a='*'*i b='.'*(scale-i) c= t-=time.process_time() print("\r{:^3.0f}%[{}->{}]{:.2f}s".format(c,a,b,-t),end='') time.sleep(0.05),请把代码补全
时间: 2024-03-02 07:49:41 浏览: 82
好的,以下是代码的完整版本:
```python
import time
def progress_bar(scale):
t = time.process_time()
for i in range(scale+1):
a = '*' * i
b = '.' * (scale-i)
t -= time.process_time()
print("\r{:^3.0f}%[{}->{}]{:.2f}s".format(i/scale*100, a, b, -t), end='')
time.sleep(0.05)
print()
# 示例用法
progress_bar(50)
```
这个代码可以生成一个长度为50的进度条,并且在每次更新进度时会自动刷新显示。你可以调整`scale`变量的值来改变总进度,也可以在其他地方调用`progress_bar`函数来生成进度条。
相关问题
程序无法执行,修改class Processor(): def __init__(self): self._inspect_step = int(cfg.get('PROCESS', 'INSPECT_STEP')) def capture_img(self): global aco aco = aco + 1 self._cam.stream_on() raw_image = self._cam.data_stream[0].get_image() if raw_image is None: print("Getting image failed.\n") return None print("Frame ID: {} Height: {} Width: {} Count: {}\n" .format(raw_image.get_frame_id(), raw_image.get_height(), raw_image.get_width(), aco - 2)) numpy_image = raw_image.get_numpy_array() if numpy_image is None: return None img = Image.fromarray(numpy_image, 'L') if self._issave: picfile = '{}/{}.bmp'.format(self._picpath, self._piccount) self._piccount = self._piccount + 1 img.save(picfile) if self._isshow: w, h = img.size scale = min(1.0 * IMG_RESIZE_W / w, 1.0 * IMG_RESIZE_H / h) self._img = ImageTk.PhotoImage(image=img.resize((int(w * scale), int(h * scale)), Image.ANTIALIAS)) self._show_cb(self._img) self._cam.stream_off() return img def process_img(self, img): return PROC_OK def inspect(self): print("process2") time.sleep(1) def rotate(self): count = 0 aco = 0 self.threadsignal = 0 while self._running: v = self._dgl.qpin(ROT) if len(v) > 0: count = count + sum(v) if count > self._inspect_step: aco += 1 img = self.capture_img() count = count - self._inspect_step if __name__ == '__main__': task2 = multiprocessing.Process(target=self.inspect) task2.start() task1 = multiprocessing.Process(target=self.rotate) task1.start()
在这段代码中,有几个问题可以被改进。首先,类 `Processor` 中的全局变量 `aco` 可能会导致并发问题,在多进程环境下应该避免使用全局变量。其次,代码中出现了 `time.sleep()`,这会阻塞当前进程并造成性能瓶颈,应该尽量避免使用。
以下是可能的改进方案:
1. 将 `aco` 变量改为实例变量,避免多进程之间的并发问题。
2. 使用 `Queue` 或其他进程间通信机制替代全局变量,避免多进程之间的竞争。
3. 将 `time.sleep()` 改为使用定时器或者事件,避免阻塞进程并提高程序的性能。
4. 在多进程环境中,使用 `if __name__ == '__main__':` 来避免创建子进程时出现递归调用的问题。
以下是修改后的代码示例:
```python
import multiprocessing
from queue import Queue
import time
class Processor():
def __init__(self):
self._inspect_step = int(cfg.get('PROCESS', 'INSPECT_STEP'))
self._aco = 0
self._queue = Queue()
def capture_img(self):
self._aco += 1
self._cam.stream_on()
raw_image = self._cam.data_stream[0].get_image()
if raw_image is None:
print("Getting image failed.\n")
return None
print("Frame ID: {} Height: {} Width: {} Count: {}\n".format(raw_image.get_frame_id(), raw_image.get_height(), raw_image.get_width(), self._aco - 2))
numpy_image = raw_image.get_numpy_array()
if numpy_image is None:
return None
img = Image.fromarray(numpy_image, 'L')
if self._issave:
picfile = '{}/{}.bmp'.format(self._picpath, self._piccount)
self._piccount = self._piccount + 1
img.save(picfile)
if self._isshow:
w, h = img.size
scale = min(1.0 * IMG_RESIZE_W / w, 1.0 * IMG_RESIZE_H / h)
self._img = ImageTk.PhotoImage(image=img.resize((int(w * scale), int(h * scale)), Image.ANTIALIAS))
self._show_cb(self._img)
self._cam.stream_off()
return img
def process_img(self, img):
return PROC_OK
def inspect(self):
print("process2")
while self._running:
time.sleep(1)
self._queue.put("task")
def rotate(self):
count = 0
while self._running:
v = self._dgl.qpin(ROT)
if len(v) > 0:
count = count + sum(v)
if count > self._inspect_step:
self._aco += 1
img = self.capture_img()
count = count - self._inspect_step
try:
task = self._queue.get(block=False)
if task == "task":
print("processing task")
except:
pass
if __name__ == '__main__':
p = Processor()
p._running = True
task2 = multiprocessing.Process(target=p.inspect)
task2.start()
task1 = multiprocessing.Process(target=p.rotate)
task1.start()
```
请注意,这里只是对原始代码的一些修改建议,并不能保证这样的修改能够完全解决问题。在实际环境中,还需要根据具体情况进行调整和优化。
代码解释# Process detections for i, det in enumerate(pred): # detections per image if webcam: # batch_size >= 1 p, s, im0 = path[i], '%g: ' % i, im0s[i].copy() else: p, s, im0 = path, '', im0s save_path = str(Path(out) / Path(p).name) s += '%gx%g ' % img.shape[2:] # print string gn = torch.tensor(im0.shape)[[1, 0, 1, 0]] # normalization gain whwh if det is not None and len(det): # Rescale boxes from img_size to im0 size det[:, :4] = scale_coords(img.shape[2:], det[:, :4], im0.shape).round() # Print results for c in det[:, -1].unique(): n = (det[:, -1] == c).sum() # detections per class s += '%g %ss, ' % (n, names[int(c)]) # add to string # Write results for *xyxy, conf, cls in det: if save_txt: # Write to file xywh = (xyxy2xywh(torch.tensor(xyxy).view(1, 4)) / gn).view(-1).tolist() # normalized xywh with open(save_path[:save_path.rfind('.')] + '.txt', 'a') as file: file.write(('%g ' * 5 + '\n') % (cls, *xywh)) # label format if save_img or view_img: # Add bbox to image label = '%s %.2f' % (names[int(cls)], conf) if label is not None: if (label.split())[0] == 'person': people_coords.append(xyxy) # plot_one_box(xyxy, im0, line_thickness=3) plot_dots_on_people(xyxy, im0) # Plot lines connecting people distancing(people_coords, im0, dist_thres_lim=(100, 150)) # Print time (inference + NMS) print('%sDone. (%.3fs)' % (s, t2 - t1)) # Stream results if 1: ui.showimg(im0) if cv2.waitKey(1) == ord('q'): # q to quit raise StopIteration # Save results (image with detections) if save_img: if dataset.mode == 'images': cv2.imwrite(save_path, im0) else: if vid_path != save_path: # new video vid_path = save_path if isinstance(vid_writer, cv2.VideoWriter): vid_writer.release() # release previous video writer fps = vid_cap.get(cv2.CAP_PROP_FPS) w = int(vid_cap.get(cv2.CAP_PROP_FRAME_WIDTH)) h = int(vid_cap.get(cv2.CAP_PROP_FRAME_HEIGHT)) vid_writer = cv2.VideoWriter(save_path, cv2.VideoWriter_fourcc(*opt.fourcc), fps, (w, h)) vid_writer.write(im0)
这段代码是目标检测算法的输出结果处理部分。主要包括以下几个步骤:
1. 对每张图片的检测结果进行处理,包括将检测框从输入图像的尺寸缩放到输出图像的尺寸,并将结果写入文本文件中。
2. 对每个类别的检测结果统计数量,并将数量和类别名称添加到输出字符串中。
3. 对每个检测到的目标绘制边界框,并在边界框上标注类别和置信度。
4. 如果检测到的目标是人,则将其坐标保存在列表中,并在图像上绘制点和连线进行社交距离监测。
5. 将处理后的图像展示出来,并将图像保存到文件中。
阅读全文
相关推荐
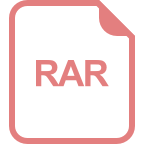
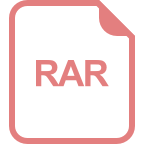
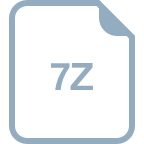










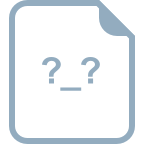
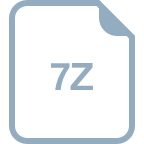
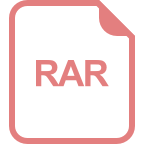
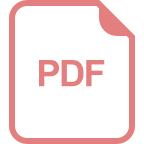