class Pointnet2MSG(nn.Module): def __init__(self, input_channels=6, use_xyz=True): super().__init__() self.SA_modules = nn.ModuleList() channel_in = input_channels skip_channel_list = [input_channels] for k in range(cfg.RPN.SA_CONFIG.NPOINTS.len()): mlps = cfg.RPN.SA_CONFIG.MLPS[k].copy() channel_out = 0 for idx in range(mlps.len()): mlps[idx] = [channel_in] + mlps[idx] channel_out += mlps[idx][-1] mlps.append(channel_out) self.SA_modules.append( nn.Sequential( PointnetSAModuleMSG( npoint=cfg.RPN.SA_CONFIG.NPOINTS[k], radii=cfg.RPN.SA_CONFIG.RADIUS[k], nsamples=cfg.RPN.SA_CONFIG.NSAMPLE[k], mlps=mlps, use_xyz=use_xyz, bn=cfg.RPN.USE_BN ), SelfAttention(channel_out) ) ) skip_channel_list.append(channel_out) channel_in = channel_out你可以给我详细分析下这段代码每一句的含义和作用吗,越详细越好,我有点看不懂它
时间: 2023-11-27 15:50:13 浏览: 33
当然可以,我会尽可能详细地解释每一句代码的含义和作用。
```
class Pointnet2MSG(nn.Module):
def __init__(self, input_channels=6, use_xyz=True):
super().__init__()
self.SA_modules = nn.ModuleList()
channel_in = input_channels
skip_channel_list = [input_channels]
```
这是定义了一个名为`Pointnet2MSG`的类,它继承自`nn.Module`。在初始化函数`__init__`中,设定了`input_channels`输入通道数和`use_xyz`是否使用点云坐标作为输入的参数。`super().__init__()`表示调用父类的初始化函数。`self.SA_modules`是一个`nn.ModuleList()`,用于存储多个`PointnetSAModuleMSG`模块,`channel_in`是输入通道数,`skip_channel_list`是跳跃连接的通道列表。
```
for k in range(cfg.RPN.SA_CONFIG.NPOINTS.len()):
mlps = cfg.RPN.SA_CONFIG.MLPS[k].copy()
channel_out = 0
for idx in range(mlps.len()):
mlps[idx] = [channel_in] + mlps[idx]
channel_out += mlps[idx][-1]
mlps.append(channel_out)
self.SA_modules.append(
nn.Sequential(
PointnetSAModuleMSG(
npoint=cfg.RPN.SA_CONFIG.NPOINTS[k],
radii=cfg.RPN.SA_CONFIG.RADIUS[k],
nsamples=cfg.RPN.SA_CONFIG.NSAMPLE[k],
mlps=mlps,
use_xyz=use_xyz,
bn=cfg.RPN.USE_BN
),
SelfAttention(channel_out)
)
)
skip_channel_list.append(channel_out)
channel_in = channel_out
```
这是一个循环,用于遍历`cfg.RPN.SA_CONFIG.NPOINTS`中的每个元素,并对每个元素都构建一个`PointnetSAModuleMSG`模块。其中,`mlps`是多层感知器(MLP)列表,每个MLP的输入通道数为`channel_in`,输出通道数为`mlps[idx][-1]`,`channel_out`表示当前模块的输出通道数。循环中,将每个MLP的输入通道数改为`channel_in+mlps[idx][0]`,并将所有MLP的输出通道数累加到`channel_out`中。最后,将总输出通道数`channel_out`添加到`mlps`的末尾,构建`PointnetSAModuleMSG`模块,并将该模块和一个`SelfAttention`层放在一起构成一个`nn.Sequential`模块,最后将该模块添加到`self.SA_modules`列表中,并将`channel_out`添加到`skip_channel_list`中。最后一行代码将`channel_out`赋值给`channel_in`,以便下一次循环使用。
总的来说,这段代码的作用是构建了一个带有多层感知器(MLP)和自注意力机制的点云分割网络,其中`PointnetSAModuleMSG`模块用于提取特征,`SelfAttention`层用于增强特征的表达能力,`nn.Sequential`用于将两个模块串联起来。
相关推荐
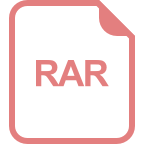
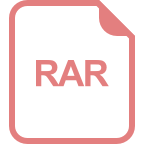











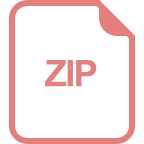
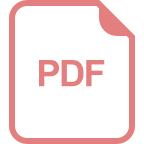
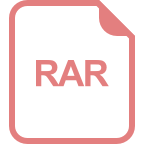
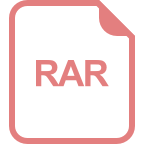
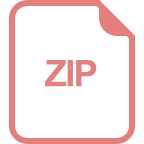