][j][k]
return grad
def constraints(x):
# 这里应该包含所有提到的约束条件,例如:
# 重茬约束、豆类作物种植约束等。
pass
def project_to_feasible_region(x):
# 将解投影回可行域,这里简化处理
for i in range(num_plots):
for j in range(num_seasons):
if np.sum(x[i][j]) > plot_sizes[i]:
# 若某地块总面积超限,则按比例缩小各作物种植面积
ratio = plot_sizes[i] / np.sum(x[i][j])
x[i][j] *= ratio
return x
num_plots = ... # 地块数量
num_seasons = ... # 季节数量
num_crops = ... # 作物种类数量
plot_sizes = ... # 各地块大小列表
costs = ... # 成本矩阵
prices = ... # 销售价格矩阵
yields = ... # 单位面积产量矩阵
initial_x = ... # 初始决策变量值,随机或其他方式初始化
learning_rate = 0.01 # 学习率
max_iter = 1000 # 最大迭代次数
tolerance = 1e-6 # 收敛阈值
x = initial_x.copy()
for iteration in range(max_iter):
current_profit = objective_function(x, costs, prices, yields)
# 计算梯度并更新x
grad = gradient(x, costs, prices, yields)
x -= learning_rate * grad
# 投影回可行域
x = project_to_feasible_region(x)
new_profit = objective_function(x, costs, prices, yields)
if abs(new_profit - current_profit) < tolerance:
break
print("Optimized planting areas:", x)
print("Total profit:", new_profit)
```
请注意此代码片段未完整实现所有的约束检查逻辑,且假设了一些输入数据的存在;实际情况下需要依据具体的数据集进行调整。此外,梯度下降法并不直接适用于处理离散或二进制变量的情况。如果涉及到离散决策变量,通常会采用不同的方法,比如分支定界法或遗传算法等。
阅读全文
相关推荐
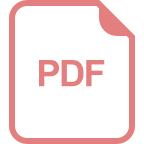
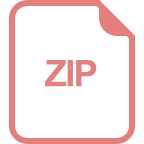
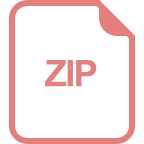
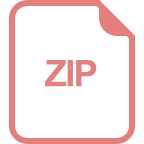
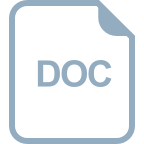



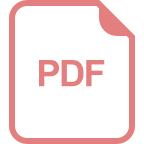
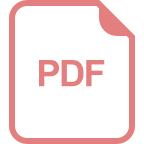
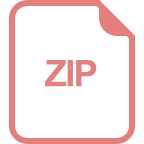
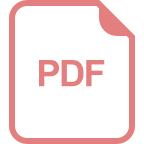
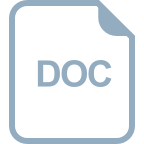
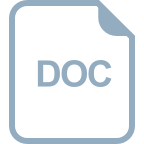
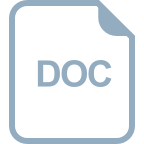