# 导入相关库 import pandas as pd import matplotlib.pyplot as plt from sklearn.model_selection import train_test_split from sklearn.tree import DecisionTreeClassifier from sklearn.ensemble import RandomForestClassifier from sklearn.metrics import accuracy_score, precision_score, recall_score, f1_score,roc_auc_score,roc_curve # 读取数据 df = pd.read_csv('C:/Users/E15/Desktop/机器学习作业/第一次作业/第一次作业/三个数据集/Titanic泰坦尼克号.csv') # 数据预处理 df = df.drop(["Name", "Ticket", "Cabin"], axis=1) # 删除无用特征 df = pd.get_dummies(df, columns=["Sex", "Embarked"]) # 将分类特征转换成独热编码 df = df.fillna(df.mean()) # 使用平均值填充缺失值 # 划分数据集 X = df.drop(["Survived"], axis=1) y = df["Survived"] X_train, X_test, y_train, y_test = train_test_split(X, y, test_size=0.2, random_state=42) # 决策树 dtc = DecisionTreeClassifier(random_state=42) dtc.fit(X_train, y_train) y_pred_dtc = dtc.predict(X_test) # 剪枝决策树 pruned_dtc = DecisionTreeClassifier(random_state=42, ccp_alpha=0.015) pruned_dtc.fit(X_train, y_train) y_pred_pruned_dtc = pruned_dtc.predict(X_test) # 随机森林 rfc = RandomForestClassifier(n_estimators=100, random_state=42) rfc.fit(X_train, y_train) y_pred_rfc = rfc.predict(X_test) # 计算评价指标 metrics = {"Accuracy": accuracy_score, "Precision": precision_score, "Recall": recall_score, "F1-Score": f1_score, "AUC": roc_auc_score} results = {} for key in metrics.keys(): if key == "AUC": results[key] = {"Decision Tree": roc_auc_score(y_test, y_pred_dtc), "Pruned Decision Tree": roc_auc_score(y_test, y_pred_pruned_dtc), "Random Forest": roc_auc_score(y_test, y_pred_rfc)} else: results[key] = {"Decision Tree": metrics[key](y_test, y_pred_dtc), "Pruned Decision Tree": metrics[key](y_test, y_pred_pruned_dtc), "Random Forest": metrics[key](y_test, y_pred_rfc)} # 打印评价指标的表格 results_df = pd.DataFrame(results) print(results_df)怎么打印auv图
时间: 2024-04-21 18:27:02 浏览: 215
要打印AUC图,可以使用`roc_curve`函数获取ROC曲线的参数,然后使用`matplotlib`库绘制曲线。具体代码如下:
```
# 计算ROC曲线参数
fpr_dtc, tpr_dtc, thresholds_dtc = roc_curve(y_test, y_pred_dtc)
fpr_pruned_dtc, tpr_pruned_dtc, thresholds_pruned_dtc = roc_curve(y_test, y_pred_pruned_dtc)
fpr_rfc, tpr_rfc, thresholds_rfc = roc_curve(y_test, y_pred_rfc)
# 绘制ROC曲线
plt.figure(figsize=(8, 6))
plt.plot(fpr_dtc, tpr_dtc, label='Decision Tree')
plt.plot(fpr_pruned_dtc, tpr_pruned_dtc, label='Pruned Decision Tree')
plt.plot(fpr_rfc, tpr_rfc, label='Random Forest')
plt.plot([0, 1], [0, 1], linestyle='--', color='grey')
plt.xlabel('False Positive Rate')
plt.ylabel('True Positive Rate')
plt.title('Receiver Operating Characteristic (ROC) Curve')
plt.legend()
plt.show()
```
这段代码将会绘制一个ROC曲线,其中每个分类器都使用不同的颜色表示,同时包含一个虚线表示随机分类器的结果。注意,这段代码需要在之前的代码块中运行,以确保`fpr`,`tpr`和`y_pred`参数已经存在。
阅读全文
相关推荐
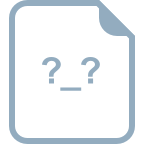
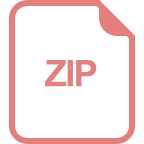
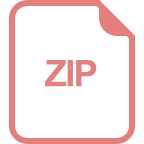















