No data files read in.
时间: 2024-04-25 15:27:34 浏览: 103
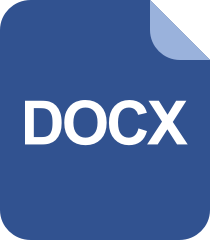
2021-2022计算机二级等级考试试题及答案No.17518.docx
这个错误通常表示 SAC 没有读取到任何数据文件,可能是因为指定的数据文件不存在、文件名错误、文件路径错误或者文件格式不支持等原因。以下是一些可能的解决方法:
1. 确认文件名和路径是否正确,可以使用 `ls` 命令查看当前目录下的文件列表。
2. 确认文件格式是否支持,SAC 可以读取的数据格式包括 SAC、SEED、MSEED、AH、CSS、GSE、JWEED、KNET、PICKLE 等。
3. 确认数据文件是否已经被删除或者移动到了其他位置,可以使用文件管理器或者命令行查看文件是否存在。
4. 确认数据文件是否损坏,可以使用 `file` 命令查看文件类型,或者使用其他数据处理软件查看数据文件是否正常。
如果以上方法仍然不能解决问题,可以提供更多的错误信息和上下文,以便更好地定位和解决问题。
阅读全文
相关推荐
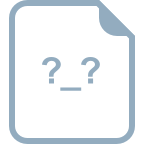
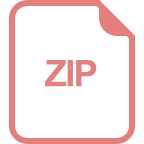
















