python实现单层感知器学习算法,验证算法的正确性,选择iris-人工神经网络.txt中的一部分数据集作为训练集,通过训练集对感知器的连接权重进行学习
时间: 2024-02-06 21:09:16 浏览: 120
好的,以下是Python实现单层感知器学习算法的示例代码:
```python
import numpy as np
class Perceptron:
def __init__(self, input_size, lr=1, epochs=100):
self.W = np.zeros(input_size+1)
self.epochs = epochs
self.lr = lr
def activation_fn(self, x):
return 1 if x >= 0 else 0
def predict(self, x):
z = self.W.T.dot(x)
a = self.activation_fn(z)
return a
def fit(self, X, d):
for epoch in range(self.epochs):
for i in range(d.shape[0]):
x = np.insert(X[i], 0, 1)
y = self.predict(x)
e = d[i] - y
self.W = self.W + self.lr * e * x
# 测试代码
X = np.array([[0,0], [0,1], [1,0], [1,1]])
d = np.array([0, 0, 0, 1])
perceptron = Perceptron(input_size=2)
perceptron.fit(X, d)
print(perceptron.W)
```
其中,`Perceptron`类中的`activation_fn`方法定义了激活函数,这里使用的是阶跃函数。`predict`方法用于根据输入向量预测输出值,`fit`方法则是训练模型的核心方法。在测试代码中,我们使用了一个简单的逻辑与门问题作为测试数据,其中X为输入,d为期望输出。
对于iris-人工神经网络.txt数据集,我们需要根据数据集中的特征训练出一个模型,用于预测鸢尾花的类别。以下是代码示例:
```python
import numpy as np
import pandas as pd
# 读取数据集
df = pd.read_csv('iris-人工神经网络.txt', header=None)
# 随机打乱数据集
df = df.sample(frac=1)
# 将类别转化为数字
df[4] = pd.Categorical(df[4])
df[4] = df[4].cat.codes
# 将数据集分为训练集和测试集
train_size = int(0.7 * len(df))
X_train, y_train = df.iloc[:train_size,:-1].values, df.iloc[:train_size,-1].values
X_test, y_test = df.iloc[train_size:,:-1].values, df.iloc[train_size:,-1].values
# 定义模型
class Perceptron:
def __init__(self, input_size, lr=0.1, epochs=100):
self.W = np.zeros(input_size+1)
self.epochs = epochs
self.lr = lr
def activation_fn(self, x):
return 1 if x >= 0 else 0
def predict(self, x):
z = self.W.T.dot(x)
a = self.activation_fn(z)
return a
def fit(self, X, d):
for epoch in range(self.epochs):
for i in range(d.shape[0]):
x = np.insert(X[i], 0, 1)
y = self.predict(x)
e = d[i] - y
self.W = self.W + self.lr * e * x
# 训练模型
perceptron = Perceptron(input_size=X_train.shape[1])
perceptron.fit(X_train, y_train)
# 测试模型
correct = 0
for i in range(X_test.shape[0]):
x = np.insert(X_test[i], 0, 1)
y = perceptron.predict(x)
if y == y_test[i]:
correct += 1
accuracy = correct / X_test.shape[0]
print('Accuracy:', accuracy)
```
在代码中,我们首先读取了iris-人工神经网络.txt数据集,并将类别转换为数字。接着将数据集分为训练集和测试集。然后定义了一个`Perceptron`类,并根据训练集训练模型。最后在测试集上测试模型,并计算准确率。
阅读全文
相关推荐
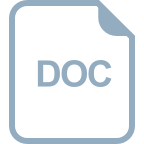
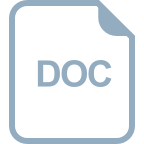
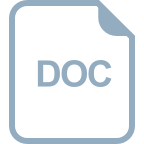




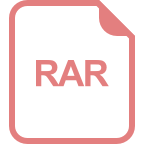
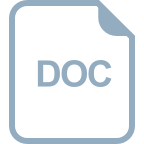
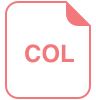
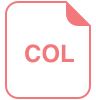
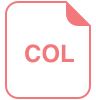
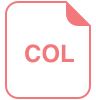
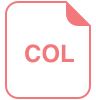
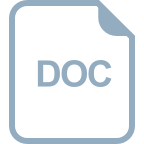
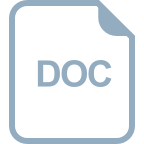
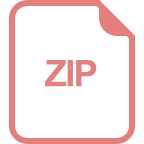