class Conv3x3BnLeakyRelu(keras.Model): """
时间: 2023-10-30 22:07:35 浏览: 83
这是一个继承自Keras Model的自定义模型类,表示一个包含卷积层、Batch Normalization层和Leaky ReLU激活函数的神经网络模型。具体来说,该模型包含一个3x3的卷积层,其输出被送入Batch Normalization层进行归一化处理,然后再通过Leaky ReLU激活函数进行非线性转换。
相关问题
class Conv3D(keras.Model):
The `Conv3D` class is a subclass of `keras.Model` in the Keras deep learning library. It is used for creating 3D convolutional neural networks (CNNs) for processing volumetric data such as videos or 3D images.
The `Conv3D` class allows you to define a 3D convolutional layer with a specified number of filters, kernel size, strides, padding, and activation function. You can also add additional layers such as pooling, dropout, and batch normalization to the network.
Here's an example of how to define a simple 3D CNN using the `Conv3D` class:
```
from keras.layers import Input, Conv3D, MaxPooling3D, Flatten, Dense
from keras.models import Model
# Define input shape
input_shape = (32, 32, 32, 1)
# Define input layer
inputs = Input(shape=input_shape)
# Define convolutional layers
conv1 = Conv3D(filters=32, kernel_size=(3, 3, 3), activation='relu')(inputs)
pool1 = MaxPooling3D(pool_size=(2, 2, 2))(conv1)
conv2 = Conv3D(filters=64, kernel_size=(3, 3, 3), activation='relu')(pool1)
pool2 = MaxPooling3D(pool_size=(2, 2, 2))(conv2)
# Define fully connected layers
flatten = Flatten()(pool2)
fc1 = Dense(units=128, activation='relu')(flatten)
outputs = Dense(units=10, activation='softmax')(fc1)
# Define model
model = Model(inputs=inputs, outputs=outputs)
```
In this example, we define a 3D CNN with two convolutional layers, two max pooling layers, and two fully connected layers for classification. The `Conv3D` class is used to define the convolutional layers with specified number of filters (32 and 64), kernel size (3x3x3), and activation function (ReLU). The `MaxPooling3D` class is used to define the pooling layers with a pool size of 2x2x2. Finally, the `Dense` class is used to define the fully connected layers with specified number of units (128 and 10 for output) and activation function (ReLU and softmax for output).
如何导入包含conv3x3函数的库,如tf.keras.layers.Conv2D或torch.nn.Conv2d
在Python中,为了导入包含`conv3x3`函数的库(如TensorFlow的`tf.keras.layers.Conv2D`或PyTorch的`torch.nn.Conv2d`),你需要按照各自的库的导入方式来做:
**对于TensorFlow(Keras API):**
```python
import tensorflow as tf
from tensorflow.keras.layers import Conv2D
# 然后你可以直接使用Conv2D函数
conv_layer = Conv2D(filters=32, kernel_size=(3, 3), activation='relu') # 假设你想创建一个3x3卷积层
```
**对于PyTorch:**
```python
import torch
import torch.nn as nn
# 使用nn.Conv2d
class ConvBlock(nn.Module):
def __init__(self):
super(ConvBlock, self).__init__()
self.conv = nn.Conv2d(in_channels=3, out_channels=32, kernel_size=3, stride=1, padding=1) # 3x3卷积层
# 创建模块实例
model = ConvBlock()
```
在上述代码中,首先导入了所需的库,然后通过库提供的模块(如`tf.keras.layers`或`torch.nn`)找到了`Conv2D`或`Conv2d`函数。这样,在代码中就可以使用它们创建卷积层了。
阅读全文
相关推荐
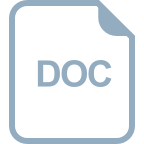
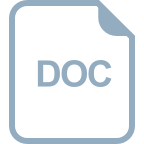
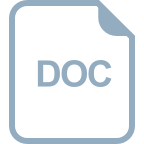


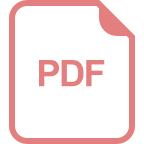
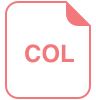
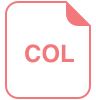
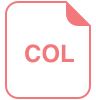
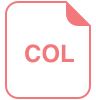
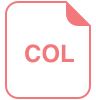
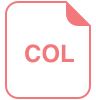
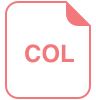
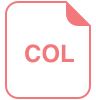
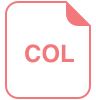
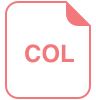
