以下代码将数据集怎样划分的:from sklearn.model_selection import train_test_split X_train,X_test,y_train,y_test = train_test_split(loan,y,test_size=.15, random_state=10,stratify=y)
时间: 2023-11-14 21:41:19 浏览: 27
这段代码使用了`train_test_split`函数,将数据集分为训练集和测试集。其中,`loan`是特征矩阵,`y`是目标变量。`test_size`参数指定了测试集所占的比例(这里是15%),`random_state`参数指定了随机种子,用于保证每次划分的结果都是一样的。`stratify`参数指定了按目标变量进行分层抽样,保证训练集和测试集中的目标变量的分布比例相同。最终,函数返回四个变量:`X_train`表示训练集的特征矩阵,`X_test`表示测试集的特征矩阵,`y_train`表示训练集的目标变量,`y_test`表示测试集的目标变量。
相关问题
from sklearn.model_selection import train_test_split与from sklearn.cross_validation import train_test_split 有什么不同
`from sklearn.model_selection import train_test_split`和`from sklearn.cross_validation import train_test_split`两者之间的不同在于模块的导入方式。
`from sklearn.model_selection import train_test_split`是在Scikit-learn 0.18版本之后引入的,用于数据集的划分。这个模块提供了更多的功能和选项,例如可以指定随机种子、分层抽样等。
而`from sklearn.cross_validation import train_test_split`是在Scikit-learn 0.17版本之前的旧版本中使用的模块。在Scikit-learn 0.18版本之后,这个模块被`model_selection`模块替代。
因此,建议使用`from sklearn.model_selection import train_test_split`来导入`train_test_split`函数,以保持与最新版本的Scikit-learn的兼容性。
from sklearn.model_selection import train_test_split、
`from sklearn.model_selection import train_test_split` 是一个用于数据集划分的函数,它可以将数据集划分为训练集和测试集。这个函数在机器学习中非常常用,因为我们需要将数据集划分为训练集和测试集来评估模型的性能。
使用 `train_test_split` 函数可以将数据集按照指定的比例划分为训练集和测试集。它的常见用法如下:
```python
from sklearn.model_selection import train_test_split
X_train, X_test, y_train, y_test = train_test_split(X, y, test_size=0.2, random_state=42)
```
其中,`X` 是特征矩阵,`y` 是目标变量。`test_size` 参数指定了测试集所占的比例,这里设置为 0.2 表示测试集占总数据集的 20%。`random_state` 参数用于设置随机种子,保证每次划分的结果一致。
划分完成后,`X_train` 和 `y_train` 是训练集的特征矩阵和目标变量,`X_test` 和 `y_test` 是测试集的特征矩阵和目标变量。
相关推荐
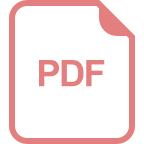
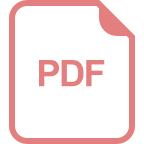
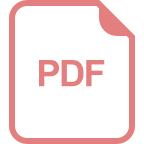
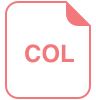
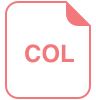
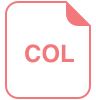
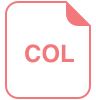
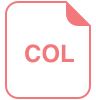







