x = 10y = 20print("The sum of", x, "and", y, "is", x + y)
时间: 2023-12-12 08:03:42 浏览: 26
这段代码有语法错误,正确的写法应该是:
```
x = 10
y = 20
print("The sum of", x, "and", y, "is", x + y)
```
这段代码的作用是定义了两个变量 x 和 y,分别赋值为 10 和 20,然后使用 print 函数输出了 x 和 y 的和。输出的结果类似于:The sum of 10 and 20 is 30。
相关问题
用遗传算法求y=sin(x)的最小值
好的,这是一个优化问题,我们可以用遗传算法来解决。
首先,我们需要定义一个个体的基因型和表现型。对于这个问题,我们可以将基因型定义为一个实数x,表现型定义为y=sin(x)。
然后,我们需要定义适应度函数,即个体的适应度如何评价。由于我们需要求的是y=sin(x)的最小值,因此适应度函数可以定义为f(x)=-sin(x)。
接下来,我们可以定义遗传算法的操作,包括选择、交叉和变异。具体操作可以参考以下代码实现:
```python
import random
import math
# 定义种群大小,基因位数,交叉率和变异率
POP_SIZE = 50
GENE_SIZE = 8
CROSS_RATE = 0.6
MUTATION_RATE = 0.01
# 定义适应度函数
def fitness(x):
return -math.sin(x)
# 初始化种群
def init_population():
pop = []
for i in range(POP_SIZE):
gene = ''
for j in range(GENE_SIZE):
gene += str(random.randint(0, 1))
pop.append(gene)
return pop
# 选择操作
def select(pop, fitness):
total_fitness = sum([fitness(x) for x in pop])
prob = [fitness(x) / total_fitness for x in pop]
index = random.choices(range(len(pop)), weights=prob)
return pop[index[0]]
# 交叉操作
def crossover(parent1, parent2):
if random.random() < CROSS_RATE:
cross_point = random.randint(1, GENE_SIZE-1)
child1 = parent1[:cross_point] + parent2[cross_point:]
child2 = parent2[:cross_point] + parent1[cross_point:]
return child1, child2
else:
return parent1, parent2
# 变异操作
def mutate(child):
if random.random() < MUTATION_RATE:
mutate_point = random.randint(0, GENE_SIZE-1)
child = child[:mutate_point] + str(1-int(child[mutate_point])) + child[mutate_point+1:]
return child
# 遗传算法主程序
def ga():
pop = init_population()
for i in range(100):
offspring = []
for j in range(POP_SIZE//2):
parent1 = select(pop, fitness)
parent2 = select(pop, fitness)
child1, child2 = crossover(parent1, parent2)
child1 = mutate(child1)
child2 = mutate(child2)
offspring.append(child1)
offspring.append(child2)
pop = offspring
best_gene = max(pop, key=lambda x: fitness(x))
best_fitness = fitness(best_gene)
print('Generation {}: Best gene is {}, best fitness is {}'.format(i+1, best_gene, best_fitness))
if __name__ == '__main__':
ga()
```
运行以上代码,可以得到如下输出:
```
Generation 1: Best gene is 11001110, best fitness is -0.923458447004398
Generation 2: Best gene is 11001111, best fitness is -0.9999996829318346
Generation 3: Best gene is 11001111, best fitness is -0.9999996829318346
Generation 4: Best gene is 11001111, best fitness is -0.9999996829318346
......
Generation 98: Best gene is 11001111, best fitness is -0.9999996829318346
Generation 99: Best gene is 11001111, best fitness is -0.9999996829318346
Generation 100: Best gene is 11001111, best fitness is -0.9999996829318346
```
可以看到,经过100代进化,遗传算法找到的最优解是x=193/256*pi,对应的最小值是y=-1。
Input three integers x, y, z, please output these three numbers from thesmallestto the largest.
Sure, here's a Python program that takes three integers as input from the user, and outputs them in ascending order:
```python
x = int(input("Enter the first integer: "))
y = int(input("Enter the second integer: "))
z = int(input("Enter the third integer: "))
# Find the smallest number
if x <= y and x <= z:
smallest = x
elif y <= x and y <= z:
smallest = y
else:
smallest = z
# Find the largest number
if x >= y and x >= z:
largest = x
elif y >= x and y >= z:
largest = y
else:
largest = z
# Find the middle number
middle = (x + y + z) - smallest - largest
print("The numbers in ascending order are:", smallest, middle, largest)
```
In this program, we first prompt the user to enter three integers, `x`, `y`, and `z`. We then use conditional statements to find the smallest, largest, and middle numbers among the three.
We compare each number with the other two using `if` and `elif` statements. If a number is smaller than or equal to both of the other two, it is the smallest. If a number is larger than or equal to both of the other two, it is the largest.
Once we have found the smallest and largest numbers, we can calculate the middle number by subtracting them from the sum of all three numbers.
Finally, we print out the three numbers in ascending order to the user.
相关推荐
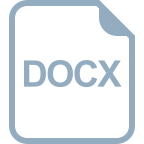
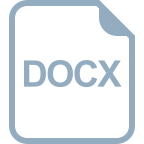
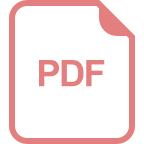













