代码报错修改img,mask = next(iter(train_loader)) plt.subplot(121) plt.imshow((convertimg(img[2],3))) print(img.shape) plt.subplot(122) plt.imshow(convertimg(mask[2],1)) print(mask.shape) plt.show()
时间: 2023-10-06 21:10:58 浏览: 62
出现错误时,请先检查错误信息,错误信息通常会告诉您出现了什么问题。
如果是 DataLoader 对象没有数据导致的错误,可以检查 DataLoader 对象中的数据是否正确加载,或者确保训练数据集中有足够的数据。
如果是 convertimg 函数的错误,可以检查函数的实现是否正确,或者检查函数是否正确调用。
另外,建议您在代码中添加异常处理机制,以便更好地跟踪和调试错误。可以使用 try-except 语句来捕获异常并进行处理。例如:
```
try:
img, mask = next(iter(train_loader))
plt.subplot(121)
plt.imshow((convertimg(img[2],3)))
print(img.shape)
plt.subplot(122)
plt.imshow(convertimg(mask[2],1))
print(mask.shape)
plt.show()
except Exception as e:
print('Error:', e)
```
这样,在出现错误时,程序就会打印出错误信息,帮助您更好地调试代码。
相关问题
img,mask = next(iter(train_loader)) plt.subplot(121) plt.imshow((convertimg(img[2],3))) print(img.shape) plt.subplot(122) plt.imshow(convertimg(mask[2],1)) print(mask.shape) plt.show()
这段代码应该是用于可视化训练数据的,其中train_loader是训练数据集的DataLoader对象,通过调用next(iter(train_loader))可以得到一个batch的数据,其中img和mask分别表示图像和对应的掩膜。接下来的代码将第三张图像和掩膜可视化,并打印出了它们的形状。其中convertimg函数可能是一个自定义函数,用于将图像和掩膜转换为可视化的格式。
from sklearn.model_selection import train_test_split import sklearn.neural_network as net import matplotlib.cm as cm file_path=r'D:\anaconda3\temp\邮政编码数据.txt' data=pd.read_csv(file_path,header=0) print(data.shape) data.head() X=data.iloc[:,1:-1] Y=data.iloc[:,0] X.shape np.random.seed(1) ids=np.random.choice(len(Y),25) plt.figure(figsize=(8,8)) for i,item in enumerate(ids): img=np.array(X.iloc[item]).reshape(16,16) plt.subplot(5,5,i+1) plt.imshow(img,cmap=cm.gray_r) plt.show X_train,X_test,Y_train,Y_test = train_test_split(X,Y,train_size = 0.60,random_state = 123) nodes = np.arange(1,20,2) acts = ['relu','logistic'] errTrain = np.zeros((len(nodes),2)) errTest = np.zeros((len(nodes),2)) for i,node in enumerate(nodes): for j ,act in enumerate(acts): NeuNet = net.MLPClassifier(hidden_layer_sizes = (node,),activation = act,random_state = 1,max_iter=300) nodes=np.arange(1,20,2) acts=['relu','logistic'] NeuNet.fit(X_train,Y_train) errTrain[i,j] = 1-NeuNet.score(X_train,Y_train) errTest[i,j] = 1-NeuNet.score(X_test,Y_test) plt.plot(nodes,errTest[:,0],label='relu_test',linestyle='-') plt.plot(nodes,errTest[:,1],label='logistic_test',linestyle='-.') plt.plot(nodes,errTrain[:,0],label='relu_train',linestyle='-',linewidth=0.5) plt.plot(nodes,errTrain[:,1],label='logistic_train',linestyle='-',linewidth=0.5) plt.title('2012010812') plt.xlabel('hidden node numbers') plt.ylabel('erros') plt.xticks(nodes) plt.legend()
这段代码看起来有一些问题。首先,第一行 `from sklearn.model_selection import train_test_split import sklearn.neural_network as net import matplotlib.cm as cm` 应该换行,每个语句单独占一行。其次,在这个代码块中,没有导入 pandas 库,但是调用了 pandas 中的函数 `read_csv`。在代码块的后半部分,您定义了变量 `nodes`,但是在循环中又重新定义了 `nodes`,这会导致变量被覆盖,可能会影响到后续的程序运行。此外,循环中的绘图部分也存在一些问题,因为在循环中重复定义了绘图的参数。根据您的问题描述,我无法确定您的具体问题在哪里,但是这些问题可能会影响到程序的正确性。因此,您需要检查一下代码并修复这些问题,才能正常运行程序。
相关推荐
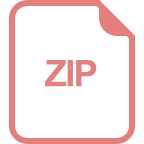
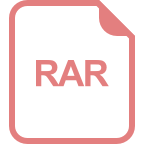












