data = importdata('1-5.txt', ' '); x = data(:, 1); image = data(:, 2); % 获取波峰位置数据 [pks, locs] = findpeaks(image); % 归一化处理波峰位置数据 normalized_locs = normalize(locs, 'range'); % 自适应背景扣除 window_size = 30; % 窗口大小 corrected_image = zeros(size(image)); for i = 1:length(image) % 确定当前窗口的起始和结束位置 window_start = max(i - floor(window_size/2), 1); window_end = min(i + floor(window_size/2), length(image)); % 获取当前窗口内的背景估计 background = median(image(window_start:window_end)); % 扣除背景估计 corrected_image(i) = image(i) - background; end
时间: 2023-06-24 08:04:30 浏览: 66
这段代码的作用是对一个图像的波峰位置数据进行处理,包括归一化处理、自适应背景扣除等。
首先,通过`importdata`函数读取名为`1-5.txt`的文件,并将第一列数据存储到变量`x`中,将第二列数据存储到变量`image`中。
接下来,使用`findpeaks`函数寻找`image`中的波峰位置,并将波峰位置数据存储在`pks`和`locs`两个变量中。
然后,使用`normalize`函数对`locs`进行归一化处理,得到`normalized_locs`。
最后,使用一个`for`循环,对`image`中每一个像素点进行自适应背景扣除。具体来说,对于每一个像素点,确定当前窗口的起始和结束位置,计算窗口内的背景估计,并将背景估计值从该像素点的值中扣除,得到经过背景扣除后的值,存储在`corrected_image`中。其中,窗口大小由`window_size`指定,这里是取窗口大小的一半作为窗口半径,即`floor(window_size/2)`。
相关问题
java解析Content-Disposition:from-data;name="data" Content-Type:image/xxx 代码
你可以使用Java中的javax.servlet.http.Part类来解析Content-Disposition头部中的数据。以下是一个示例代码:
```java
import javax.servlet.http.Part;
import java.io.InputStream;
// 获取Part对象
Part part = request.getPart("data");
// 获取Content-Disposition头部中的name属性值
String name = part.getName();
// 获取Content-Disposition头部中的filename属性值
String filename = getFilename(part);
// 获取Content-Type头部中的值
String contentType = part.getContentType();
// 获取文件内容
InputStream inputStream = part.getInputStream();
// 在这里可以对文件内容进行处理
// 获取filename属性值的方法
private String getFilename(Part part) {
String contentDisposition = part.getHeader("content-disposition");
String[] elements = contentDisposition.split(";");
for (String element : elements) {
if (element.trim().startsWith("filename")) {
return element.substring(element.indexOf('=') + 1).trim()
.replace("\"", "");
}
}
return null;
}
```
在上述代码中,`request.getPart("data")`会获取名为"data"的Part对象。然后,可以使用`part.getName()`获取name属性值,使用`getFilename(part)`获取filename属性值,使用`part.getContentType()`获取Content-Type头部中的值。最后,可以通过`part.getInputStream()`获取文件的内容。
请注意,这是一个基本示例代码,你可能需要根据你的具体需求做一些修改和扩展。
没有GPU,优化程序class point_cloud_generator(): def init(self, rgb_file, depth_file, save_ply, camera_intrinsics=[312.486, 243.928, 382.363, 382.363]): self.rgb_file = rgb_file self.depth_file = depth_file self.save_ply = save_ply self.rgb = cv2.imread(rgb_file) self.depth = cv2.imread(self.depth_file, -1) print("your depth image shape is:", self.depth.shape) self.width = self.rgb.shape[1] self.height = self.rgb.shape[0] self.camera_intrinsics = camera_intrinsics self.depth_scale = 1000 def compute(self): t1 = time.time() depth = np.asarray(self.depth, dtype=np.uint16).T self.Z = depth / self.depth_scale fx, fy, cx, cy = self.camera_intrinsics X = np.zeros((self.width, self.height)) Y = np.zeros((self.width, self.height)) for i in range(self.width): X[i, :] = np.full(X.shape[1], i) self.X = ((X - cx / 2) * self.Z) / fx for i in range(self.height): Y[:, i] = np.full(Y.shape[0], i) self.Y = ((Y - cy / 2) * self.Z) / fy data_ply = np.zeros((6, self.width * self.height)) data_ply[0] = self.X.T.reshape(-1)[:self.width * self.height] data_ply[1] = -self.Y.T.reshape(-1)[:self.width * self.height] data_ply[2] = -self.Z.T.reshape(-1)[:self.width * self.height] img = np.array(self.rgb, dtype=np.uint8) data_ply[3] = img[:, :, 0:1].reshape(-1)[:self.width * self.height] data_ply[4] = img[:, :, 1:2].reshape(-1)[:self.width * self.height] data_ply[5] = img[:, :, 2:3].reshape(-1)[:self.width * self.height] self.data_ply = data_ply t2 = time.time() print('calcualte 3d point cloud Done.', t2 - t1) def write_ply(self): start = time.time() float_formatter = lambda x: "%.4f" % x points = [] for i in self.data_ply
It that the code is generating a point cloud from an RGB-D image pair. Since you mentioned that you do not have a GPU, one possible optimization could be to use the `numba` library to speed up the computation. Here is how you can modify the code to use `numba`:
1. Import the `numba` library by adding the following line at the top of your code:
```python
import numba
```
2. Add the `@numba.jit(nopython=True)` decorator to the `compute` method to enable `numba` JIT compilation:
```python
@numba.jit(nopython=True)
def compute(self):
# rest of the code
```
3. Modify the `for` loops in the `compute` method to use `numba`'s `prange` function to parallelize the computation across multiple CPU cores:
```python
for i in numba.prange(self.width):
# rest of the code
for i in numba.prange(self.height):
# rest of the code
```
4. Add the `@numba.jit(nopython=True)` decorator to the `write_ply` method as well, since it is also computationally intensive:
```python
@numba.jit(nopython=True)
def write_ply(self):
# rest of the code
```
With these modifications, the `compute` and `write_ply` methods should run faster. However, keep in mind that `numba`'s JIT compilation can take some time during the first function call, so subsequent calls should be faster. Also, note that `numba`'s `prange` function only works with integer indices, so you may need to convert floating-point indices to integers before using them in the loops.
相关推荐
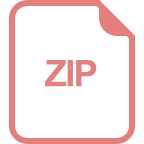
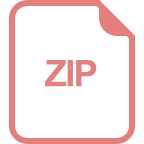
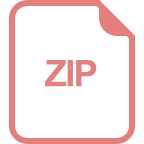













