newdf['date'] = newdf.index newdf['hour'] = newdf['date'].dt.hour newdf = encode(newdf, 'hour', 24) newdf['dayofweek'] = newdf['date'].dt.dayofweek newdf = encode(newdf, 'dayofweek', 7) newdf['month'] = newdf['date'].dt.month newdf = encode(newdf, 'month', 12)
时间: 2023-10-11 21:10:43 浏览: 37
这段代码是对时间数据进行编码的一系列操作。首先,将数据集的索引列转化为一个名为 date 的新列。然后,从 date 列中提取出小时信息,并使用 encode 函数对其进行编码,得到名为 hour_sin 和 hour_cos 的新列。接着,从 date 列中提取出星期几的信息,并使用 encode 函数对其进行编码,得到名为 dayofweek_sin 和 dayofweek_cos 的新列。最后,从 date 列中提取出月份信息,并使用 encode 函数对其进行编码,得到名为 month_sin 和 month_cos 的新列。这些操作可以将时间数据转化为一组连续的数值型特征,方便模型进行处理。
相关问题
newdf1= df1.groupby(['安检通道','安检时刻'],as_index=False)['排队时间'].agg('mean')#取平均
这行代码的意思是,以“安检通道”和“安检时刻”这两列为分类条件,对“排队时间”这一列进行分组,并计算均值。生成一个新的数据框“newdf1”。同时,参数“as_index=False”表示不要将分组后的列设置为索引。
while True: x=x+7 pyautogui.moveTo(x,y) img1 = pyautogui.screenshot(region=(2279 - 24,237- 66, 214, 154)) img2 = pyautogui.screenshot(region=(117 - 24, 238 - 66, 214, 154)) pool.submit(gsmpiodbspn_thread, newdf, img1,img2) if (("15:00" in newdf) == True or x >= 2475): break。上面这段个代码,在不改变原来功能的情况下,如何提高运行速度。
可以考虑使用多线程或协程来加速图像截屏和处理的过程。具体方法如下:
1. 使用`concurrent.futures`或`multiprocessing`模块创建线程池或进程池。
2. 将截屏和处理图像的代码放在一个函数中,作为线程或进程的任务。
3. 将任务提交到线程池或进程池中,使用`submit()`方法异步执行,并将结果存储在列表中。
4. 在主线程中等待所有任务完成,并将结果合并为一个字典。
这样可以将图像截屏和处理的过程并行化,提高程序的运行速度。具体实现如下:
```python
import concurrent.futures
def get_minute_price(pool, newdf, x, y):
img1 = pyautogui.screenshot(region=(2279 - 24,237- 66, 214, 154))
img2 = pyautogui.screenshot(region=(117 - 24, 238 - 66, 214, 154))
pool.submit(gsmpiodbspn_thread, newdf, img1,img2)
with concurrent.futures.ThreadPoolExecutor() as pool:
while True:
x += 7
pyautogui.moveTo(x, y)
get_minute_price(pool, newdf, x, y)
if "15:00" in newdf or x >= 2475:
break
```
此外,还可以考虑使用 `pyautogui.locateAllOnScreen()` 方法替换 `pyautogui.locateOnScreen()` 方法,以避免多次查找同一个位置的图像。
相关推荐
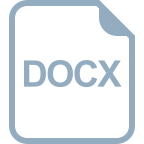
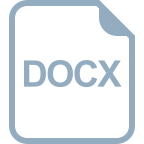
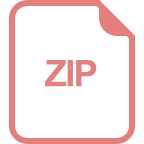












