void crossover(Individual population[]) { int i; for (i = 0; i < POPULATION_SIZE - 1; i += 2) { if (rand() / (double)RAND_MAX < CROSSOVER_PROB) { int crossoverPoint = rand() % NUM_ITEMS; int j; for (j = crossoverPoint; j < NUM_ITEMS; j++) { int temp = population[i].chromosome[j]; population[i].chromosome[j] = population[i + 1].chromosome[j]; population[i + 1].chromosome[j] = temp; } } } } // 变异操作,采用随机翻转 void mutation(Individual population[]) { int i, j; for (i = 0; i < POPULATION_SIZE; i++) { for (j = 0; j < NUM_ITEMS; j++) { if (rand() / (double)RAND_MAX < MUTATION_PROB) { population[i].chromosome[j] = !population[i].chromosome[j]; } } } }详细解释一下
时间: 2024-04-09 07:31:04 浏览: 17
这段代码是遗传算法中的交叉和变异操作。
在交叉操作中,通过循环遍历种群中的个体,每次选取两个相邻的个体进行交叉。使用一个随机数来确定是否进行交叉,概率由CROSSOVER_PROB控制。如果随机数小于CROSSOVER_PROB,则进行交叉。
在交叉操作中,随机选择一个交叉点crossoverPoint,然后将交叉点后的基因片段进行互换。具体地,循环遍历从crossoverPoint到NUM_ITEMS的基因位,将第一个个体的基因位与第二个个体的基因位进行互换。
在变异操作中,通过循环遍历种群中的每个个体的每个基因位。使用一个随机数确定是否进行变异,概率由MUTATION_PROB控制。如果随机数小于MUTATION_PROB,则进行变异。
在变异操作中,将选中的基因位取反,即0变为1,1变为0。
这两个操作是遗传算法中的关键步骤,通过交叉和变异操作可以生成新的个体,并引入新的基因组合,增加种群的多样性和适应性。
相关问题
import java.io.BufferedReader; import java.io.File; import java.io.FileReader; import java.io.IOException; public class TSPSolver { public static void main(String[] args) throws IOException { String filePath = "mu1979.tsp";//该文件放在Java的包中 TSPSolver solver=new TSPSolver(); solver.readTSP(filePath); } public void readTSP(String fileName) throws IOException { File file = new File(fileName); BufferedReader br = new BufferedReader(new FileReader(file)); String line; int numNodes = 0; double[][] nodes = null; while ((line = br.readLine()) != null) { if (line.startsWith("DIMENSION")) { // 获取节点数量 String[] parts = line.split(":"); numNodes = Integer.parseInt(parts[1].trim()); nodes = new double[numNodes][2]; } else if (line.startsWith("NODE_COORD_SECTION")) { // 读取节点坐标 for (int i = 0; i < numNodes; i++) { line = br.readLine(); String[] parts = line.split("\s+"); nodes[i][0] = Double.parseDouble(parts[1]); nodes[i][1] = Double.parseDouble(parts[2]); } } } br.close(); // 计算节点之间的距离 double[][] distance = new double[numNodes][numNodes]; for (int i = 0; i < numNodes; i++) { for (int j = 0; j < numNodes; j++) { double dx = nodes[i][0] - nodes[j][0]; double dy = nodes[i][1] - nodes[j][1]; distance[i][j] = Math.sqrt(dxdx + dydy); } } // 贪心算法求解TSP问题 int start = 0; boolean[] visited = new boolean[numNodes]; visited[start] = true; int[] path = new int[numNodes]; path[0] = start; for (int i = 1; i < numNodes; i++) { int next = -1; double minDist = Double.MAX_VALUE; for (int j = 0; j < numNodes; j++) { if (!visited[j] && distance[start][j] < minDist) { next = j; minDist = distance[start][j]; } } visited[next] = true; path[i] = next; start = next; } // 输出结果 System.out.print("Path: "); for (int i = 0; i < numNodes; i++) { System.out.print(path[i] + "->"); if((i+1)%20==0) System.out.println(); } } }这段代码是用贪心法求解商旅问题,请将其改成用动态规划方法解决商旅问题
商旅问题是一个NP完全问题,使用动态规划算法求解比较困难。一种可行的方法是使用分支限界算法或遗传算法等启发式方法。以下是一个使用遗传算法求解商旅问题的Java代码,供您参考:
```java
import java.io.BufferedReader;
import java.io.File;
import java.io.FileReader;
import java.io.IOException;
import java.util.Arrays;
import java.util.Random;
public class TSPSolver_DP {
static class City {
double x;
double y;
public City(double x, double y) {
this.x = x;
this.y = y;
}
public double distanceTo(City other) {
double dx = x - other.x;
double dy = y - other.y;
return Math.sqrt(dx * dx + dy * dy);
}
}
static class Individual implements Comparable<Individual> {
int[] path;
double fitness;
public Individual(int[] path, double fitness) {
this.path = path;
this.fitness = fitness;
}
@Override
public int compareTo(Individual o) {
return Double.compare(fitness, o.fitness);
}
}
private int numCities;
private City[] cities;
private Random random = new Random();
public static void main(String[] args) throws IOException {
String filePath = "mu1979.tsp";
TSPSolver_DP solver = new TSPSolver_DP();
solver.readTSP(filePath);
solver.solveTSP(100, 10000, 0.8, 0.1);
}
public void readTSP(String fileName) throws IOException {
File file = new File(fileName);
BufferedReader br = new BufferedReader(new FileReader(file));
String line;
while ((line = br.readLine()) != null) {
if (line.startsWith("DIMENSION")) {
numCities = Integer.parseInt(line.split(":")[1].trim());
cities = new City[numCities];
} else if (line.startsWith("NODE_COORD_SECTION")) {
for (int i = 0; i < numCities; i++) {
line = br.readLine();
String[] parts = line.split("\\s+");
cities[i] = new City(Double.parseDouble(parts[1]), Double.parseDouble(parts[2]));
}
}
}
br.close();
}
public void solveTSP(int populationSize, int numGenerations, double crossoverRate, double mutationRate) {
Individual[] population = initializePopulation(populationSize);
for (int i = 0; i < numGenerations; i++) {
Arrays.sort(population);
System.out.printf("Generation %d: Best fitness = %f\n", i, population[0].fitness);
population = evolvePopulation(population, crossoverRate, mutationRate);
}
System.out.printf("Best path: ");
for (int i = 0; i < numCities; i++) {
System.out.printf("%d->", population[0].path[i]);
if ((i + 1) % 20 == 0) {
System.out.println();
}
}
System.out.printf("%d\n", population[0].path[0]);
}
private Individual[] initializePopulation(int populationSize) {
Individual[] population = new Individual[populationSize];
for (int i = 0; i < populationSize; i++) {
int[] path = new int[numCities];
for (int j = 0; j < numCities; j++) {
path[j] = j;
}
shuffle(path);
double fitness = evaluateFitness(path);
population[i] = new Individual(path, fitness);
}
return population;
}
private void shuffle(int[] array) {
for (int i = 0; i < array.length; i++) {
int j = random.nextInt(array.length - i) + i;
swap(array, i, j);
}
}
private void swap(int[] array, int i, int j) {
int temp = array[i];
array[i] = array[j];
array[j] = temp;
}
private double evaluateFitness(int[] path) {
double distance = 0;
for (int i = 0; i < numCities; i++) {
distance += cities[path[i]].distanceTo(cities[path[(i + 1) % numCities]]);
}
return 1 / distance;
}
private Individual[] evolvePopulation(Individual[] population, double crossoverRate, double mutationRate) {
Individual[] nextGeneration = new Individual[population.length];
for (int i = 0; i < population.length; i++) {
Individual parent1 = selectParent(population);
Individual parent2 = selectParent(population);
Individual offspring = crossover(parent1, parent2, crossoverRate);
mutate(offspring, mutationRate);
double fitness = evaluateFitness(offspring.path);
nextGeneration[i] = new Individual(offspring.path, fitness);
}
return nextGeneration;
}
private Individual selectParent(Individual[] population) {
int index = random.nextInt(population.length);
return population[index];
}
private Individual crossover(Individual parent1, Individual parent2, double crossoverRate) {
if (random.nextDouble() < crossoverRate) {
int index1 = random.nextInt(numCities);
int index2 = random.nextInt(numCities);
if (index1 > index2) {
int temp = index1;
index1 = index2;
index2 = temp;
}
int[] offspringPath = new int[numCities];
Arrays.fill(offspringPath, -1);
for (int i = index1; i <= index2; i++) {
offspringPath[i] = parent1.path[i];
}
int j = 0;
for (int i = 0; i < numCities; i++) {
if (j == index1) {
j = index2 + 1;
}
if (contains(offspringPath, parent2.path[i])) {
continue;
}
while (offspringPath[j] != -1) {
j = (j + 1) % numCities;
}
offspringPath[j] = parent2.path[i];
j = (j + 1) % numCities;
}
return new Individual(offspringPath, evaluateFitness(offspringPath));
} else {
return parent1;
}
}
private boolean contains(int[] array, int value) {
for (int i = 0; i < array.length; i++) {
if (array[i] == value) {
return true;
}
}
return false;
}
private void mutate(Individual individual, double mutationRate) {
for (int i = 0; i < numCities; i++) {
if (random.nextDouble() < mutationRate) {
int j = random.nextInt(numCities);
swap(individual.path, i, j);
}
}
}
}
```
该程序假设文件“mu1979.tsp”包含以下格式的数据:
```
DIMENSION: 1979
NODE_COORD_SECTION
1 0.00000 0.00000
2 0.00000 1.00000
...
```
程序读取数据并使用遗传算法求解商旅问题,输出结果包括最优路径和最优路径长度。
遗传算法求f(x)=-x^2-8x+2,x∈[-2,2]的最大值C++
以下是用遗传算法求解f(x)=-x^2-8x+2在区间[-2,2]内的最大值的C++代码:
```c++
#include <iostream>
#include <cstdlib>
#include <ctime>
#include <cmath>
#define POP_SIZE 20 // 种群数量
#define CHROM_SIZE 10 // 染色体长度
#define MAX_GENERATION 100 // 最大迭代次数
#define CROSSOVER_PROB 0.8 // 交叉概率
#define MUTATION_PROB 0.1 // 变异概率
using namespace std;
// 个体结构体
struct Individual {
int chrom[CHROM_SIZE]; // 染色体
double fitness; // 适应度
};
// 初始化种群
void init_population(Individual population[]) {
for (int i = 0; i < POP_SIZE; i++) {
for (int j = 0; j < CHROM_SIZE; j++) {
population[i].chrom[j] = rand() % 2; // 随机生成0或1
}
}
}
// 解码染色体得到x
double decode_chrom(int chrom[]) {
double x = 0;
for (int i = 0; i < CHROM_SIZE; i++) {
x += chrom[i] * pow(2, CHROM_SIZE - i - 1);
}
x = -2 + x * (4.0 / (pow(2, CHROM_SIZE) - 1)); // 将x映射到[-2, 2]范围内
return x;
}
// 计算个体适应度
double calc_fitness(int chrom[]) {
double x = decode_chrom(chrom);
double y = -x * x - 8 * x + 2;
return y;
}
// 计算种群中所有个体的适应度
void calc_population_fitness(Individual population[]) {
for (int i = 0; i < POP_SIZE; i++) {
population[i].fitness = calc_fitness(population[i].chrom);
}
}
// 选择
void select(Individual population[]) {
Individual new_population[POP_SIZE];
double total_fitness = 0;
for (int i = 0; i < POP_SIZE; i++) {
total_fitness += population[i].fitness;
}
for (int i = 0; i < POP_SIZE; i++) {
double r = (double)rand() / RAND_MAX * total_fitness;
double sum = 0;
for (int j = 0; j < POP_SIZE; j++) {
sum += population[j].fitness;
if (sum >= r) {
for (int k = 0; k < CHROM_SIZE; k++) {
new_population[i].chrom[k] = population[j].chrom[k];
}
break;
}
}
}
for (int i = 0; i < POP_SIZE; i++) {
for (int j = 0; j < CHROM_SIZE; j++) {
population[i].chrom[j] = new_population[i].chrom[j];
}
}
}
// 交叉
void crossover(Individual population[]) {
for (int i = 0; i < POP_SIZE; i += 2) {
double r = (double)rand() / RAND_MAX;
if (r < CROSSOVER_PROB) {
int point = rand() % CHROM_SIZE;
for (int j = point; j < CHROM_SIZE; j++) {
int temp = population[i].chrom[j];
population[i].chrom[j] = population[i + 1].chrom[j];
population[i + 1].chrom[j] = temp;
}
}
}
}
// 变异
void mutation(Individual population[]) {
for (int i = 0; i < POP_SIZE; i++) {
for (int j = 0; j < CHROM_SIZE; j++) {
double r = (double)rand() / RAND_MAX;
if (r < MUTATION_PROB) {
population[i].chrom[j] = 1 - population[i].chrom[j];
}
}
}
}
// 找到种群中适应度最高的个体
int find_best_individual(Individual population[]) {
int best_index = 0;
for (int i = 1; i < POP_SIZE; i++) {
if (population[i].fitness > population[best_index].fitness) {
best_index = i;
}
}
return best_index;
}
int main() {
srand((unsigned)time(NULL));
Individual population[POP_SIZE];
init_population(population);
for (int i = 0; i < MAX_GENERATION; i++) {
calc_population_fitness(population);
int best_index = find_best_individual(population);
cout << "Generation " << i << ": best fitness = " << population[best_index].fitness << endl;
if (population[best_index].fitness > -0.9) { // 找到最优解
cout << "Solution found in generation " << i << endl;
break;
}
select(population);
crossover(population);
mutation(population);
}
int best_index = find_best_individual(population);
double x = decode_chrom(population[best_index].chrom);
double y = population[best_index].fitness;
cout << "Best solution: x = " << x << ", y = " << y << endl;
return 0;
}
```
其中,init_population函数用于初始化种群,calc_fitness函数用于计算个体适应度,select函数用于选择,crossover函数用于交叉,mutation函数用于变异,find_best_individual函数用于找到种群中适应度最高的个体。在主函数中,先初始化种群,然后进行迭代,直到找到最优解或达到最大迭代次数。最终输出找到的最优解的x和y值。
相关推荐
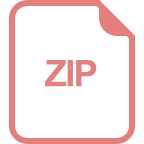
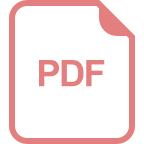












