写一段7分类的深度残差收缩网络pytorch代码
时间: 2023-12-10 20:37:27 浏览: 89
以下是一个简单的7分类深度残差收缩网络的 PyTorch 代码示例:
```python
import torch
import torch.nn as nn
import torch.nn.functional as F
class ResNet(nn.Module):
def __init__(self, num_classes=7):
super(ResNet, self).__init__()
self.conv1 = nn.Conv2d(3, 64, kernel_size=3, stride=1, padding=1, bias=False)
self.bn1 = nn.BatchNorm2d(64)
self.relu = nn.ReLU(inplace=True)
self.layer1 = self._make_layer(64, 2)
self.layer2 = self._make_layer(128, 2, stride=2)
self.layer3 = self._make_layer(256, 2, stride=2)
self.layer4 = self._make_layer(512, 2, stride=2)
self.avgpool = nn.AdaptiveAvgPool2d((1, 1))
self.fc = nn.Linear(512, num_classes)
def _make_layer(self, planes, blocks, stride=1):
downsample = None
if stride != 1 or planes != 64:
downsample = nn.Sequential(
nn.Conv2d(64, planes, kernel_size=1, stride=stride, bias=False),
nn.BatchNorm2d(planes),
)
layers = []
layers.append(ResidualBlock(self.conv1, self.bn1, planes, stride, downsample))
for i in range(1, blocks):
layers.append(ResidualBlock(None, None, planes))
return nn.Sequential(*layers)
def forward(self, x):
x = self.relu(self.bn1(self.conv1(x)))
x = self.layer1(x)
x = self.layer2(x)
x = self.layer3(x)
x = self.layer4(x)
x = self.avgpool(x)
x = x.view(x.size(0), -1)
x = self.fc(x)
return x
class ResidualBlock(nn.Module):
def __init__(self, conv, bn, planes, stride=1, downsample=None):
super(ResidualBlock, self).__init__()
self.conv1 = conv if conv is not None else nn.Conv2d(planes, planes, kernel_size=3, stride=stride, padding=1, bias=False)
self.bn1 = bn if bn is not None else nn.BatchNorm2d(planes)
self.relu = nn.ReLU(inplace=True)
self.conv2 = nn.Conv2d(planes, planes, kernel_size=3, stride=1, padding=1, bias=False)
self.bn2 = nn.BatchNorm2d(planes)
self.downsample = downsample
self.stride = stride
def forward(self, x):
identity = x
out = self.relu(self.bn1(self.conv1(x)))
out = self.bn2(self.conv2(out))
if self.downsample is not None:
identity = self.downsample(x)
out += identity
out = self.relu(out)
return out
```
在上面的代码中,我们定义了一个 `ResNet` 类,它包含了多个残差块,每个块都包含两个卷积层和一个跳跃连接。我们还定义了一个 `ResidualBlock` 类,它包含了两个卷积层和一个跳跃连接。最后,我们使用全局平均池化层和全连接层对特征进行分类。
阅读全文
相关推荐
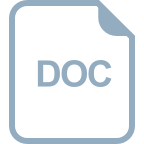
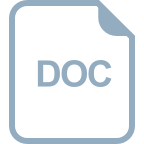
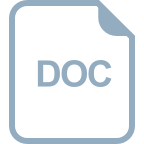
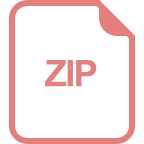
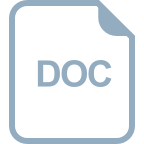
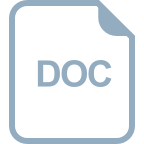
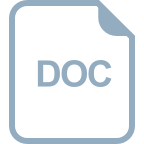
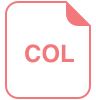
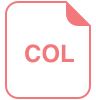
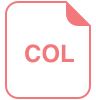
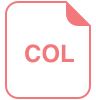

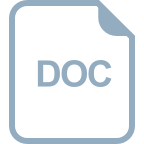
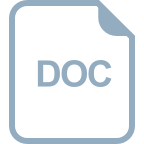
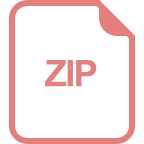