predictions = np.concatenate(predictions,prediction)出现TypeError: only integer scalar arrays can be converted to a scalar index是什么原因
时间: 2023-12-25 07:18:59 浏览: 26
这个错误是由于np.concatenate()函数的第一个参数应该是一个包含数组的序列,而不是单个数组。在这种情况下,将单个数组作为第一个参数传递给np.concatenate()函数导致了TypeError错误。要解决这个问题,需要将所有的预测数组作为一个序列传递给np.concatenate()函数,例如predictions = np.concatenate((predictions, prediction))。
相关问题
filtered_predictions = np.array(filtered_predictions)
这行代码的作用是将`filtered_predictions`转换为numpy数组。`filtered_predictions`是一个列表,通过`np.array()`函数将其转换为numpy数组,可以方便地进行一些数值计算和处理。最终,转换后的numpy数组被赋值给`filtered_predictions`变量。
predictions= np.dot(theta.transpose(),x)
这段代码使用了numpy库中的dot函数,计算了theta矩阵的转置和x矩阵的点积,得到一个一维数组predictions。其中theta是一个列数等于1的m行矩阵,x是一个n行m列的矩阵,predictions的长度为n。这个计算过程对应了线性回归模型中的预测过程,即通过输入特征x和模型参数theta,计算出模型的预测值。
相关推荐
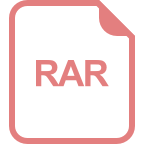
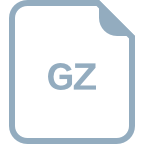












