def forward(self, x: torch.Tensor, H, W) -> torch.Tensor:
时间: 2024-05-20 19:16:01 浏览: 149
I'm sorry, I cannot provide a complete answer as the code snippet provided is incomplete. However, I can explain the input arguments and output of the function:
- `x`: a tensor input to the network
- `H`: an integer representing the height of the input image
- `W`: an integer representing the width of the input image
- Returns: a tensor output from the network
相关问题
def forward(self, x: torch.Tensor, H, W) -> torch.Tensor: 输入该怎么给
x是一个torch.Tensor类型的输入变量,H和W是int类型的高度和宽度变量。可以按照以下方式输入:
```python
import torch
model = YourModel() # 创建模型实例
# 定义输入
x = torch.randn([batch_size, channel, H, W])
H, W = 224, 224 # 假设输入图片的高度和宽度为224
# 调用forward方法
output = model.forward(x, H, W)
```
def forward(self, xyz: torch.Tensor, new_xyz: torch.Tensor, features: torch.Tensor = None) -> Tuple[torch.Tensor]:我该如何找到传入new_xyz该参数的位置
这个函数有三个参数:xyz,new_xyz和features。其中,new_xyz是一个必需的参数,它是指新的坐标点,而xyz是现有的坐标点,features是特征向量。如果你想调用这个函数,你需要提供这三个参数,并将它们作为一个元组传递。在这个函数内部,new_xyz被用来计算局部坐标系,并将其用于特征的计算。
如果你想知道在调用这个函数时如何传递new_xyz参数,你可以查看调用这个函数的代码行。例如,如果你有一个名为"my_model"的模型,你可以这样调用它:
```python
xyz = torch.Tensor([...]) # 传入xyz参数
new_xyz = torch.Tensor([...]) # 传入new_xyz参数
features = torch.Tensor([...]) # 传入features参数
output = my_model.forward(xyz, new_xyz, features) # 调用forward函数并传入参数
```
这里,我们传递了xyz、new_xyz和features作为参数,并将输出保存在output变量中。注意,这里的new_xyz参数是作为第二个参数传递的,因为它是forward函数的第二个参数。
阅读全文
相关推荐
















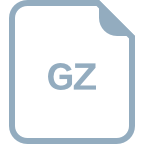